Machine Learning and the Data Science Process
OxWaSP, Berlin
ARM Data Science Conference
2017-03-30
Neil D. Lawrence
Amazon and University of Sheffield
@lawrennd
inverseprobability.com
Machine Learning
\[ \text{data} + \text{model} \xrightarrow{\text{compute}} \text{prediction}\]
Machine Learning
Normal ML (& stats?) focus: model
In real world need more focus on: data
motivation for data science
Background: Big Data
The pervasiveness of data brings forward particular challenges.
Emerging themes: Devolving compute onto device.
Data preprocessing: Internet of Intelligence.
Effects
This phenomenon has already revolutionised biology.
Large scale data acquisition and distribution.
What does it mean for IoT
Challenges
Paradoxes of the Data Society
Quantifying the Value of Data
Privacy, loss of control, marginalisation
Deploying AI in the real world.
Challenges
Paradoxes of the Data Society
Quantifying the Value of Data
Privacy, loss of control, marginalisation
Deploying AI in the real world.
Quantifying the Value of Data
There’s a sea of data, but most of it is undrinkable
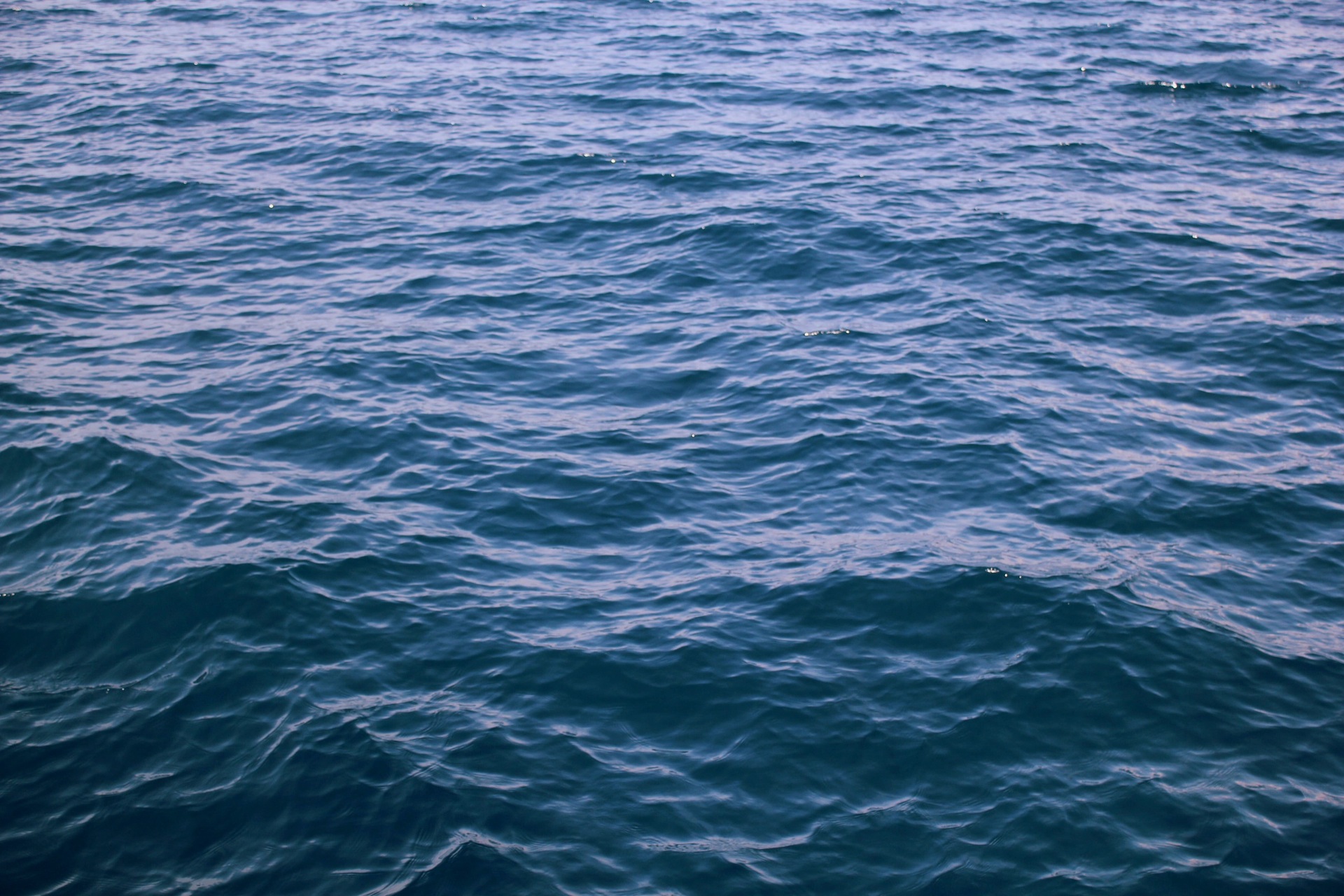
We require data-desalination before it can be consumed!
Data — Quotes from NIPS Workshop on ML for Healthcare
- 90% of our time is spent on validation and integration (Leo Anthony Celi)
- “The Dirty Work We Don’t Want to Think About” (Eric Xing)
- “Voodoo to get it decompressed” (Francisco Giminez)
- In health care clinicians collect the data and often control the direction of research through guardianship of data.
Value
- How do we measure value in the data economy?
- How do we encourage data workers: curation and management
- Incentivization for sharing and production.
- Quantifying the value in the contribution of each actor.
Three Grades of Data Readiness
- Grade C - accessibility
- Transition: data becomes electronically available
- Grade B - validity
- Transition: pose a question to the data.
- Grade A - usability
Accessibility: Grade C
- Hearsay data.
- Availability, is it actually being recorded?
- privacy or legal constraints on the accessibility of the recorded data, have ethical constraints been alleviated?
- Format: log books, PDF …
- limitations on access due to topology (e.g. it’s distributed across a number of devices)
- At the end of Grade C data is ready to be loaded into analysis software (R, SPSS, Matlab, Python, Mathematica)
Validity: Grade B
- faithfulness and representation
- visualisations.
- exploratory data analysis
- noise characterisation.
Grade B Checks
- Missing values.
- Schema alignment, record linkage, data fusion
- Example:
Grade B Transition
- At the end of Grade B, ready to define a task, or question
- Compare with classical statistics:
- Classically: question is first data comes later.
- Today: data is first question comes later.
Data First
In a data first company teams own their data quality issues at least as far as grade B1.
Usability: Grade A
- The usability of data
- Grade A is about data in context.
- Consider appropriateness of a given data set to answer a particular question or to be subject to a particular analysis.
Recursive Effects
- Grade A may also require:
- data integration
- active collection of new data.
- rebalancing of data to ensure fairness
- annotation of data by human experts
- revisiting the collection (and running through the appropriate stages again)
A1 Data
- A1 data is ready to make available for challenges or AutoML platforms.
Also …
- Encourage greater interaction between application domains and data scientists
- Encourage visualization of data
Machine Learning Systems Design
Fragility of AI Systems
- They are componentwise built from ML Capabilities.
- Each capability is independently constructed and verified.
- Pedestrian detection
- Road line detection
- Important for verification purposes.
Rapid Reimplementation
- Whole systems are being deployed.
- But they change their environment.
- The experience evolved adversarial behaviour.
Turnaround And Update
- There is a massive need for turn around and update
- A redeploy of the entire system.
- This involves changing the way we design and deploy.
- Early Example: Stuxnet.
Peppercorns
- A new name for system failures which aren’t bugs.
- Difference between finding a fly in your soup vs a peppercorn in your soup.
Conclusion
- Data science offers a great deal of promise for personalized health
- There are challenges and pitfalls
- It is incumbent on us to avoid them
Many solutions rely on education and awareness
- There are particular challenges around the Internet of Intelligence.
Thanks!
Lawrence, N.D., 2017a. Living together: Mind and machine intelligence. arXiv.
Lawrence, N.D., 2017b. Data readiness levels. arXiv.