AI needs to serve people, science, and society
Abstract
As artificial intelligence becomes ubiquitous in our homes and workplaces, we need to develop a widespread understanding of what it is and how we use it in the interests of our societies. Neil will discuss how the artificial systems we have developed operate in a fundamentally different way to our own intelligence and how this difference in operational capability leads us to misunderstand the influence that decisions made by machine intelligence are having on our lives. Without this understanding we cannot take back control of those decisions from the machine. This will set the scene for approaches we are taking in Cambridge to address these challenges such as AI@Cam, the University’s flagship mission on AI.
Henry Ford’s Faster Horse
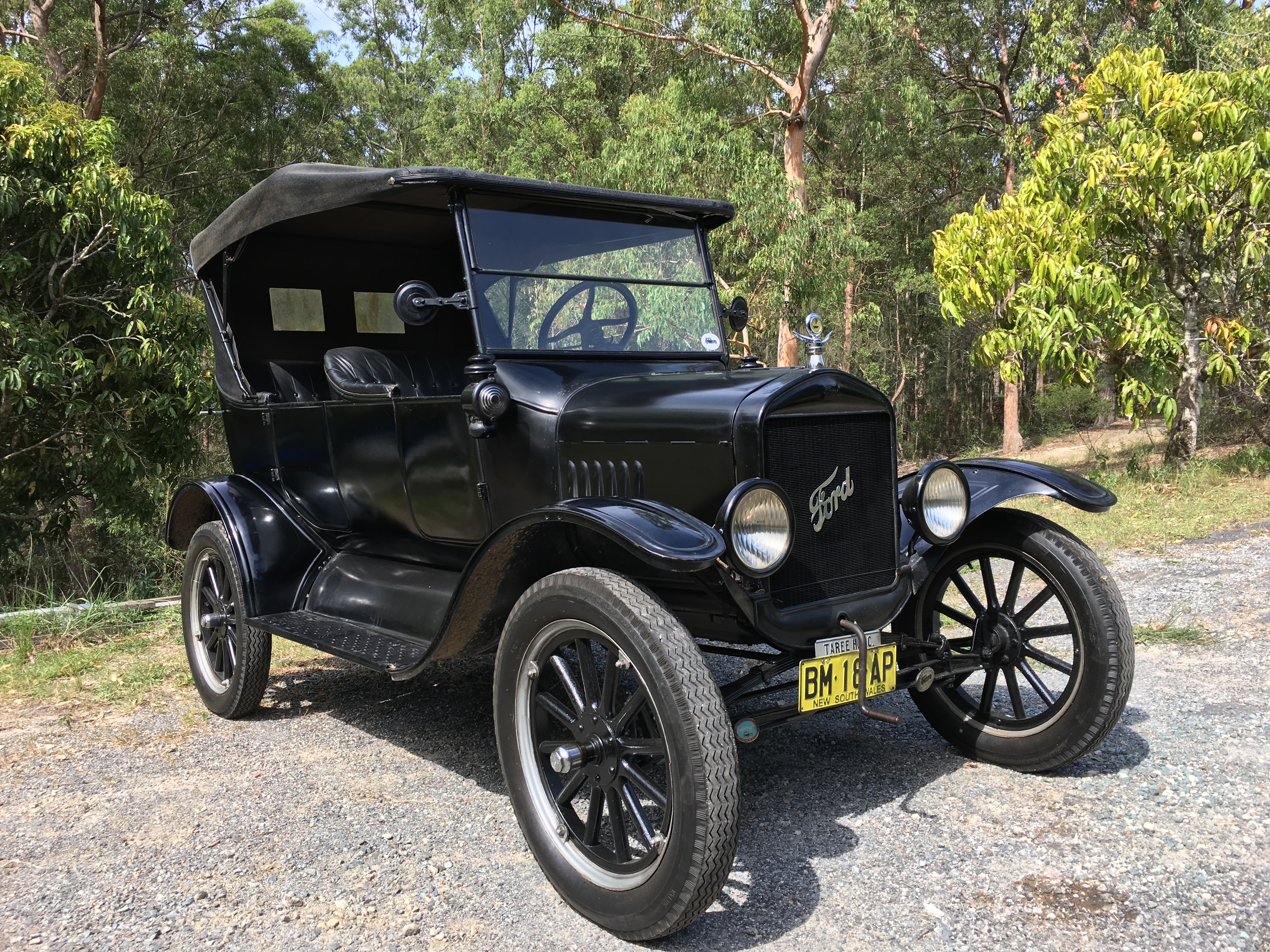
Figure: A 1925 Ford Model T built at Henry Ford’s Highland Park Plant in Dearborn, Michigan. This example now resides in Australia, owned by the founder of FordModelT.net. From https://commons.wikimedia.org/wiki/File:1925_Ford_Model_T_touring.jpg
It’s said that Henry Ford’s customers wanted a “a faster horse”. If Henry Ford was selling us artificial intelligence today, what would the customer call for, “a smarter human”? That’s certainly the picture of machine intelligence we find in science fiction narratives, but the reality of what we’ve developed is much more mundane.
Car engines produce prodigious power from petrol. Machine intelligences deliver decisions derived from data. In both cases the scale of consumption enables a speed of operation that is far beyond the capabilities of their natural counterparts. Unfettered energy consumption has consequences in the form of climate change. Does unbridled data consumption also have consequences for us?
If we devolve decision making to machines, we depend on those machines to accommodate our needs. If we don’t understand how those machines operate, we lose control over our destiny. Our mistake has been to see machine intelligence as a reflection of our intelligence. We cannot understand the smarter human without understanding the human. To understand the machine, we need to better understand ourselves.
The Great AI Fallacy
There is a lot of variation in the use of the term artificial intelligence. I’m sometimes asked to define it, but depending on whether you’re speaking to a member of the public, a fellow machine learning researcher, or someone from the business community, the sense of the term differs.
However, underlying its use I’ve detected one disturbing trend. A trend I’m beginining to think of as “The Great AI Fallacy”.
The fallacy is associated with an implicit promise that is embedded in many statements about Artificial Intelligence. Artificial Intelligence, as it currently exists, is merely a form of automated decision making. The implicit promise of Artificial Intelligence is that it will be the first wave of automation where the machine adapts to the human, rather than the human adapting to the machine.
How else can we explain the suspension of sensible business judgment that is accompanying the hype surrounding AI?
This fallacy is particularly pernicious because there are serious benefits to society in deploying this new wave of data-driven automated decision making. But the AI Fallacy is causing us to suspend our calibrated skepticism that is needed to deploy these systems safely and efficiently.
The problem is compounded because many of the techniques that we’re speaking of were originally developed in academic laboratories in isolation from real-world deployment.
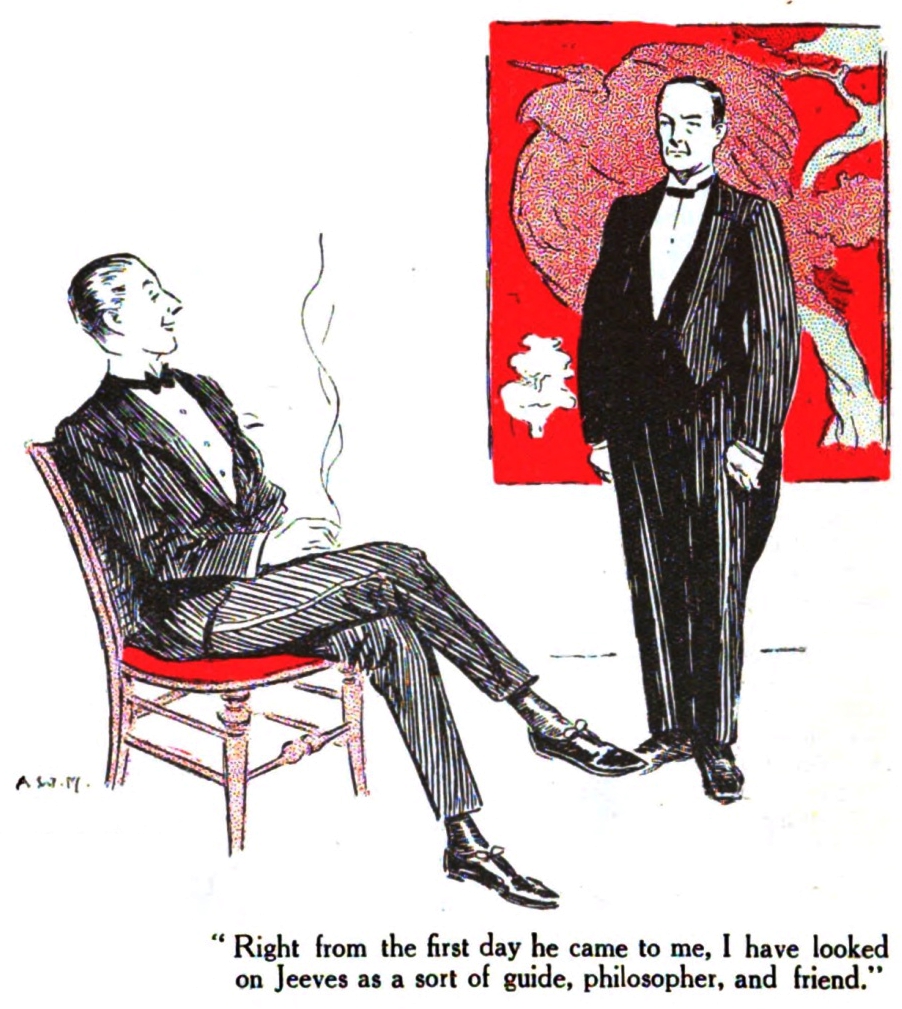
Figure: We seem to have fallen for a perspective on AI that suggests it will adapt to our schedule, rather in the manner of a 1930s manservant.
In Greek mythology, Panacea was the goddess of the universal remedy. One consequence of the pervasive potential of AI is that it is positioned, like Panacea, as the purveyor of a universal solution. Whether it is overcoming industry’s productivity challenges, or as a salve for strained public sector services, or a remedy for pressing global challenges in sustainable development, AI is presented as an elixir to resolve society’s problems.
In practice, translation of AI technology into practical benefit is not simple. Moreover, a growing body of evidence shows that risks and benefits from AI innovations are unevenly distributed across society.
When carelessly deployed, AI risks exacerbating existing social and economic inequalities.
The Diving Bell and the Butterfly
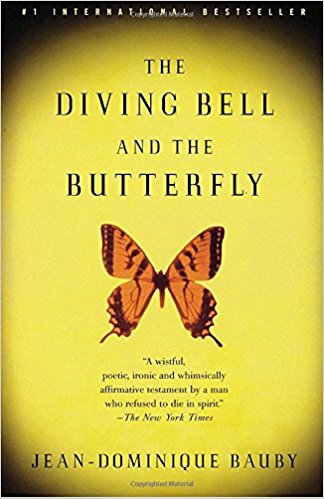
Figure: The Diving Bell and the Buttefly is the autobiography of Jean Dominique Bauby.
The Diving Bell and the Butterfly is the autobiography of Jean Dominique Bauby. Jean Dominique was the editor of the French Elle magazine, in 1995 at the age of 43, he suffered a major stroke. The stroke paralyzed him and rendered him speechless. He was only able to blink his left eyelid, he became a sufferer of locked in syndrome.
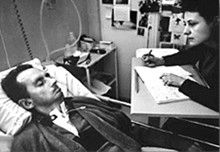
Figure: Jean Dominique Bauby was the Editor in Chief of the French Elle Magazine, he suffered a stroke that destroyed his brainstem, leaving him only capable of moving one eye. Jean Dominique became a victim of locked in syndrome.
Incredibly, Jean Dominique wrote his book after he became locked in. It took him 10 months of four hours a day to write the book. Each word took two minutes to write.
The idea behind embodiment factors is that we are all in that situation. While not as extreme as for Bauby, we all have somewhat of a locked in intelligence.
![]() |
![]() |
Figure: Claude Shannon developed information theory which allows us to quantify how much Bauby can communicate. This allows us to compare how locked in he is to us.
Embodiment Factors
bits/min | billions | 2,000 |
billion calculations/s |
~100 | a billion |
embodiment | 20 minutes | 5 billion years |
Figure: Embodiment factors are the ratio between our ability to compute and our ability to communicate. Relative to the machine we are also locked in. In the table we represent embodiment as the length of time it would take to communicate one second’s worth of computation. For computers it is a matter of minutes, but for a human, it is a matter of thousands of millions of years. See also “Living Together: Mind and Machine Intelligence” Lawrence (2017)
There is a fundamental limit placed on our intelligence based on our ability to communicate. Claude Shannon founded the field of information theory. The clever part of this theory is it allows us to separate our measurement of information from what the information pertains to.1
Shannon measured information in bits. One bit of information is the amount of information I pass to you when I give you the result of a coin toss. Shannon was also interested in the amount of information in the English language. He estimated that on average a word in the English language contains 12 bits of information.
Given typical speaking rates, that gives us an estimate of our ability to communicate of around 100 bits per second (Reed and Durlach, 1998). Computers on the other hand can communicate much more rapidly. Current wired network speeds are around a billion bits per second, ten million times faster.
When it comes to compute though, our best estimates indicate our computers are slower. A typical modern computer can process make around 100 billion floating-point operations per second, each floating-point operation involves a 64 bit number. So the computer is processing around 6,400 billion bits per second.
It’s difficult to get similar estimates for humans, but by some estimates the amount of compute we would require to simulate a human brain is equivalent to that in the UK’s fastest computer (Ananthanarayanan et al., 2009), the MET office machine in Exeter, which in 2018 ranked as the 11th fastest computer in the world. That machine simulates the world’s weather each morning, and then simulates the world’s climate in the afternoon. It is a 16-petaflop machine, processing around 1,000 trillion bits per second.
A Six Word Novel
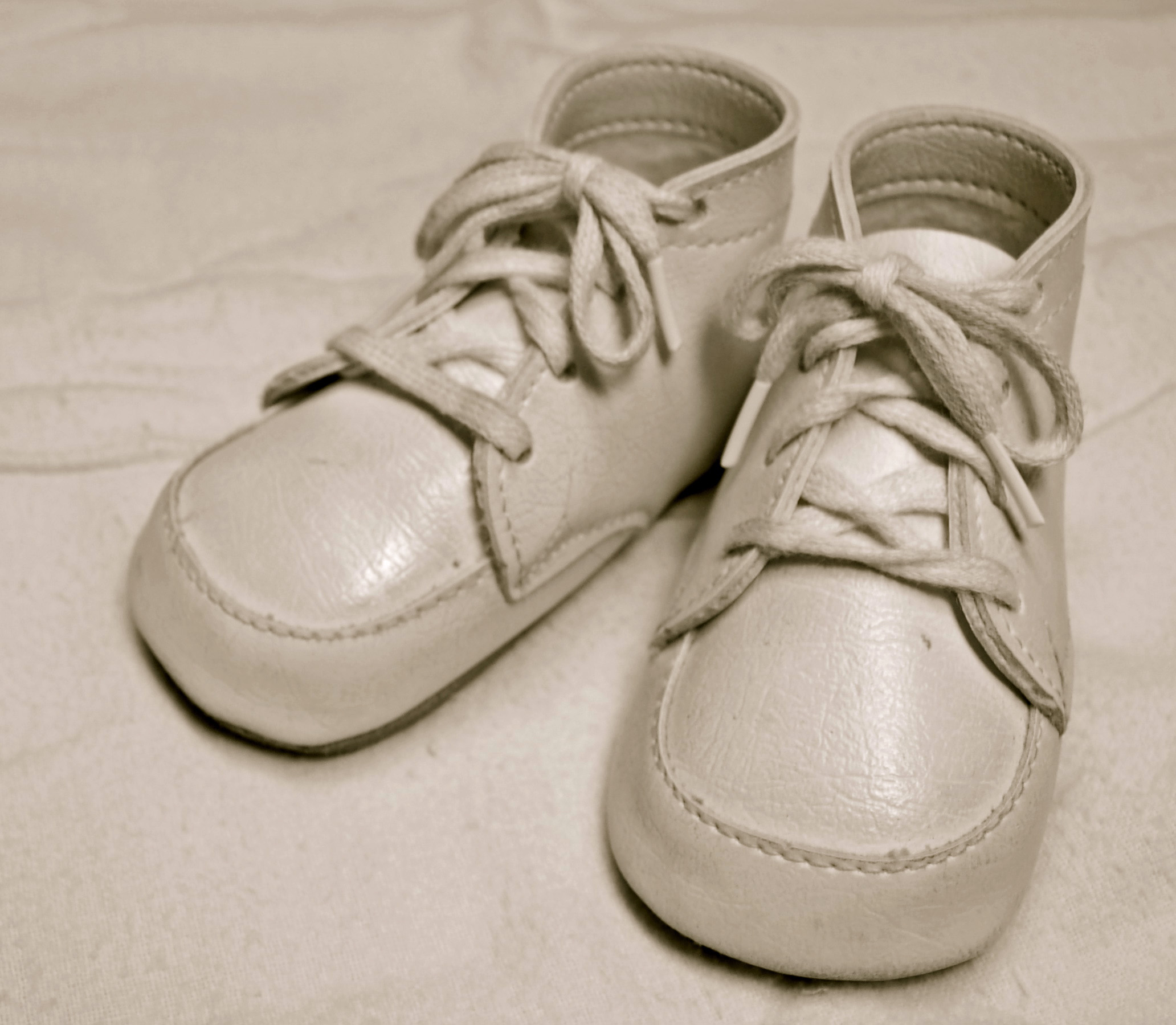
Figure: Consider the six-word novel, apocryphally credited to Ernest Hemingway, “For sale: baby shoes, never worn”. To understand what that means to a human, you need a great deal of additional context. Context that is not directly accessible to a machine that has not got both the evolved and contextual understanding of our own condition to realize both the implication of the advert and what that implication means emotionally to the previous owner.
But this is a very different kind of intelligence than ours. A computer cannot understand the depth of the Ernest Hemingway’s apocryphal six-word novel: “For Sale, Baby Shoes, Never worn”, because it isn’t equipped with that ability to model the complexity of humanity that underlies that statement.
Revolution
Arguably the information revolution we are experiencing is unprecedented in history. But changes in the way we share information have a long history. Over 5,000 years ago in the city of Uruk, on the banks of the Euphrates, communities which relied on the water to irrigate their corps developed an approach to recording transactions in clay. Eventually the system of recording system became sophisticated enough that their oral histories could be recorded in the form of the first epic: Gilgamesh.
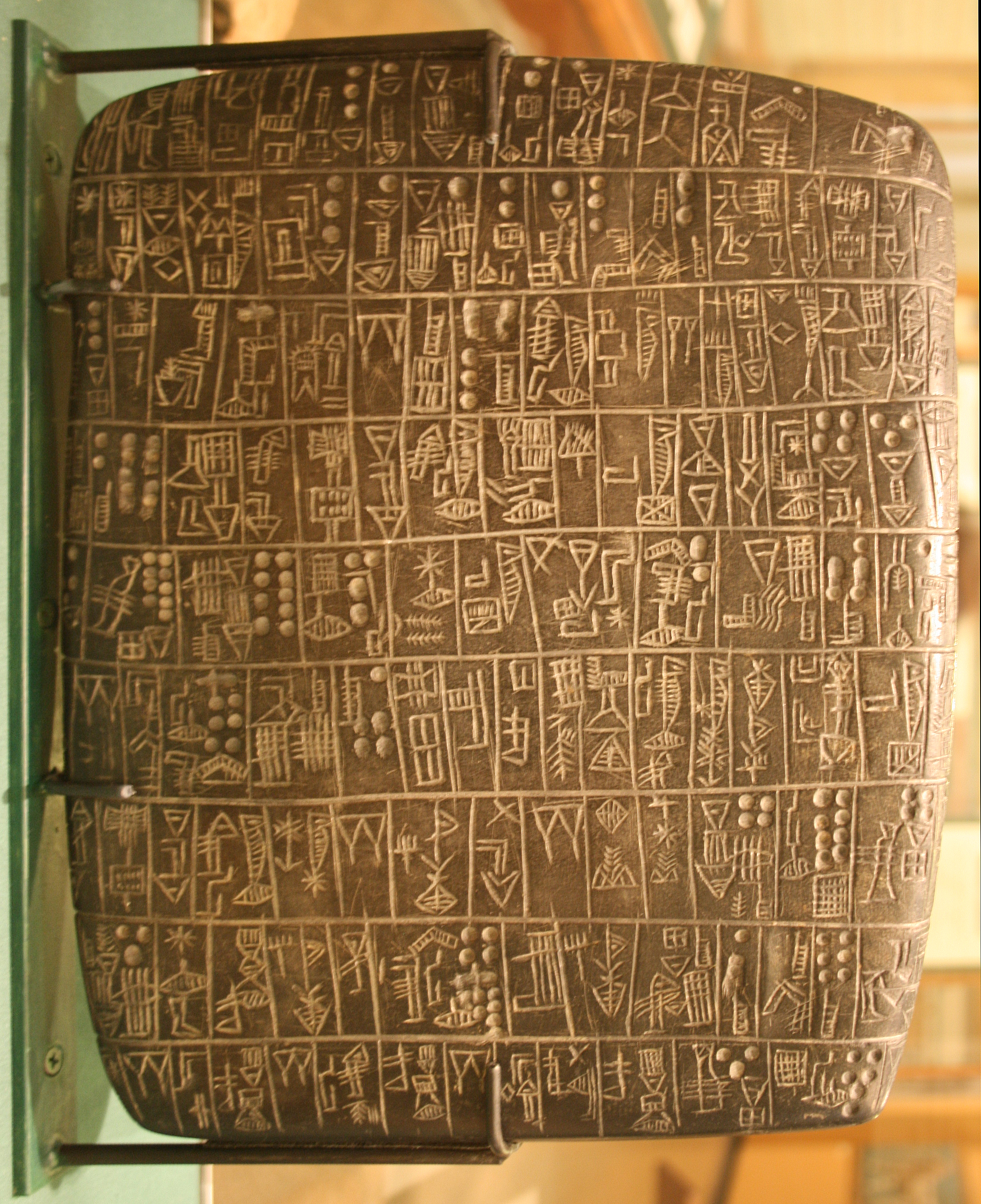
Figure: Chicago Stone, side 2, recording sale of a number of fields, probably from Isin, Early Dynastic Period, c. 2600 BC, black basalt
It was initially develoepd for people as a recordd of who owed what to whom, expanding individuals’ capacity to remember. But over a five hundred year period writing evolved to become a tool for literature as well. More pithily put, writing was invented by accountants not poets (see e.g. this piece by Tim Harford has highlighted.
In some respects today’s revolution is different, because it involves also the creation of stories as well as their curation. But in some fundamental ways we can see what we have produced as another tool for us in the information revolution.
The Future of Professions
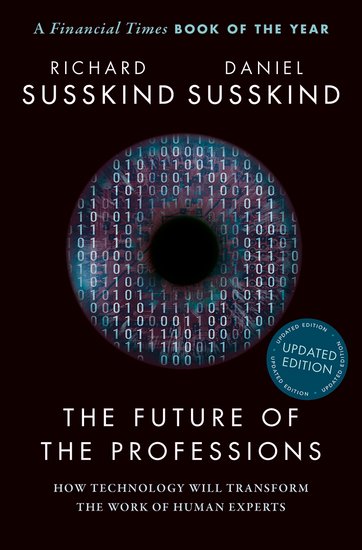
Figure: The Future of Professions (Susskind and Susskind, 2015) is a 2015 book focussed on how the next wave of technology revolution is going to effect the professions.
And this is very likely true, but in practice we know that even if the disruption is being felt initially by the professional classes, those groups tend to be protected by their ability to adapt, which is correlated with higher education.
Whether this remains true this time is another question. I’m particularly struck by the “convergent evolution” of ChatGPT. The model is trained by reinforcement learning with feedback provided by people. ChatGPT’s answers are highly plausible, make use of sophisticated language in an intelligent sounding way and are often incorrect. I’m struck by the similarity to fresh Oxbridge graduates. I wonder if this is also an example of convergent evolution.
Coin Pusher
Disruption of society is like a coin pusher, it’s those who are already on the edge who are most likely to be effected by disruption.
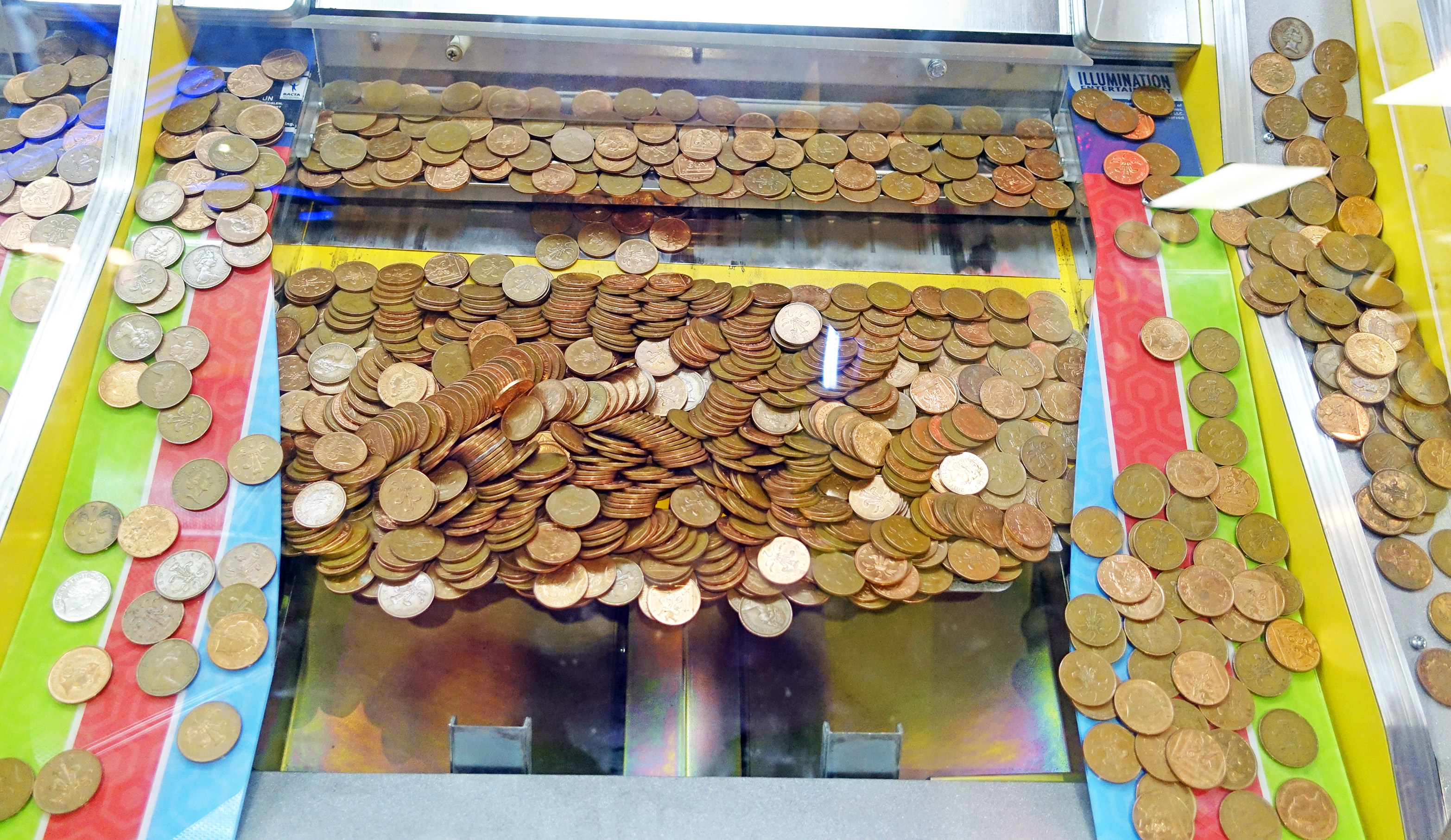
Figure: A coin pusher is a game where coins are dropped into th etop of the machine, and they disrupt those on the existing steps. With any coin drop, many coins move, but it is those on the edge, who are often only indirectly effected, but also most traumatically effected by the change.
One danger of the current hype around ChatGPT is that we are overly focussing on the fact that it seems to have significant effect on professional jobs, people are naturally asking the question “what does it do for my role?”. No doubt, there will be disruption, but the coin pusher hypothesis suggests that that disruption will likely involve movement on the same step. However it is those on the edge already, who are often not working directly in the information economy, who often have less of a voice in the policy conversation who are likely to be most disrupted.
I’m reminded of this because from 2015 to 2017 I was on the Working Group that compiled the Royal Society’s machine learning report. The process of constructing the report went across the UK Referendum, and the 2016 US election. I remember vividly a meeting we convened at the Society in London which had experts alongside MPs from all parties, policy advisors and civil servants. One of the MPs (likely correctly) pointed out “I suspect no one around this table voted for Brexit” to which I replied “But isn’t that the problem? There are a large number of people who aren’t empowered who are experiencing quite a different reality than us. And they aren’t reprented in these forums.” So it’s no surprise that so much of the press conversation around AI is still focussed on how it is likely to effect middle class jobs. We shouldn’t underestimate these effects, but it’s often the case that better educated people are better placed to deal with such challenges. For example, when stock brokers’ roles disappeared they simply moved on to other roles in banks and related industries.
Royal Society Report
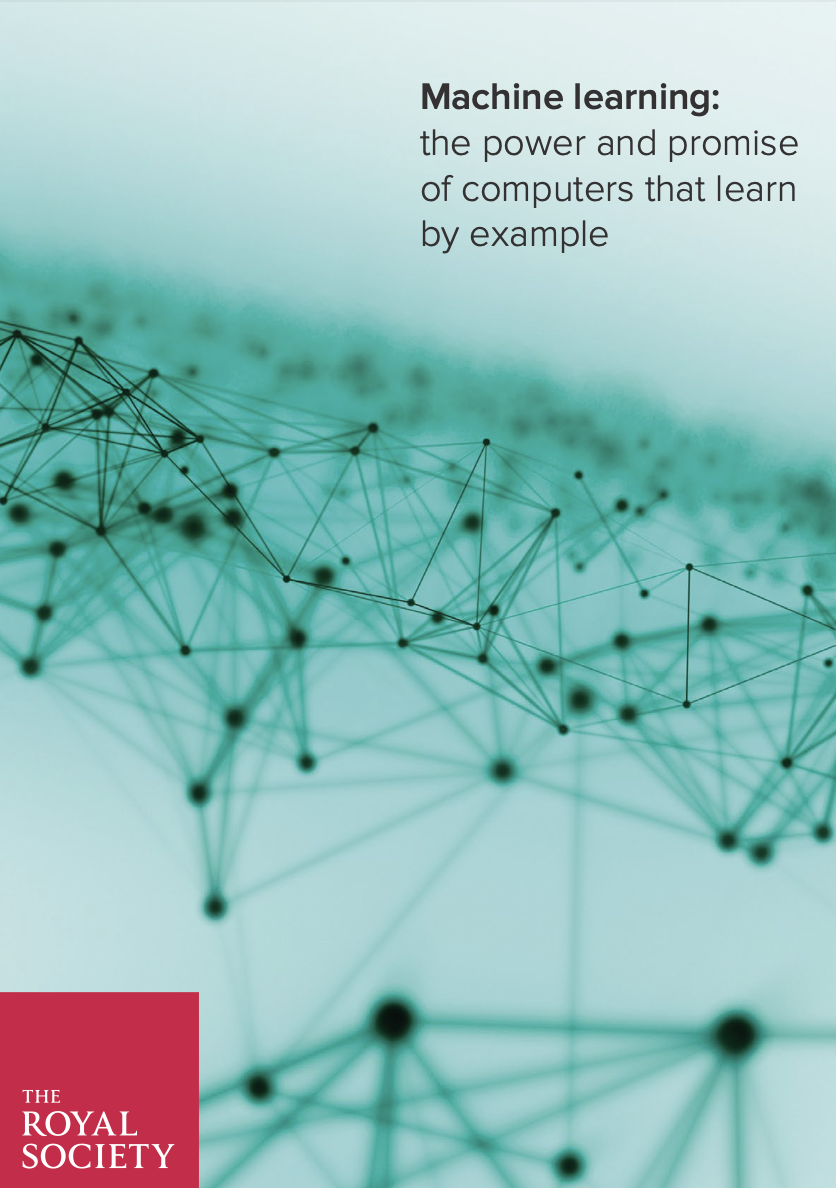
Figure: The Royal Society report on Machine Learning was released on 25th April 2017
A useful reference for state of the art in machine learning is the UK Royal Society Report, Machine Learning: Power and Promise of Computers that Learn by Example.
Public Research
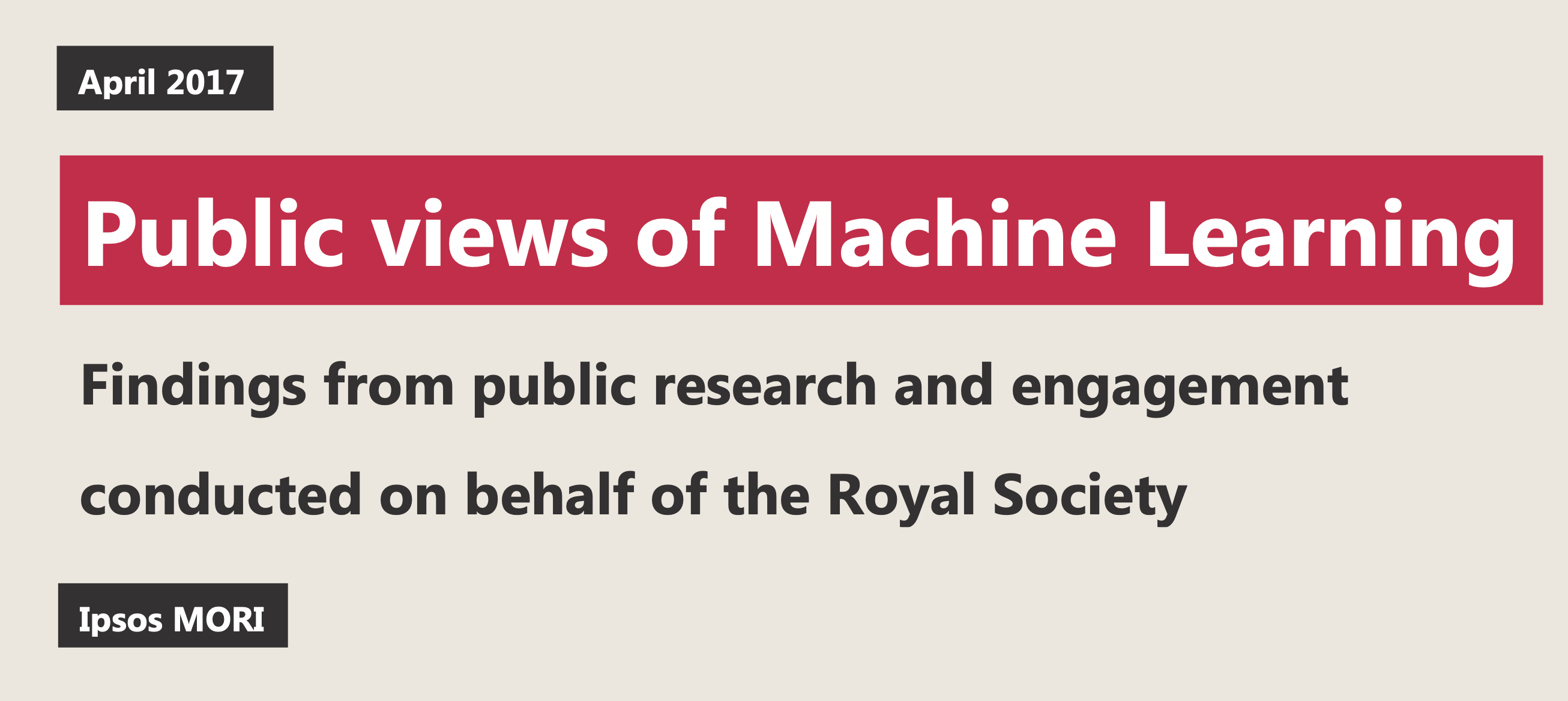
Figure: The Royal Society comissioned public research from Mori as part of the machine learning review.
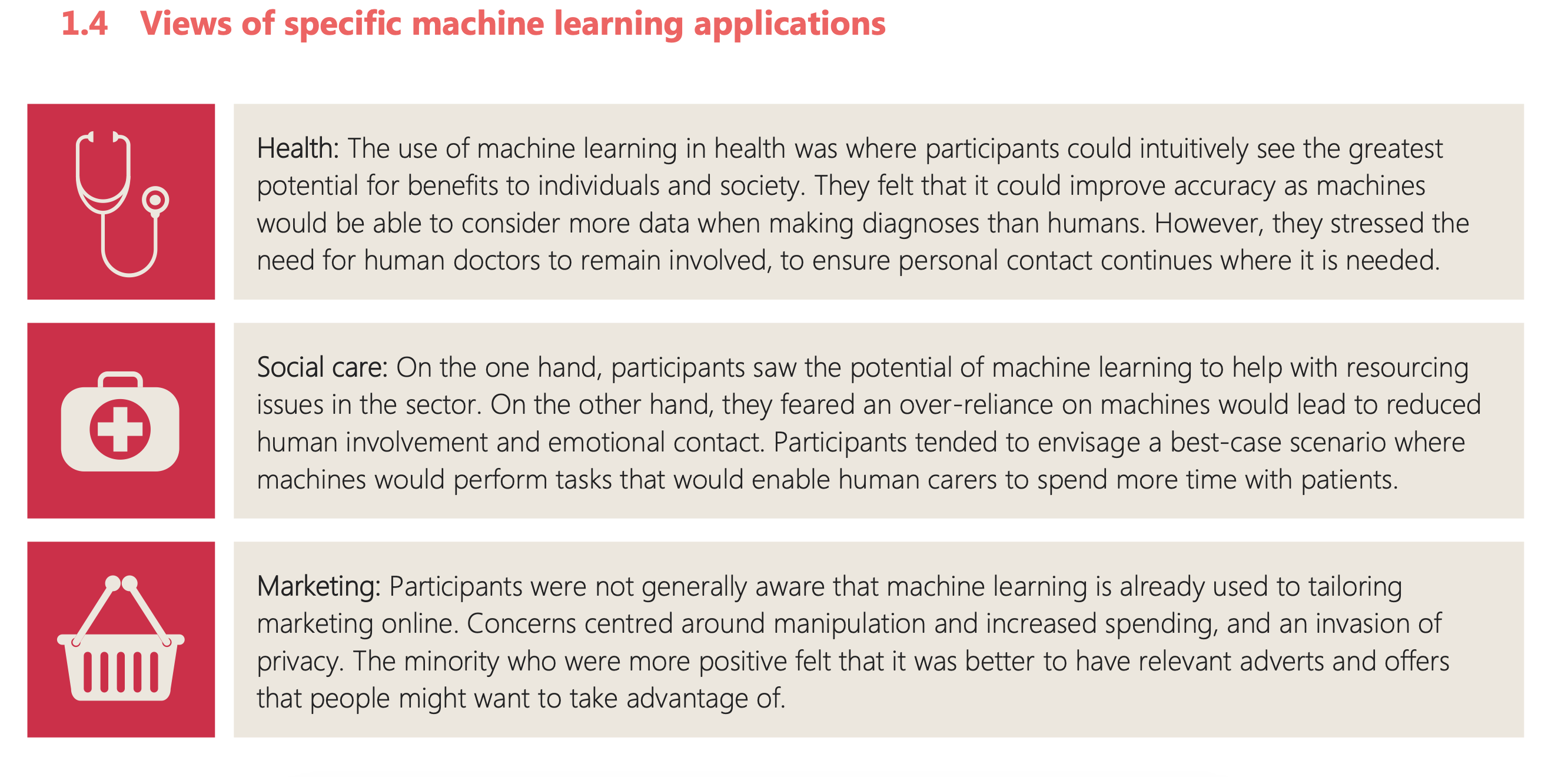
Figure: One of the questions focussed on machine learning applications.
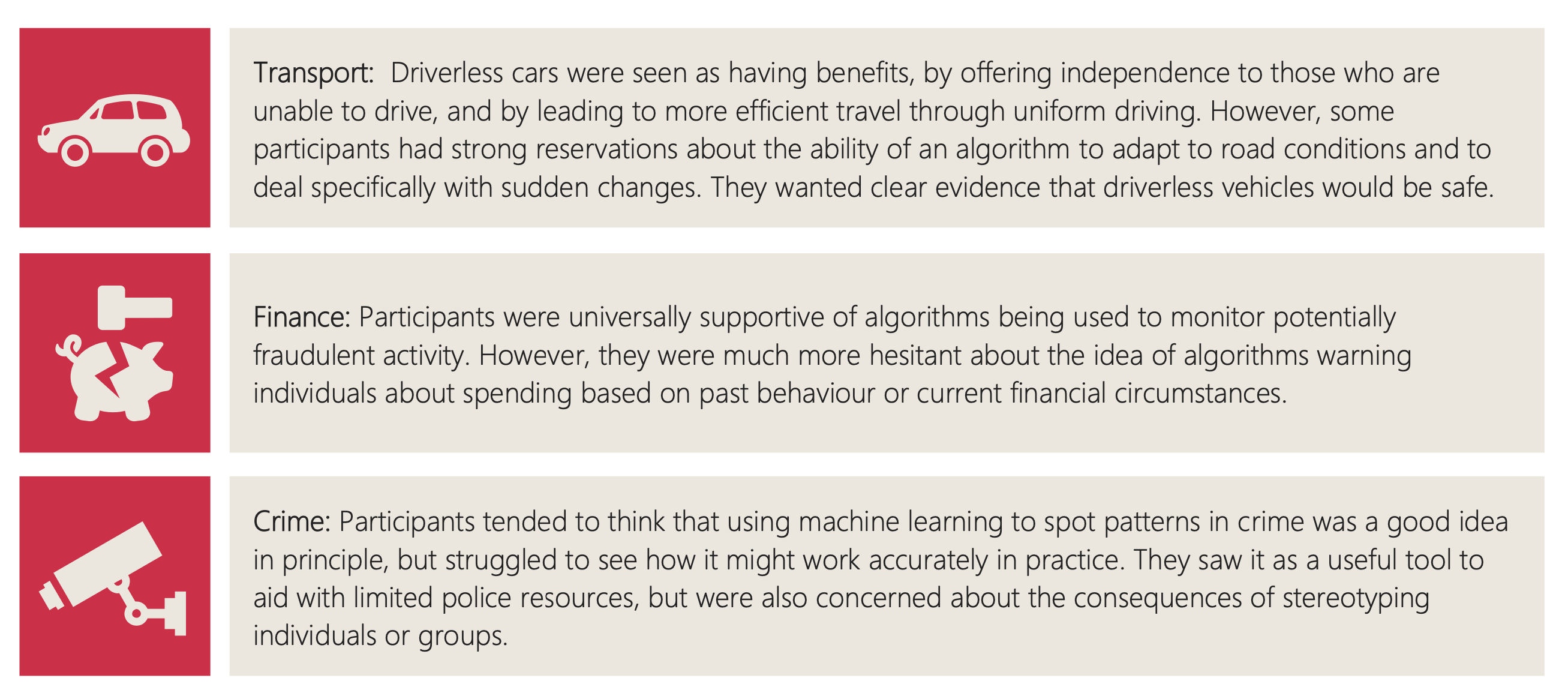
Figure: The public were broadly supportive of a range of application areas.
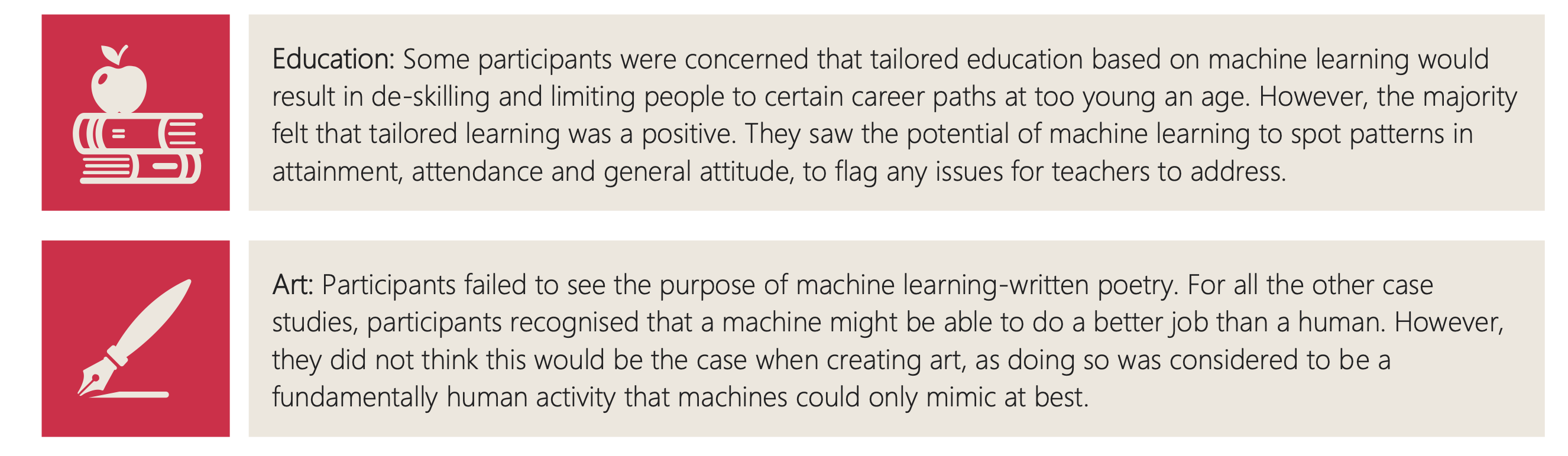
Figure: But they failed to see the point in AI’s that could produce poetry.
Mercutio
It’s ironic that despite the public focus on transport, health, cities, crime and their confusion as to why we might want AI to do art, one area where significant progress has been made is on AIs that can create literature.
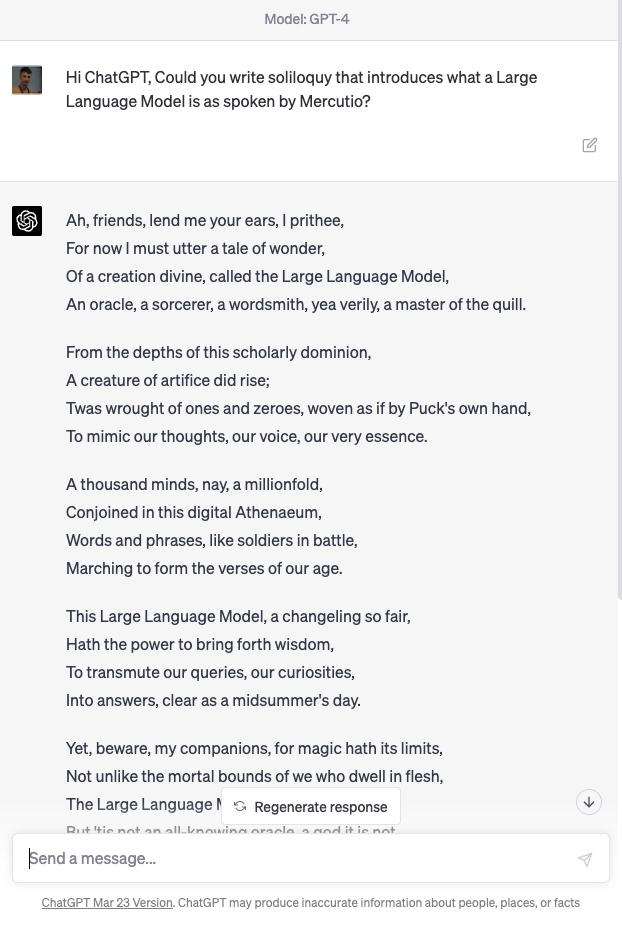
Figure: Despite the public’s desire to see challenges in health, finance, transport, crime solved by machine learning and their skepticism around poetry, one of the problems we have solved seems to be poetry.
User: Hi ChatGPT, Could you write soliloquy that introduces what a Large Language Model is as spoken by Mercutio?
Machine: Ah, friends, lend me your ears, I prithee, For now I must utter a tale of wonder, Of a creation divine, called the Large Language Model, An oracle, a sorcerer, a wordsmith, yea verily, a master of the quill.
From the depths of this scholarly dominion, A creature of artifice did rise; Twas wrought of ones and zeroes, woven as if by Puck’s own hand, To mimic our thoughts, our voice, our very essence.
A thousand minds, nay, a millionfold, Conjoined in this digital Athenaeum, Words and phrases, like soldiers in battle, Marching to form the verses of our age.
This Large Language Model, a changeling so fair, Hath the power to bring forth wisdom, To transmute our queries, our curiosities, Into answers, clear as a midsummer’s day.
Yet, beware, my companions, for magic hath its limits, Not unlike the mortal bounds of we who dwell in flesh, The Large Language Model hath its strengths, But ’tis not an all-knowing oracle, a god it is not.
As Mercutio, your humble servant, doth stand before thee, I marvel at the art and science that hath brought forth this wondrous creation, This Large Language Model, a testament to man’s yearning, To comprehend the world and make meaning of the great tapestry of existence.
In faith, dear friends, let us embrace the magic and beauty of this digital bard, And mayhaps, by its grace, we shall glean some wisdom, As we wander together, hand in hand, Through this boundless realm of knowledge and wonder.
ChatGPT transcript, 14th April 2023.
Weather
So what’s going on here? One analogy I like to use is with weather forecasting. Historically, before the use of computer driven weather forecasting, we used a process of interpolation to measure the pressure.
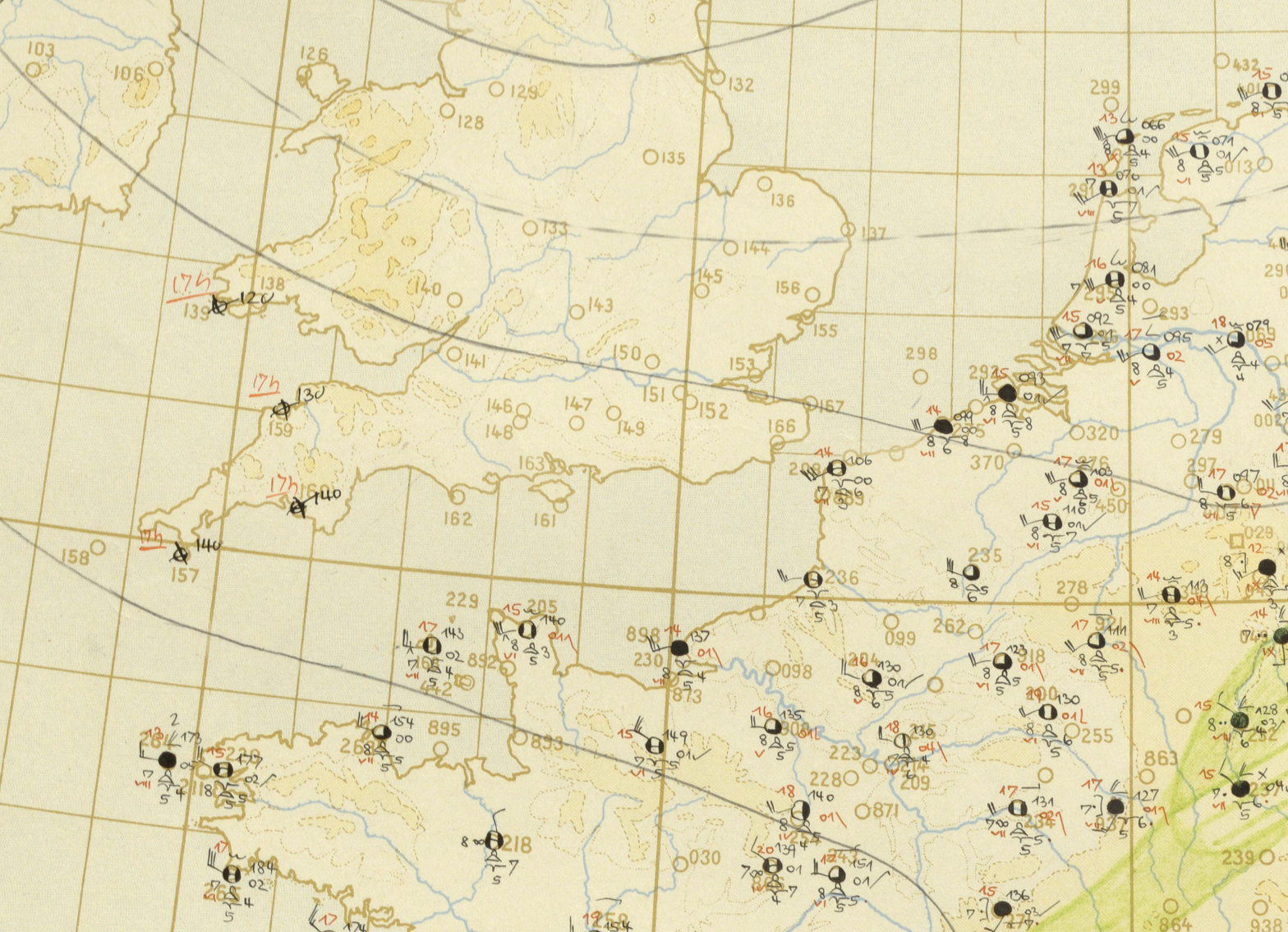
Figure: Forecast from UK Met Office on 5th June 1944. (detail from https://www.metoffice.gov.uk/research/library-and-archive/archive-hidden-treasures/d-day)
This was problematic for German forces in the Second World War because they had no ability to predict the weather when it was coming in from across the UK. Conversely, the UK had a number of weather stations in the UK, and some information (perhaps from spies or Enigma decrypts) about weather on the mainland.
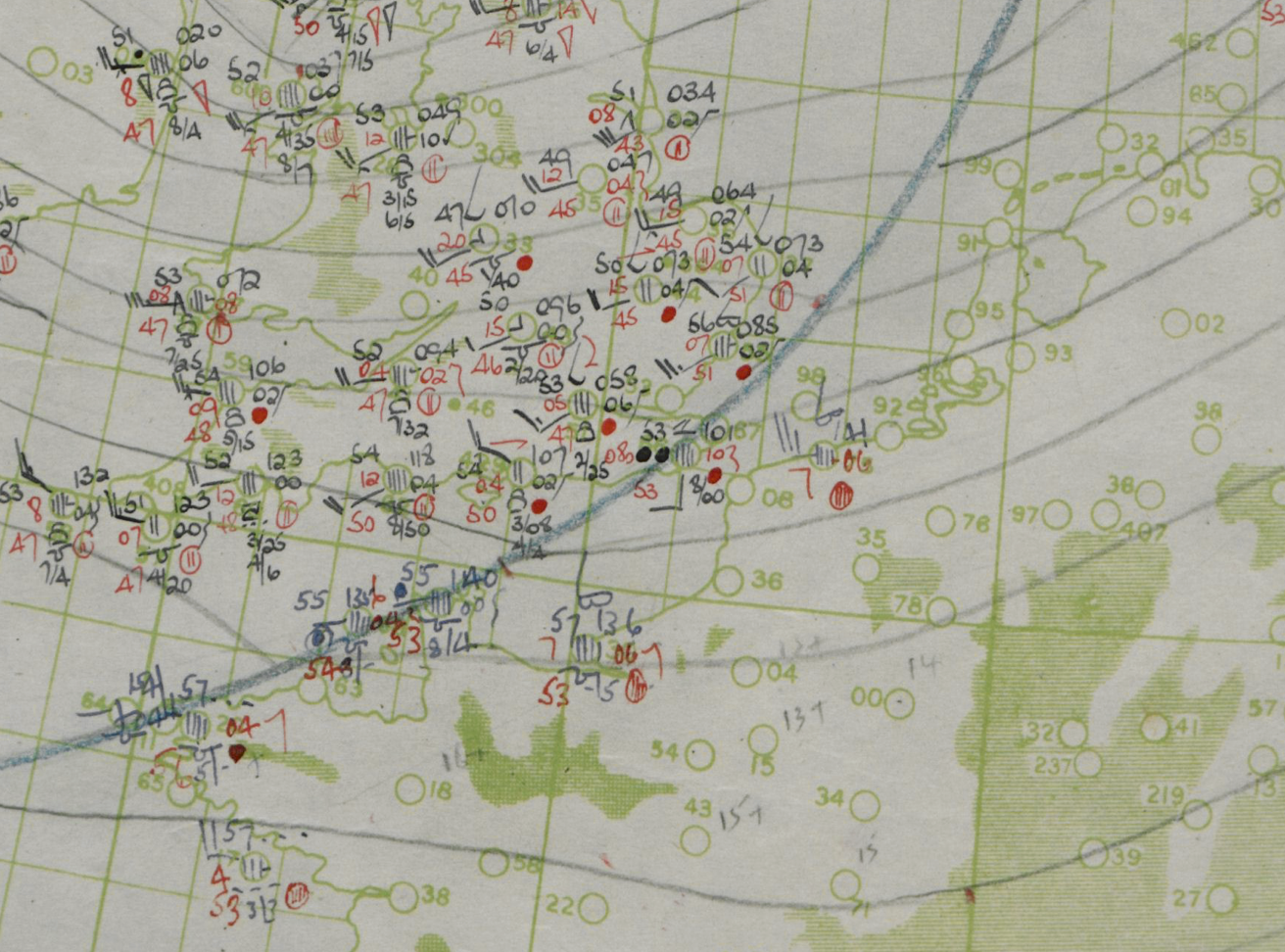
Figure: Forecast from Deutscher Wetterdienst on 5th June 1944. (detail from https://www.metoffice.gov.uk/research/library-and-archive/archive-hidden-treasures/d-day). Note the lack of measurements within the UK. THis is the direction that weather was coming from so the locaiton of weather fronts (and associated storms) was harder for the Deutscher Wetterdienst to predict than the Met Office.
This meant that more accurate forecasts were possible for D-Day for the Allies than for the defending forces. As a result, on the morning that Eisenhower invated, Rommel was back in Germany attending his wife’s 50th birthday party.
Modern artificial intelligence solutions are using very large amounts of data to build a landscape in which this interpolation can take place. Tools like ChatGPT are allowing us to interpolate between different human concepts. This is an amazing achievement, but it is also a challenge.
\(p\)-Fairness and \(n\)-Fairness
Again Univesities are to treat each applicant fairly on the basis of ability and promise, but they are supposed also to admit a socially more representative intake.
There’s no guarantee that the process meets the target.
Onora O’Neill A Question of Trust: Called to Account Reith Lectures 2002 O’Neill (2002)]
Figure: We seem to have two different aspects to fairness, which in practice can be in tension.
We’ve outlined \(n\)-fairness and \(p\)-fairness. By \(n\)-fairness we mean the sort of considerations that are associated with substantive equality of opportunity vs formal equality of opportunity. Formal equality of community is related to \(p\)-fairness. This is sometimes called procedural fairness and we might think of it as a performative form of fairness. It’s about clarity of rules, for example as applied in sport. \(n\)-Fairness is more nuanced. It’s a reflection of society’s normative judgment about how individuals may have been disadvantaged, e.g. due to their upbringing.
The important point here is that these forms of fairness are in tension. Good procedural fairness needs to be clear and understandable. It should be clear to everyone what the rules are, they shouldn’t be obscured by jargon or overly subtle concepts. \(p\)-Fairness should not be easily undermined by adversaries, it should be difficult to “cheat” good \(p\)-fairness. However, \(n\)-fairness requires nuance, understanding of the human condition, where we came from and how different individuals in our society have been advantaged or disadvantaged in their upbringing and their access to opportunity.
Pure \(n\)-fairness and pure \(p\)-fairness both have the feeling of dystopias. In practice, any decision making system needs to balance the two. The correct point of operation will depend on the context of the decision. Consider fair rules of a game of football, against fair distribution of social benefit. It is unlikely that there is ever an objectively correct balance between the two for any given context. Different individuals will favour \(p\) vs \(n\) according to their personal values.
Given the tension between the two forms of fairness, with \(p\) fairness requiring simple rules that are understandable by all, and \(n\) fairness requiring nuance and subtlety, how do we resolve this tension in practice?
Normally in human systems, significant decisions involve trained professionals. For example, judges, or accountants or doctors.
Training a professional involves lifting their “reflexive” response to a situation with “reflective” thinking about the consequences of their decision that rely not just on the professional’s expertise, but also their knowledge of what it is to be a human.
This marvellous resolution exploits the fact that while humans are increadibly complicated nuanced entities, other humans have an intuitive ability to understand their motivations and values. So the human is a complex entity that seems simple to other humans.
A Question of Trust
In Baroness Onora O’Neill’s Reeith Lectures from 2002, she raises the challenge of trust. There are many aspects to her arcuments, but one of the key points she makes is that we cannot trust without the notion of duty. O’Neill is bemoaning the substitution of duty with process. The idea is that processes and transparency are supposed to hold us to account by measuring outcomes. But these processes themselves overwhelm decision makers and undermine their professional duty to deliver the right outcome.
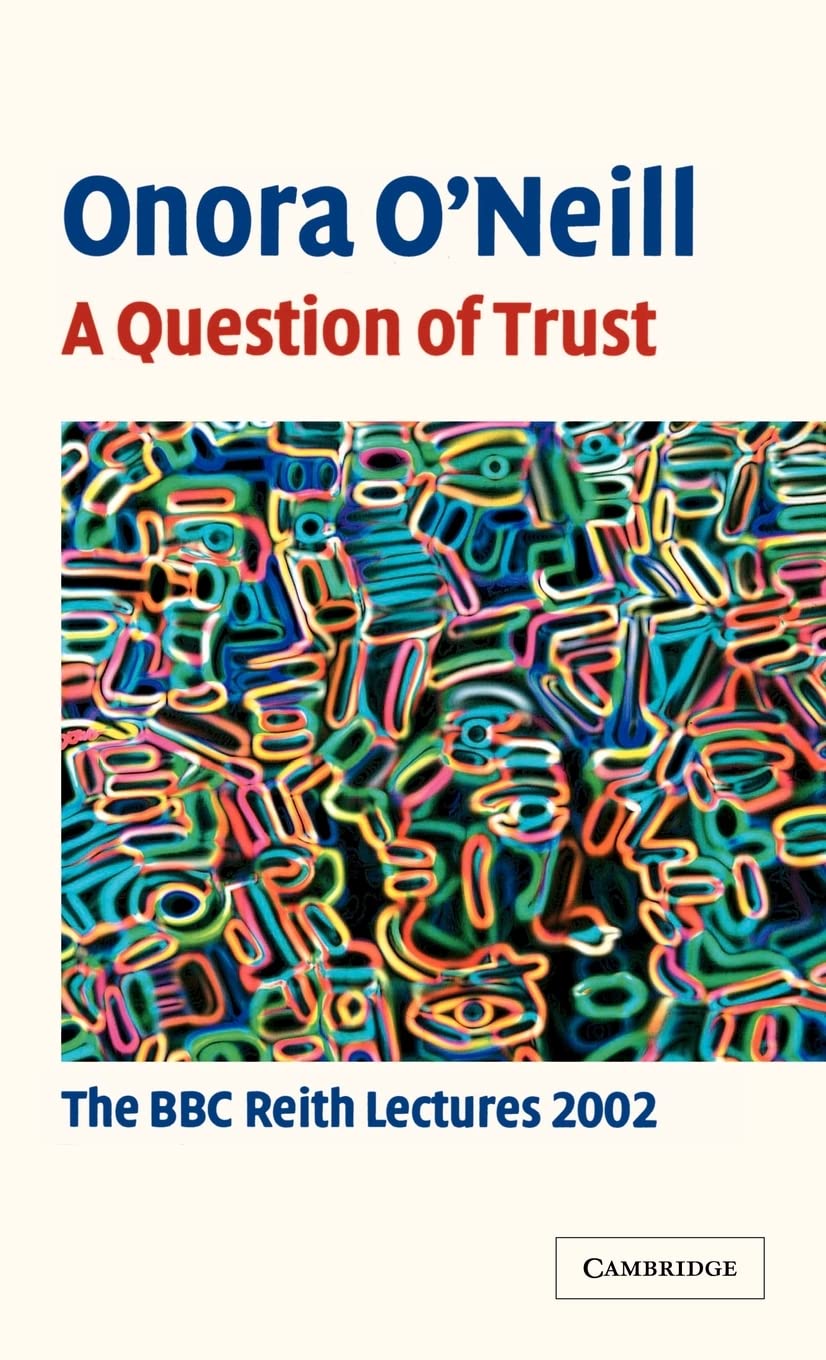
Figure: A Question of Trust by Onora O’Neil which examines the nature of trust and its role in society.
O’Neill is speaking in 2002, in the early days of the internet and before social media. Much of her thoughts are even more relevant for today than they were when she spoke. This is because the increased availability of information and machine driven decision-making makes the mistaken premise, that process is an adequate substitute for duty, more apparently plausible. But this undermines what O’Neill calls “intelligent accountability”, which is not accounting by the numbers, but through professional education and institutional safeguards.
NACA Langley
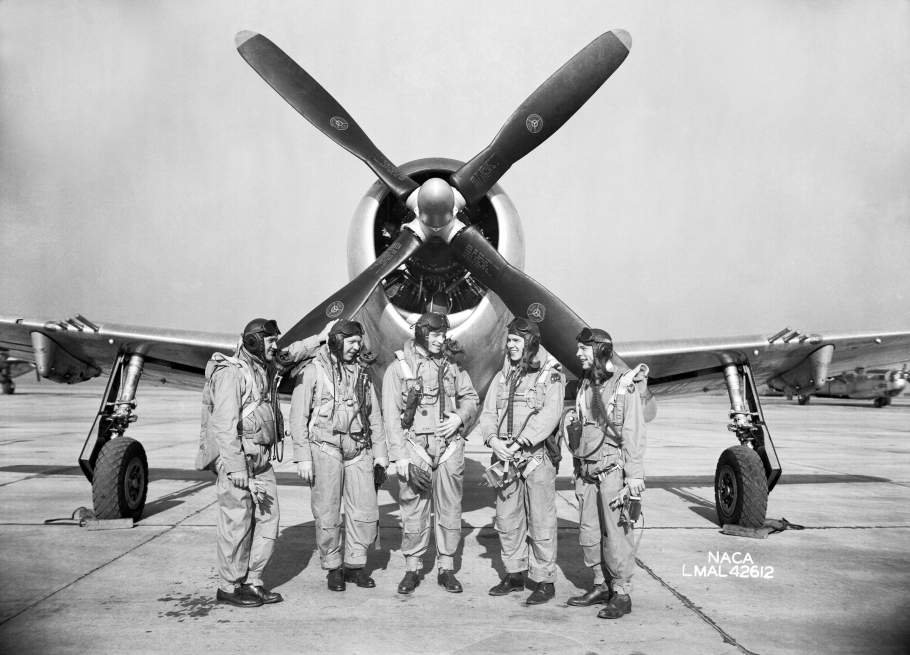
Figure: 1945 photo of the NACA test pilots, from left Mel Gough, Herb Hoover, Jack Reeder, Stefan Cavallo and Bill Gray (photo NASA, NACA LMAL 42612)
The NACA Langley Field proving ground tested US aircraft. Bob Gilruth worked on the flying qualities of aircraft. One of his collaborators suggested that
Hawker Hurricane airplane. A heavily armed fighter airplane noted for its role in the Battle of Britain, the Hurricane’s flying qualities were found to be generally satisfactory. The most notable deficiencies were heavy aileron forces at high speeds and large friction in the controls.
W. Hewitt Phillips2
and
Supermarine Spitfire airplane. A high-performance fighter noted for its role in the Battle of Britain and throughout WW II, the Spitfire had desirably light elevator control forces in maneuvers and near neutral longitudinal stability. Its greatest deficiency from the combat standpoint was heavy aileron forces and sluggish roll response at high speeds.
W. Hewitt Phillips3
Gilruth went beyond the reports of feel to characterise how the plane should respond to different inputs on the control stick. In other words he quantified that feel of the plane.
Innovating to serve science and society requires a pipeline of interventions. As well as advances in the technical capabilities of AI technologies, engineering knowhow is required to safely deploy and monitor those solutions in practice. Regulatory frameworks need to adapt to ensure trustworthy use of these technologies. Aligning technology development with public interests demands effective stakeholder engagement to bring diverse voices and expertise into technology design.
Building this pipeline will take coordination across research, engineering, policy and practice. It also requires action to address the digital divides that influence who benefits from AI advances. These include digital divides within the socioeconomic strata that need to be overcome – AI must not exacerbate existing equalities or create new ones. In addressing these challenges, we can be hindered by divides that exist between traditional academic disciplines. We need to develop common understanding of the problems and a shared knowledge of possible solutions.
Making AI equitable
AI@Cam is a new flagship University mission that seeks to address these challenges. It recognises that development of safe and effective AI-enabled innovations requires this mix of expertise from across research domains, businesses, policy-makers, civill society, and from affected communities. AI@Cam is setting out a vision for AI-enabled innovation that benefits science, citizens and society.
This vision will be achieved through leveraging the University’s vibrant interdisciplinary research community. AI@Cam will form partnerships between researchers, practitioners, and affected communities that embed equity and inclusion. It will develop new platforms for innovation and knowledge transfer. It will deliver innovative interdisciplinary teaching and learning for students, researchers, and professionals. It will build strong connections between the University and national AI priorities.
The University operates as both an engine of AI-enabled innovation and steward of those innovations.
AI is not a universal remedy. It is a set of tools, techniques and practices that correctly deployed can be leveraged to deliver societal benefit and mitigate social harm.
In that sense AI@Cam’s mission is close in spirit to that of Panacea’s elder sister Hygeia. It is focussed on building and maintaining the hygiene of a robust and equitable AI research ecosystem.
Data Science Africa
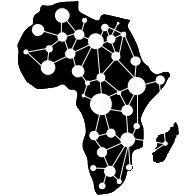
Figure: Data Science Africa http://datascienceafrica.org is a ground up initiative for capacity building around data science, machine learning and artificial intelligence on the African continent.
Figure: Data Science Africa meetings held up to October 2021.
Data Science Africa is a bottom up initiative for capacity building in data science, machine learning and artificial intelligence on the African continent.
As of October 2021 there have been five workshops and five schools, located in Nyeri, Kenya (twice); Kampala, Uganda; Arusha, Tanzania; Abuja, Nigeria; Addis Ababa, Ethiopia; Accra, Ghana; Kampala, Uganda and Kimberley, South Africa.
The main notion is end-to-end data science. For example, going from data collection in the farmer’s field to decision making in the Ministry of Agriculture. Or going from malaria disease counts in health centers to medicine distribution.
The philosophy is laid out in (Lawrence, 2015). The key idea is that the modern information infrastructure presents new solutions to old problems. Modes of development change because less capital investment is required to take advantage of this infrastructure. The philosophy is that local capacity building is the right way to leverage these challenges in addressing data science problems in the African context.
Data Science Africa is now a non-govermental organization registered in Kenya. The organising board of the meeting is entirely made up of scientists and academics based on the African continent.
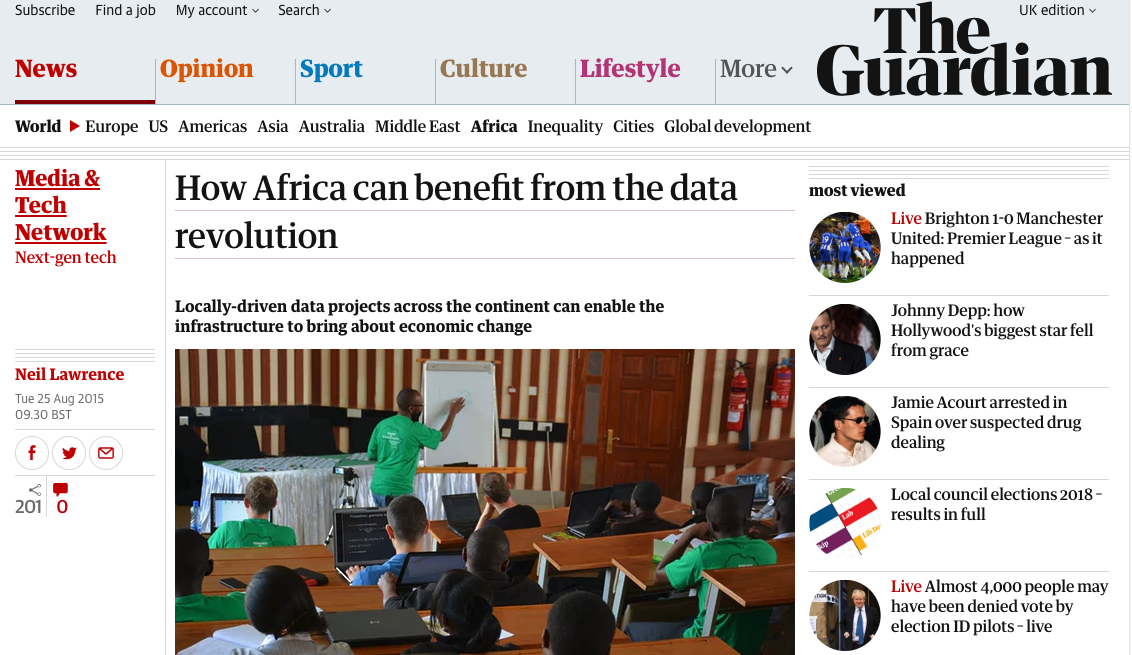
Figure: The lack of existing physical infrastructure on the African continent makes it a particularly interesting environment for deploying solutions based on the information infrastructure. The idea is explored more in this Guardian op-ed on Guardian article on How African can benefit from the data revolution.
Guardian article on Data Science Africa
AI@Cam
Finally, we are working across the University to empower the diversity ofexpertise and capability we have to focus on these broad societal problems. We will recently launched AI@Cam with a vision document that outlines these challenges for the University.
The initiative was funded in November 2022 where a £5M investment from the University was secured.
Progress so far: * Developing the vision * Engaged over 100 members of the University community across 30 departments/institutes, start-ups, and large businesses. * Supported 6 new funding bids}
The Accelerate Programme
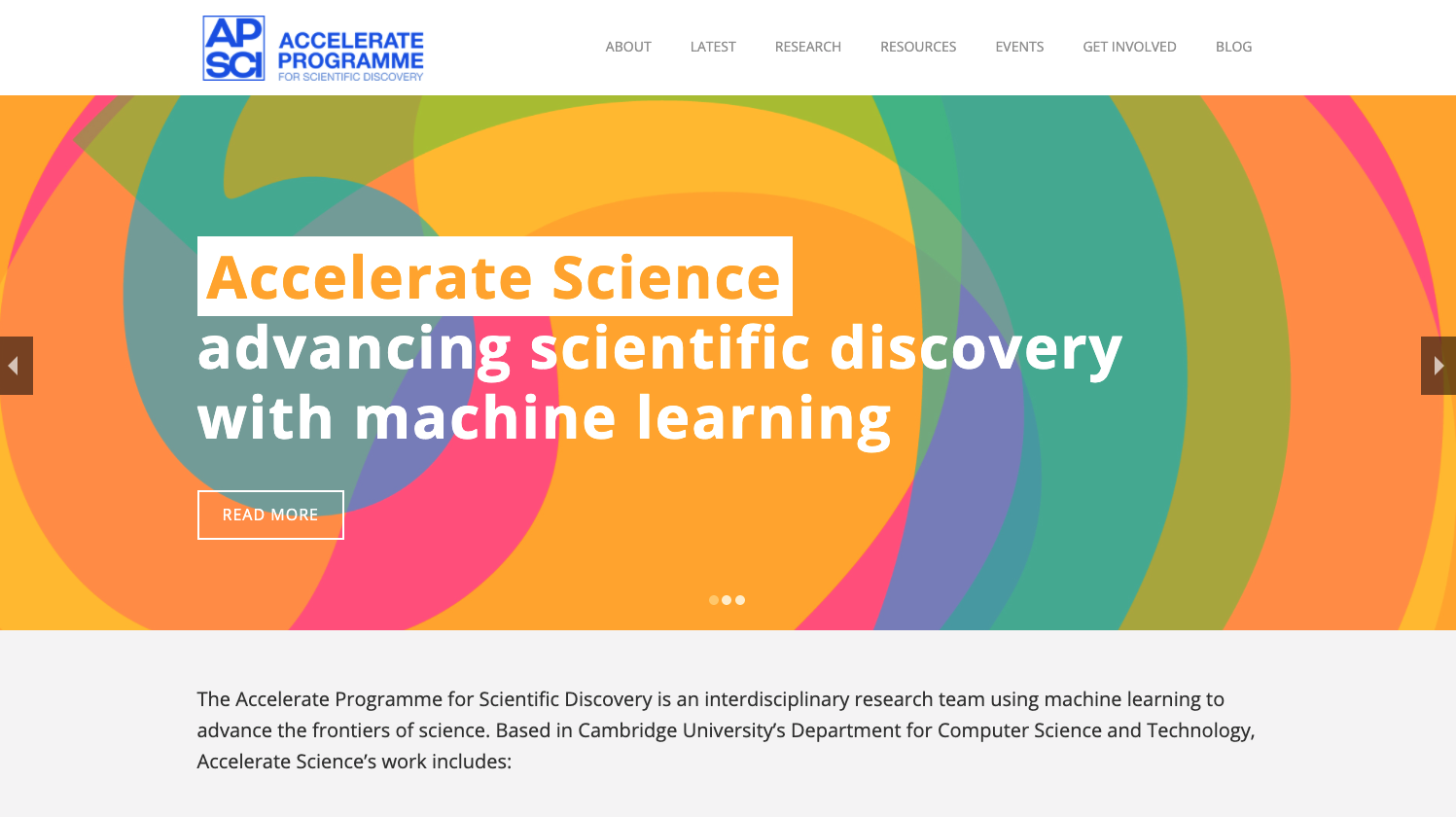
Figure: The Accelerate Programme for Scientific Discovery covers research, education and training, engagement. Our aim is to bring about a step change in scientific discovery through AI. http://acceleratescience.github.io
We’re now in a new phase of the development of computing, with rapid advances in machine learning. But we see some of the same issues – researchers across disciplines hope to make use of machine learning, but need access to skills and tools to do so, while the field machine learning itself will need to develop new methods to tackle some complex, ‘real world’ problems.
It is with these challenges in mind that the Computer Lab has started the Accelerate Programme for Scientific Discovery. This new Programme is seeking to support researchers across the University to develop the skills they need to be able to use machine learning and AI in their research.
To do this, the Programme is developing three areas of activity:
- Research: we’re developing a research agenda that develops and applies cutting edge machine learning methods to scientific challenges, with three Accelerate Research fellows working directly on issues relating to computational biology, psychiatry, and string theory. While we’re concentrating on STEM subjects for now, in the longer term our ambition is to build links with the social sciences and humanities.
Progress so far includes: * Recruited a core research team working on the application of AI in mental health, bioinformatics, healthcare, string theory, and complex systems. * Funded 9 interdisciplinary projects: * Antimicrobial resistance in farming * Quantifying Design Trade-offs in Electricity-generation-focused Tokamaks using AI * Automated preclinical drug discovery in vivo using pose estimation * Causal Methods for Environmental Science Workshop * Automatic tree mapping in Cambridge * Acoustic monitoring for biodiversity conservation * AI, mathematics and string theory * Theoretical, Scientific, and Philosophical Perspectives on Biological Understanding in the age of Artificial Intelligence * AI in pathology: optimising a classifier for digital images of duodenal biopsies
- Teaching and learning: building on the teaching activities already delivered through University courses, we’re creating a pipeline of learning opportunities to help PhD students and postdocs better understand how to use data science and machine learning in their work.
Progress so far includes: Teaching and learning * Brought over 250 participants from over 30 departments through tailored data science and machine learning for science training (Data Science Residency and Machine Learning Academy); * Convened workshops with over 80 researchers across the University on the development of data pipelines for science; * Delivered University courses to over 100 students in Advanced Data Science and Machine Learning and the Physical World.
- Engagement: we hope that Accelerate will help build a community of researchers working across the University at the interface on machine learning and the sciences, helping to share best practice and new methods, and support each other in advancing their research. Over the coming years, we’ll be running a variety of events and activities in support of this.
Progress so far includes: * Launched a Machine Learning Engineering Clinic that has supported over 40 projects across the University with MLE troubleshooting and advice; * Hosted and participated in events reaching over 300 people; * Created a roadmap for the development of AI in science, based on a Dagstuhl event that brought together 30 researchers from across the world.
Personal Data Trusts
The machine learning solutions we are dependent on to drive automated decision making are dependent on data. But with regard to personal data there are important issues of privacy. Data sharing brings benefits, but also exposes our digital selves. From the use of social media data for targeted advertising to influence us, to the use of genetic data to identify criminals, or natural family members. Control of our virtual selves maps on to control of our actual selves.
The feudal system that is implied by current data protection legislation has significant power asymmetries at its heart, in that the data controller has a duty of care over the data subject, but the data subject may only discover failings in that duty of care when it’s too late. Data controllers also may have conflicting motivations, and often their primary motivation is not towards the data-subject, but that is a consideration in their wider agenda.
Personal Data Trusts (Delacroix and Lawrence, 2018; Edwards, 2004; Lawrence, 2016) are a potential solution to this problem. Inspired by land societies that formed in the 19th century to bring democratic representation to the growing middle classes. A land society was a mutual organization where resources were pooled for the common good.
A Personal Data Trust would be a legal entity where the trustees’ responsibility was entirely to the members of the trust. So the motivation of the data-controllers is aligned only with the data-subjects. How data is handled would be subject to the terms under which the trust was convened. The success of an individual trust would be contingent on it satisfying its members with appropriate balancing of individual privacy with the benefits of data sharing.
Formation of Data Trusts became the number one recommendation of the Hall-Presenti report on AI, but unfortunately, the term was confounded with more general approaches to data sharing that don’t necessarily involve fiduciary responsibilities or personal data rights. It seems clear that we need to better characterize the data sharing landscape as well as propose mechanisms for tackling specific issues in data sharing.
It feels important to have a diversity of approaches, and yet it feels important that any individual trust would be large enough to be taken seriously in representing the views of its members in wider negotiations.
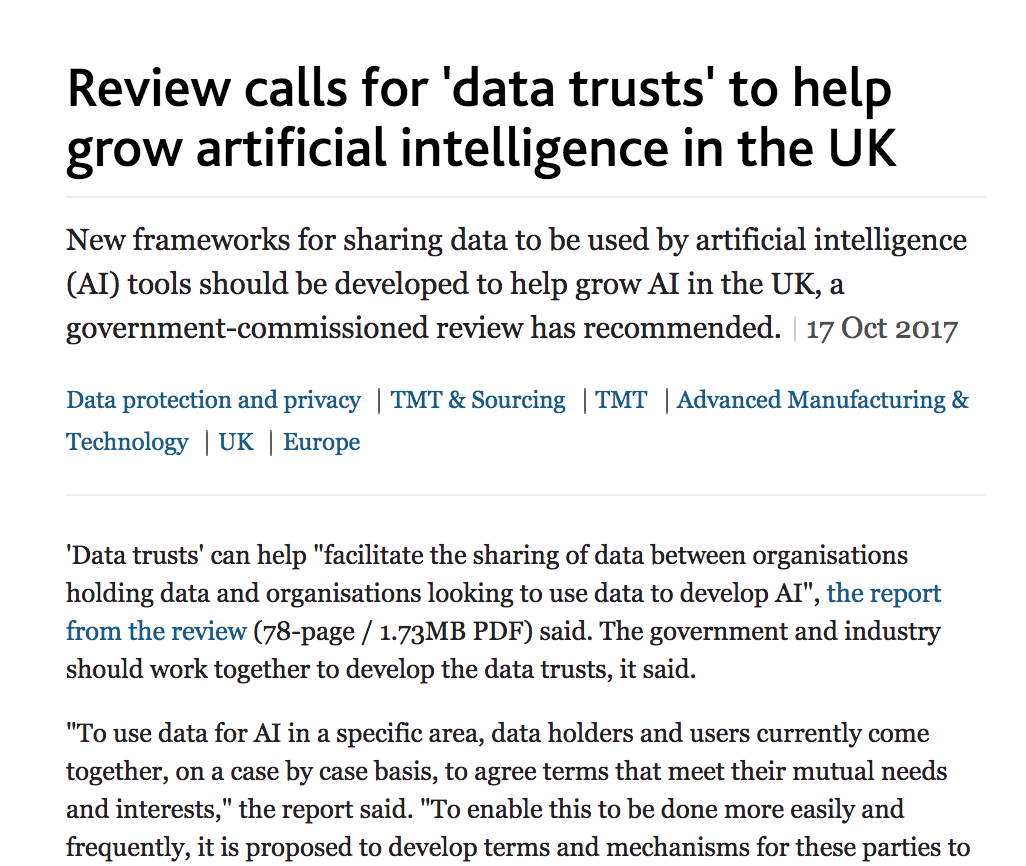
Figure: Data Trusts were the first recommendation of the Hall-Presenti Report. More recently the nature of different data intermediaries was clarified in a report on https://www.adalovelaceinstitute.org/report/legal-mechanisms-data-stewardship/ from the Ada Lovelace Institute.
See Guardian article on Digital Oligarchies and Guardian article on Information Feudalism.
Data Trusts Initiative
The Data Trusts Initiative, funded by the Patrick J. McGovern Foundation is supporting three pilot projects that consider how bottom-up empowerment can redress the imbalance associated with the digital oligarchy.
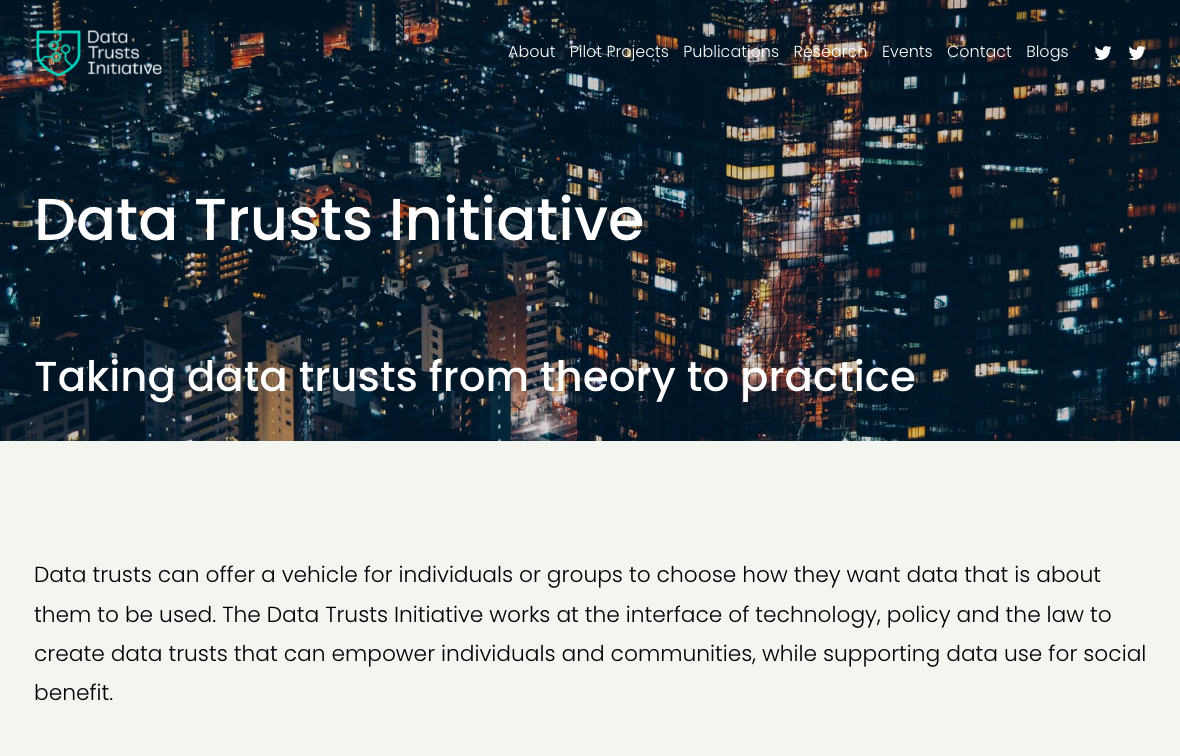
Figure: The Data Trusts Initiative (http://datatrusts.uk) hosts blog posts helping build understanding of data trusts and supports research and pilot projects.
Progress So Far
In its first 18 months of operation, the Initiative has:
Convened over 200 leading data ethics researchers and practitioners;
Funded 7 new research projects tackling knowledge gaps in data trust theory and practice;
Supported 3 real-world data trust pilot projects establishing new data stewardship mechanisms.
Building on the analogy of data rivers we use, my collaborator Sylvie Delacroix has also recently posted about the challenges large language models (and other generative AI) pose for intellectual property (Delacroix, 2023).
Conclusions
Although the capabilities of new generative AIs are surprising society, and being deployed more widely than previous waves of AI with greater accessibility, many of the fundamental policy challenges of AI haven’t changed. There is perhaps a greater urgency in the need for solutions but the nature of the solutions needs to be responsive to the impact of the technology we see. All the initiatives above exploit great agility through building collaborations between social scientists, humanities experts, technical experts and policy experts to give agile advice to policy makers, domain experts and tech experts.
Thanks!
For more information on these subjects and more you might want to check the following resources.
- twitter: @lawrennd
- podcast: The Talking Machines
- newspaper: Guardian Profile Page
- blog: http://inverseprobability.com