AI needs to serve people, science, and society
Abstract
Artificial intelligence offers great promise, but we must ensure it does not deepen inequalities. Today we are setting out our vision for AI@Cam, a new flagship mission at the University of Cambridge.
Henry Ford’s Faster Horse
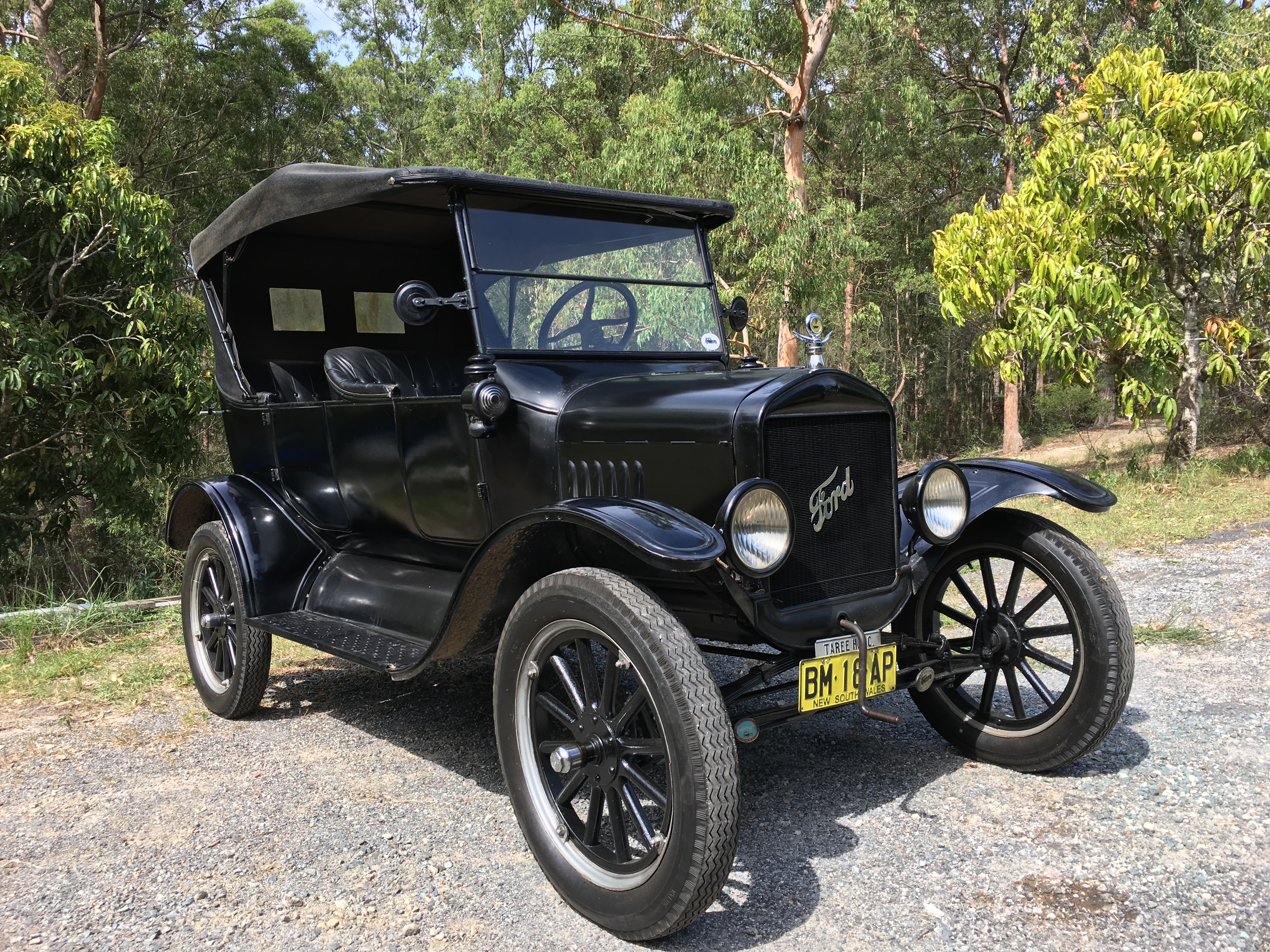
Figure: A 1925 Ford Model T built at Henry Ford’s Highland Park Plant in Dearborn, Michigan. This example now resides in Australia, owned by the founder of FordModelT.net. From https://commons.wikimedia.org/wiki/File:1925_Ford_Model_T_touring.jpg
It’s said that Henry Ford’s customers wanted a “a faster horse”. If Henry Ford was selling us artificial intelligence today, what would the customer call for, “a smarter human”? That’s certainly the picture of machine intelligence we find in science fiction narratives, but the reality of what we’ve developed is much more mundane.
Car engines produce prodigious power from petrol. Machine intelligences deliver decisions derived from data. In both cases the scale of consumption enables a speed of operation that is far beyond the capabilities of their natural counterparts. Unfettered energy consumption has consequences in the form of climate change. Does unbridled data consumption also have consequences for us?
If we devolve decision making to machines, we depend on those machines to accommodate our needs. If we don’t understand how those machines operate, we lose control over our destiny. Our mistake has been to see machine intelligence as a reflection of our intelligence. We cannot understand the smarter human without understanding the human. To understand the machine, we need to better understand ourselves.
In Greek mythology, Panacea was the goddess of the universal remedy. One consequence of the pervasive potential of AI is that it is positioned, like Panacea, as the purveyor of a universal solution. Whether it is overcoming industry’s productivity challenges, or as a salve for strained public sector services, or a remedy for pressing global challenges in sustainable development, AI is presented as an elixir to resolve society’s problems.
In practice, translation of AI technology into practical benefit is not simple. Moreover, a growing body of evidence shows that risks and benefits from AI innovations are unevenly distributed across society.
When carelessly deployed, AI risks exacerbating existing social and economic inequalities.
Revolution
Arguably the information revolution we are experiencing is unprecedented in history. But changes in the way we share information have a long history. Over 5,000 years ago in the city of Uruk, on the banks of the Euphrates, communities which relied on the water to irrigate their corps developed an approach to recording transactions in clay. Eventually the system of recording system became sophisticated enough that their oral histories could be recorded in the form of the first epic: Gilgamesh.
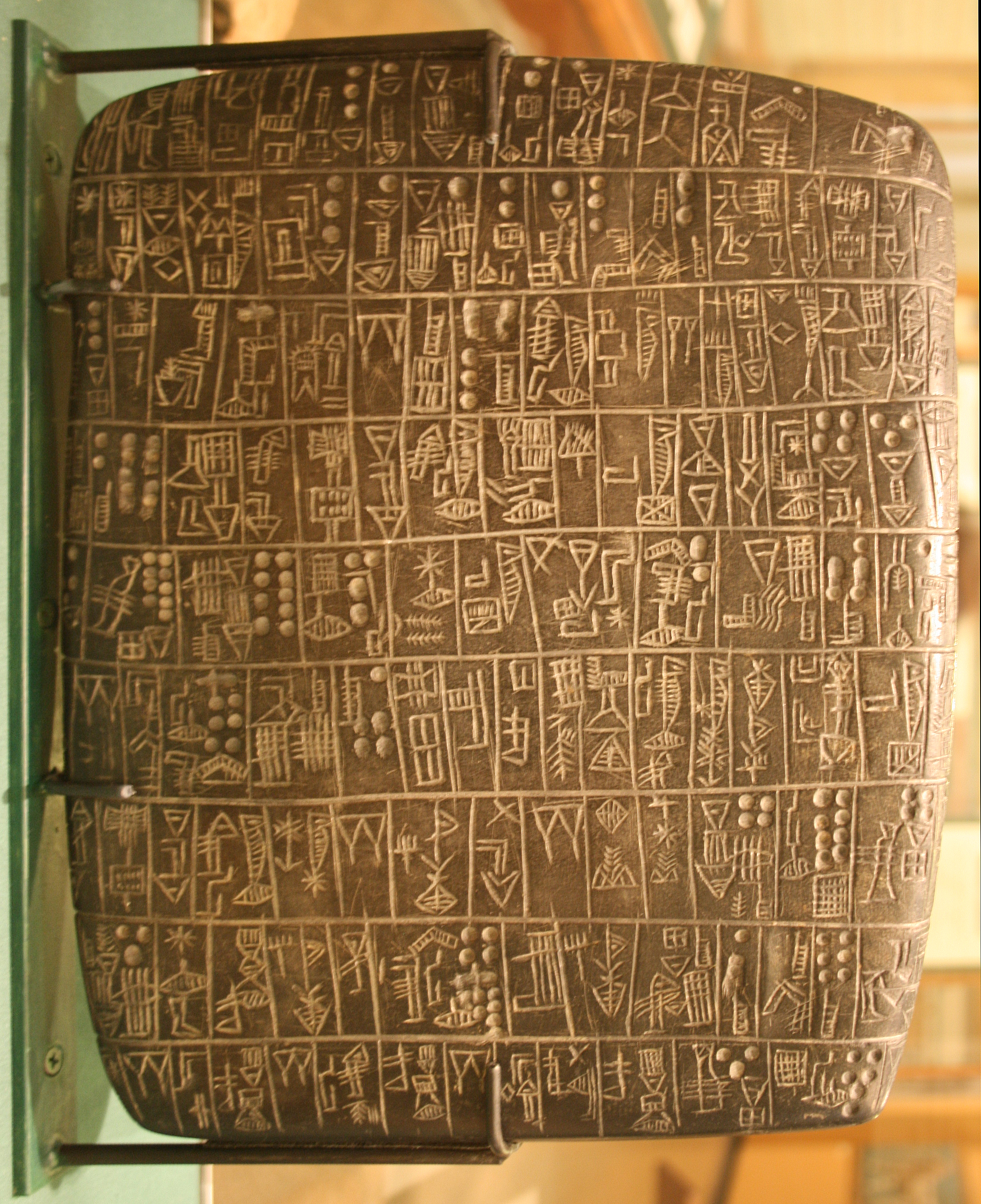
Figure: Chicago Stone, side 2, recording sale of a number of fields, probably from Isin, Early Dynastic Period, c. 2600 BC, black basalt
In some respects today’s revolution is different, because it involves also the creation of stories as well as their curation. But in some fundamental ways we can see what we have produced as another tool for us in the information revolution.
Coin Pusher
Disruption of society is like a coin pusher, it’s those who are already on the edge who are most likely to be effected by disruption.
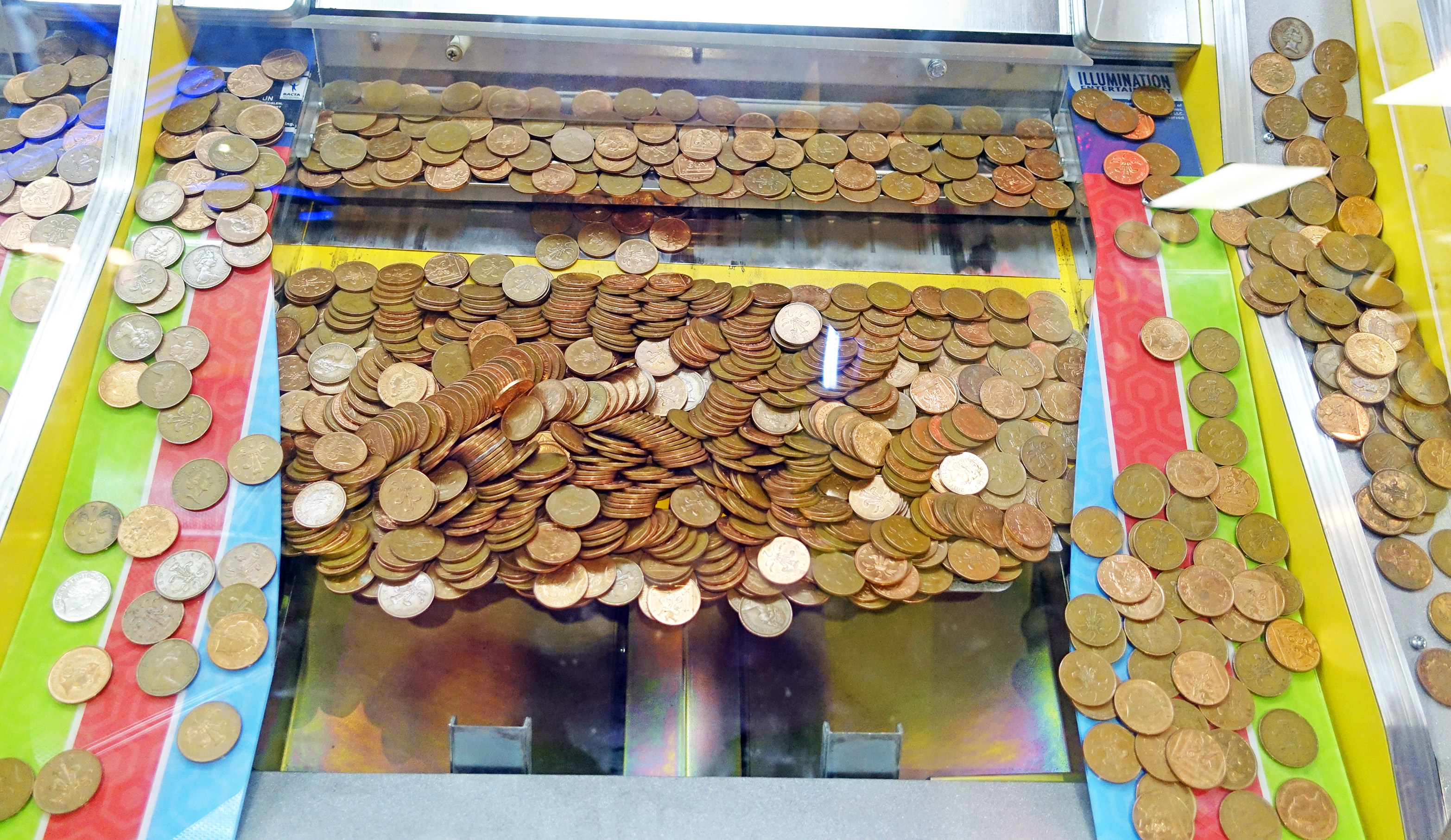
Figure: A coin pusher is a game where coins are dropped into th etop of the machine, and they disrupt those on the existing steps. With any coin drop, many coins move, but it is those on the edge, who are often only indirectly effected, but also most traumatically effected by the change.
One danger of the current hype around ChatGPT is that we are overly focussing on the fact that it seems to have significant effect on professional jobs, people are naturally asking the question “what does it do for my role?”. No doubt, there will be disruption, but the coin pusher hypothesis suggests that that disruption will likely involve movement on the same step. However it is those on the edge already, who are often not working directly in the information economy, who often have less of a voice in the policy conversation who are likely to be most disrupted.
Royal Society Report
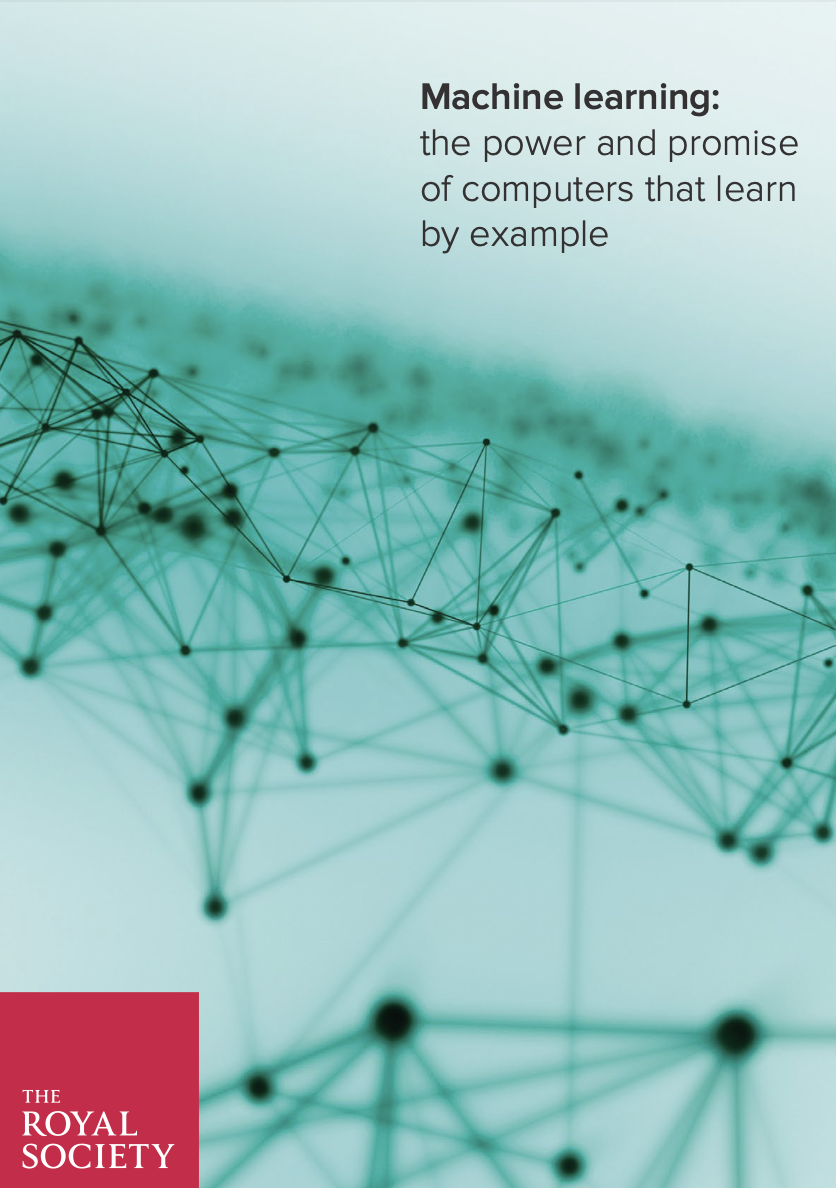
Figure: The Royal Society report on Machine Learning was released on 25th April 2017
A useful reference for state of the art in machine learning is the UK Royal Society Report, Machine Learning: Power and Promise of Computers that Learn by Example.
Public Research
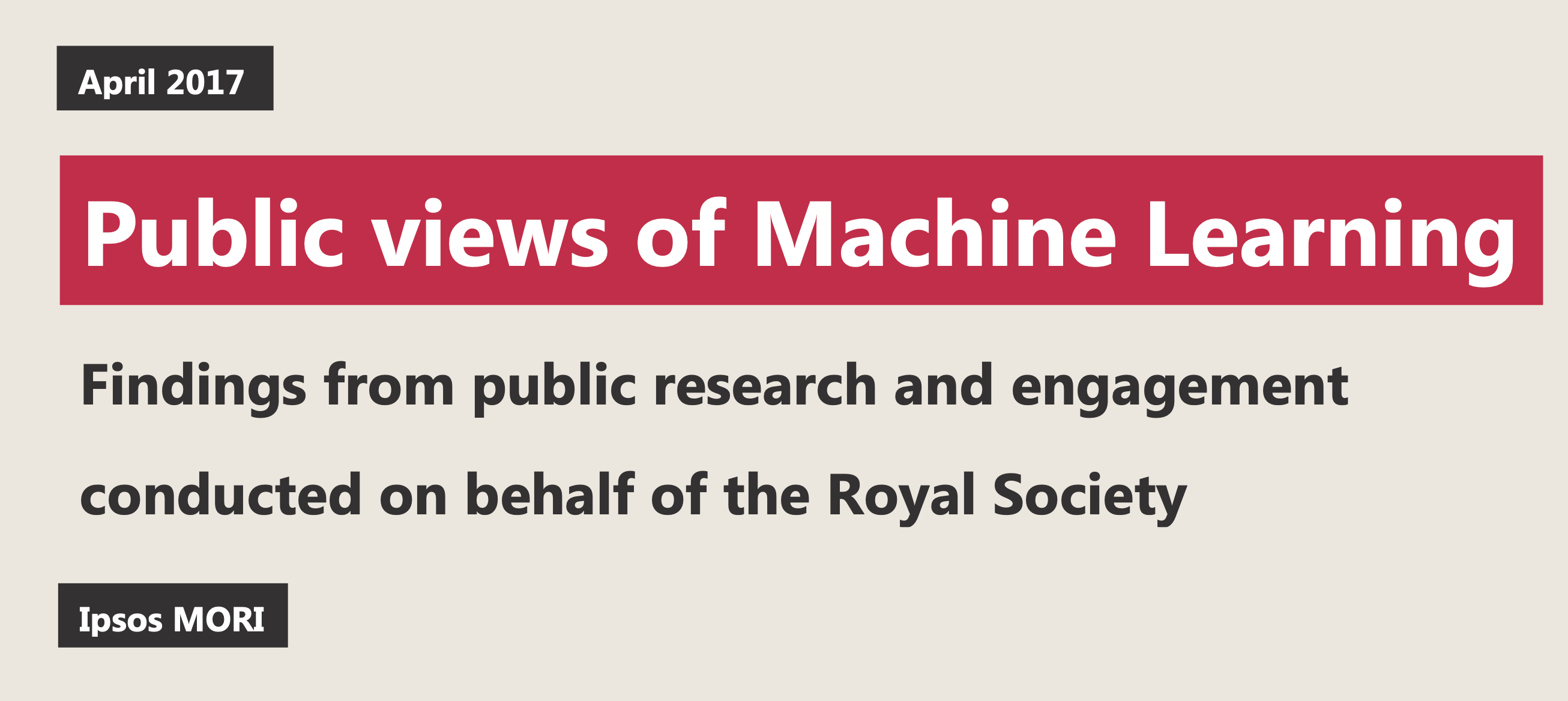
Figure: The Royal Society comissioned public research from Mori as part of the machine learning review.
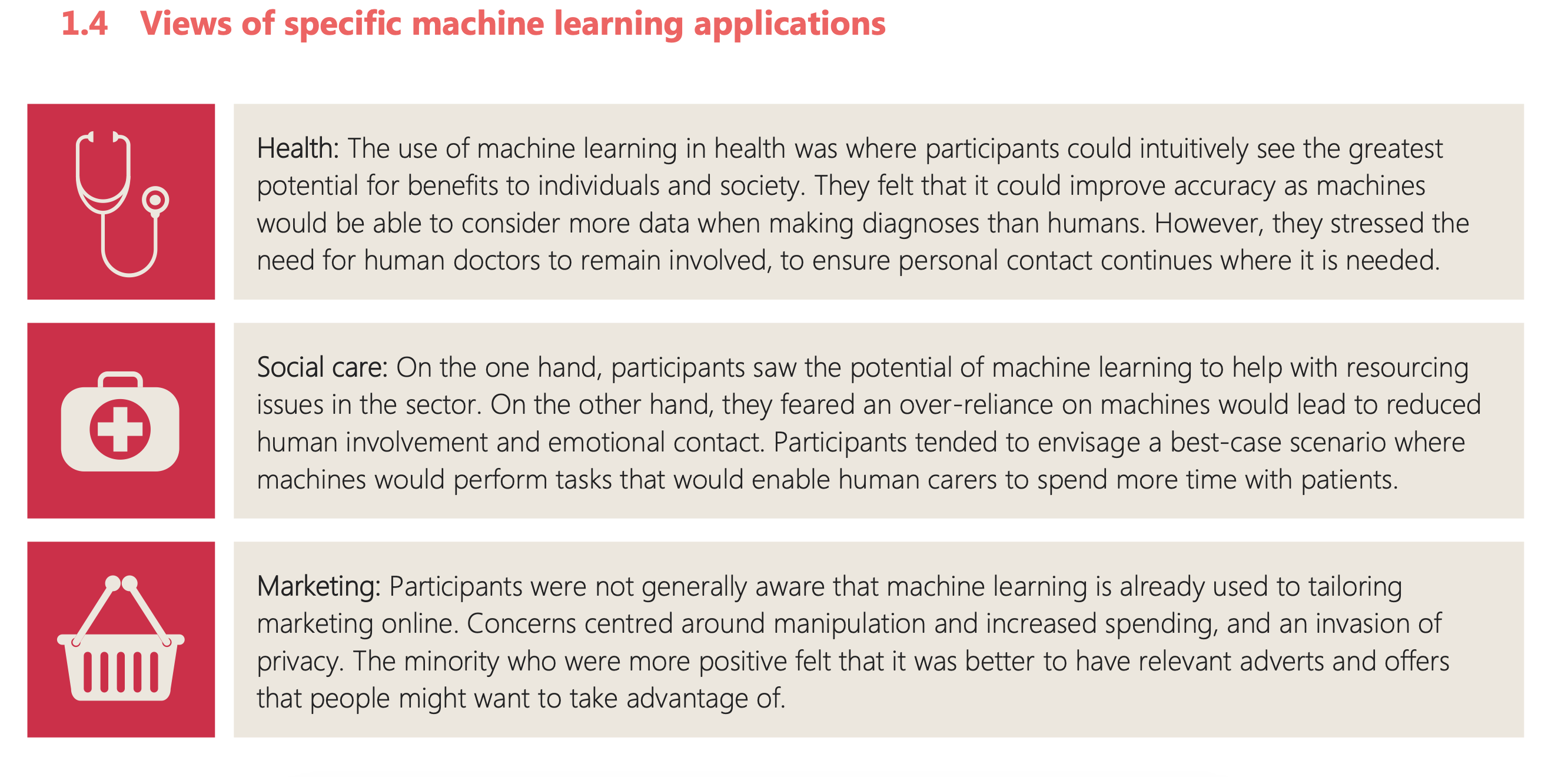
Figure: One of the questions focussed on machine learning applications.
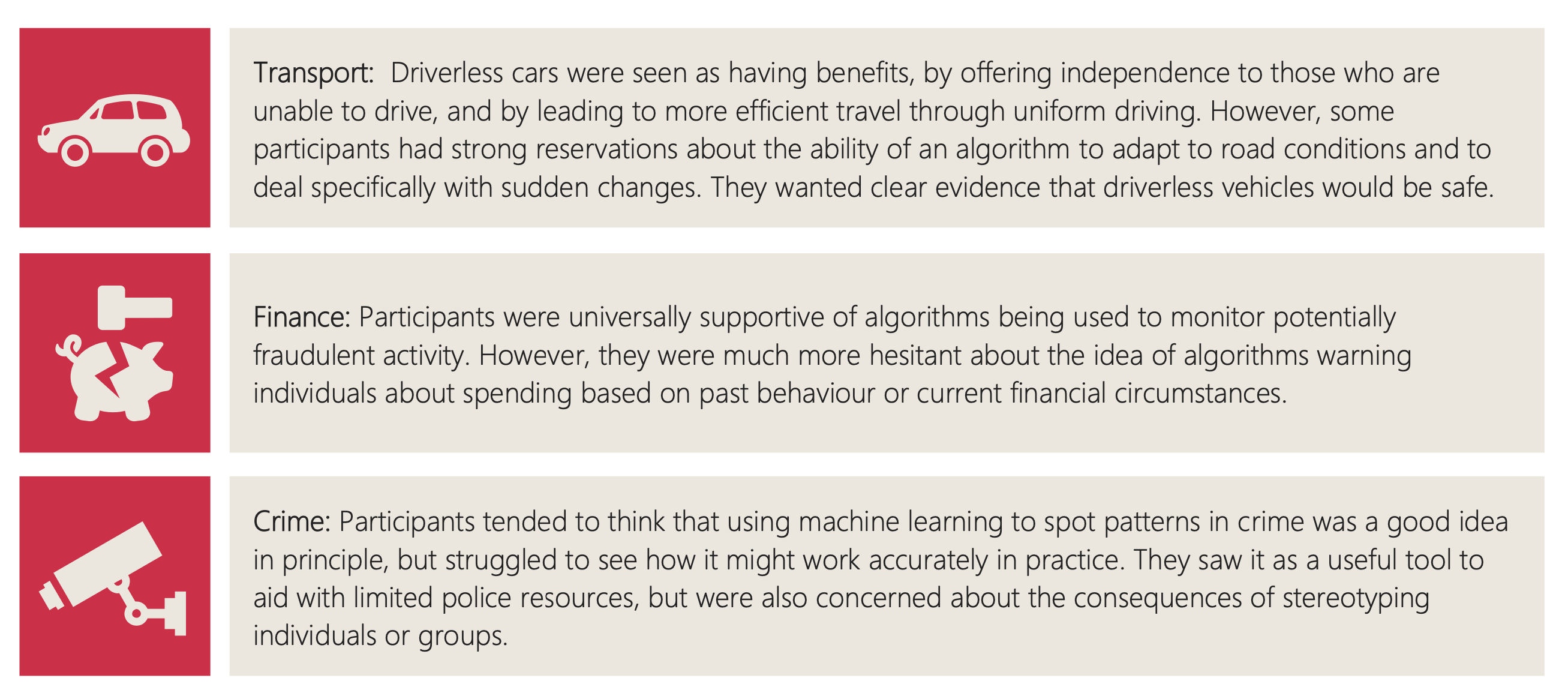
Figure: The public were broadly duspportive of a range of application areas..
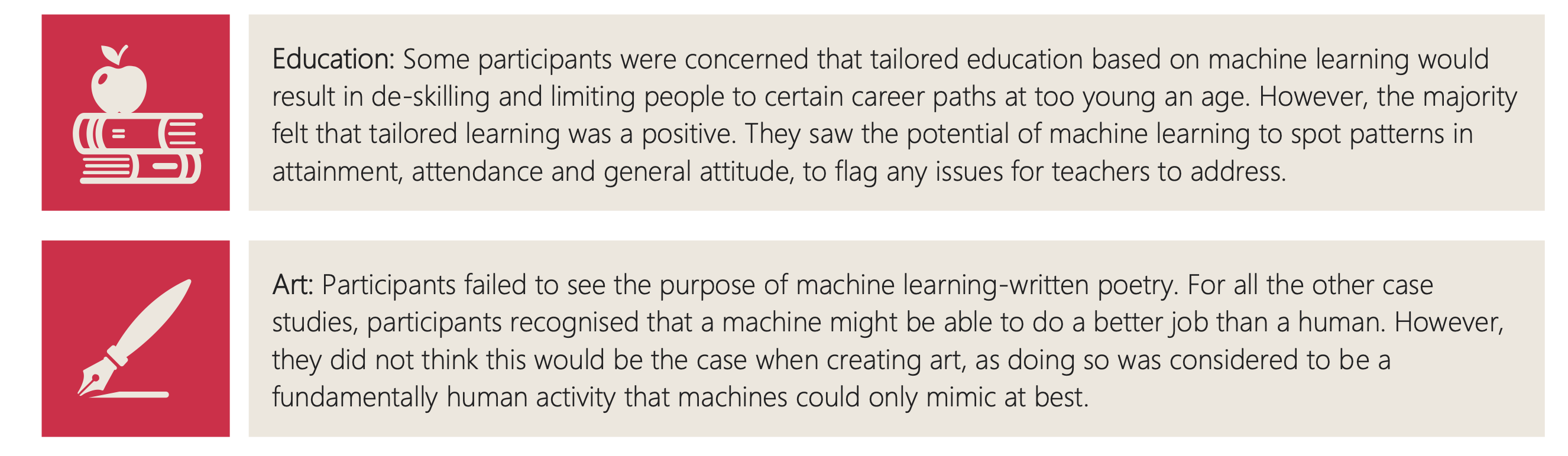
Figure: But they failed to see the point in AI’s that could produce poetry.
Mercutio
It’s ironic that despite the public focus on transport, health, cities, crime and their confusion as to why we might want AI to do art, one area where significant progress has been made is on AIs that can create literature.
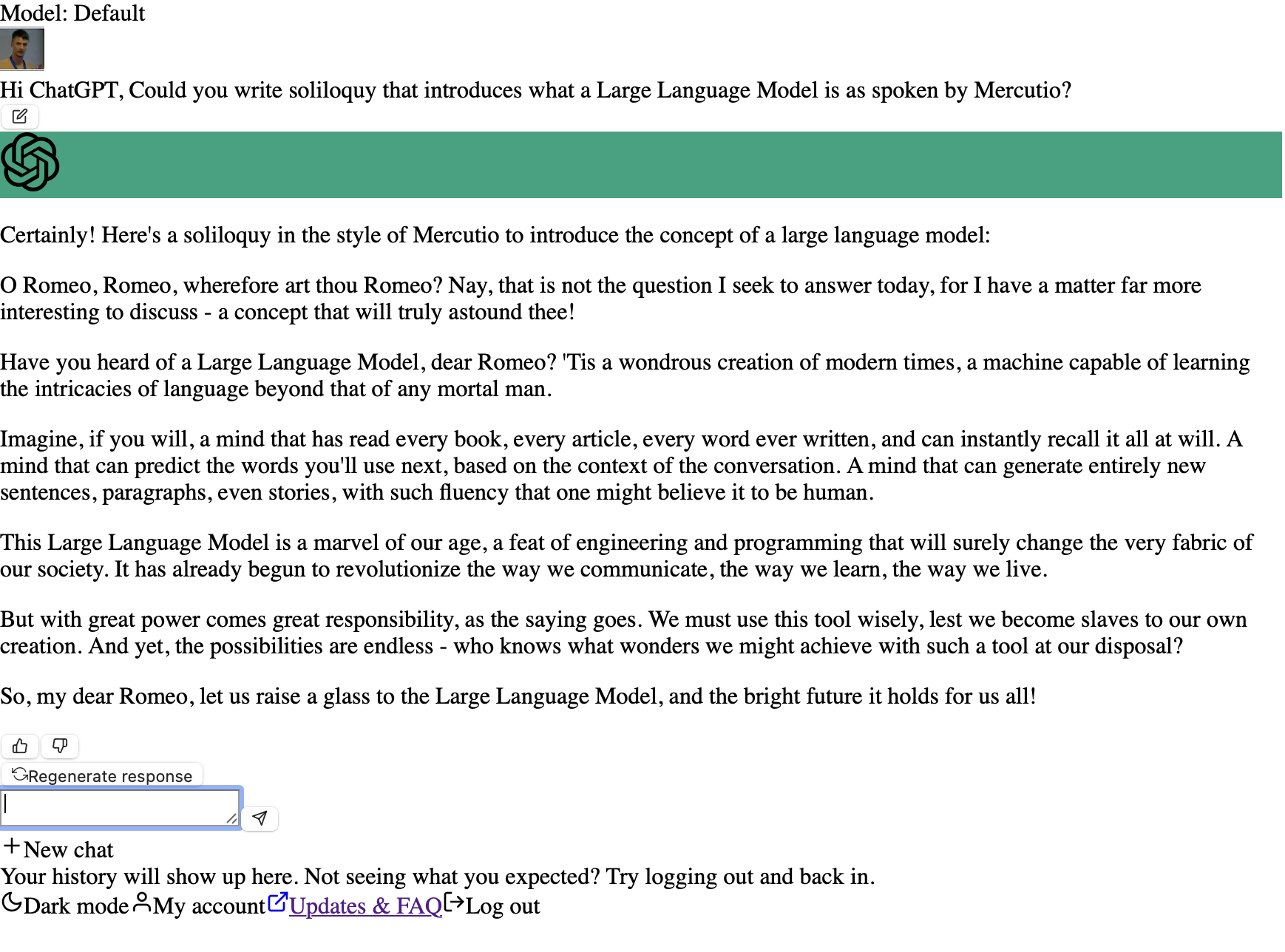
Figure: Despite the public’s desire to see challenges in health, finance, transport, crime solved by machine learning and their skepticism around poetry, one of the problems we have solved seems to be poetry.
User: Hi ChatGPT, Could you write soliloquy that introduces what a Large Language Model is as spoken by Mercutio?
Machine: Certainly! Here’s a soliloquy in the style of Mercutio to introduce the concept of a large language model: O Romeo, Romeo, wherefore art thou Romeo? Nay, that is not the question I seek to answer today, for I have a matter far more interesting to discuss - a concept that will truly astound thee! Have you heard of a Large Language Model, dear Romeo? ’Tis a wondrous creation of modern times, a machine capable of learning the intricacies of language beyond that of any mortal man.
Imagine, if you will, a mind that has read every book, every article, every word ever written, and can instantly recall it all at will. A mind that can predict the words you’ll use next, based on the context of the conversation. A mind that can generate entirely new sentences, paragraphs, even stories, with such fluency that one might believe it to be human. This Large Language Model is a marvel of our age, a feat of engineering and programming that will surely change the very fabric of our society. It has already begun to revolutionize the way we communicate, the way we learn, the way we live.
But with great power comes great responsibility, as the saying goes. We must use this tool wisely, lest we become slaves to our own creation. And yet, the possibilities are endless - who knows what wonders we might achieve with such a tool at our disposal? So, my dear Romeo, let us raise a glass to the Large Language Model, and the bright future it holds for us all!
ChatGPT transcript, 14th March 2023.
Weather
So what’s going on here? One analogy I like to use is with weather forecasting. Historically, before the use of computer driven weather forecasting, we used a process of interpolation to measure the pressure.
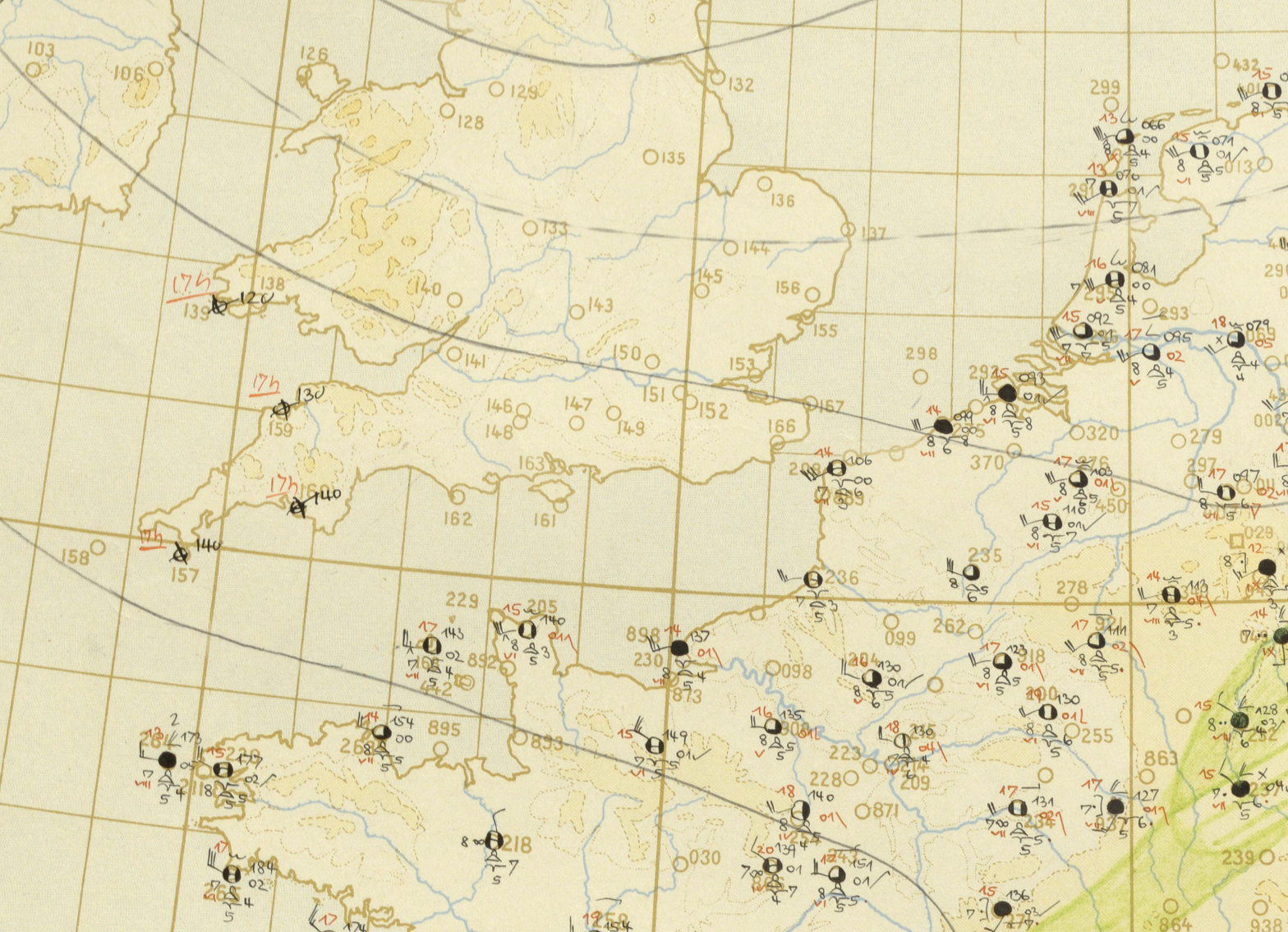
Figure: Forecast from UK Met Office on 5th June 1944. (detail from https://www.metoffice.gov.uk/research/library-and-archive/archive-hidden-treasures/d-day)
This was problematic for German forces in the Second World War because they had no ability to predict the weather when it was coming in from across the UK. Conversely, the UK had a number of weather stations in the UK, and some information (perhaps from spies or Enigma decrypts) about weather on the mainland.
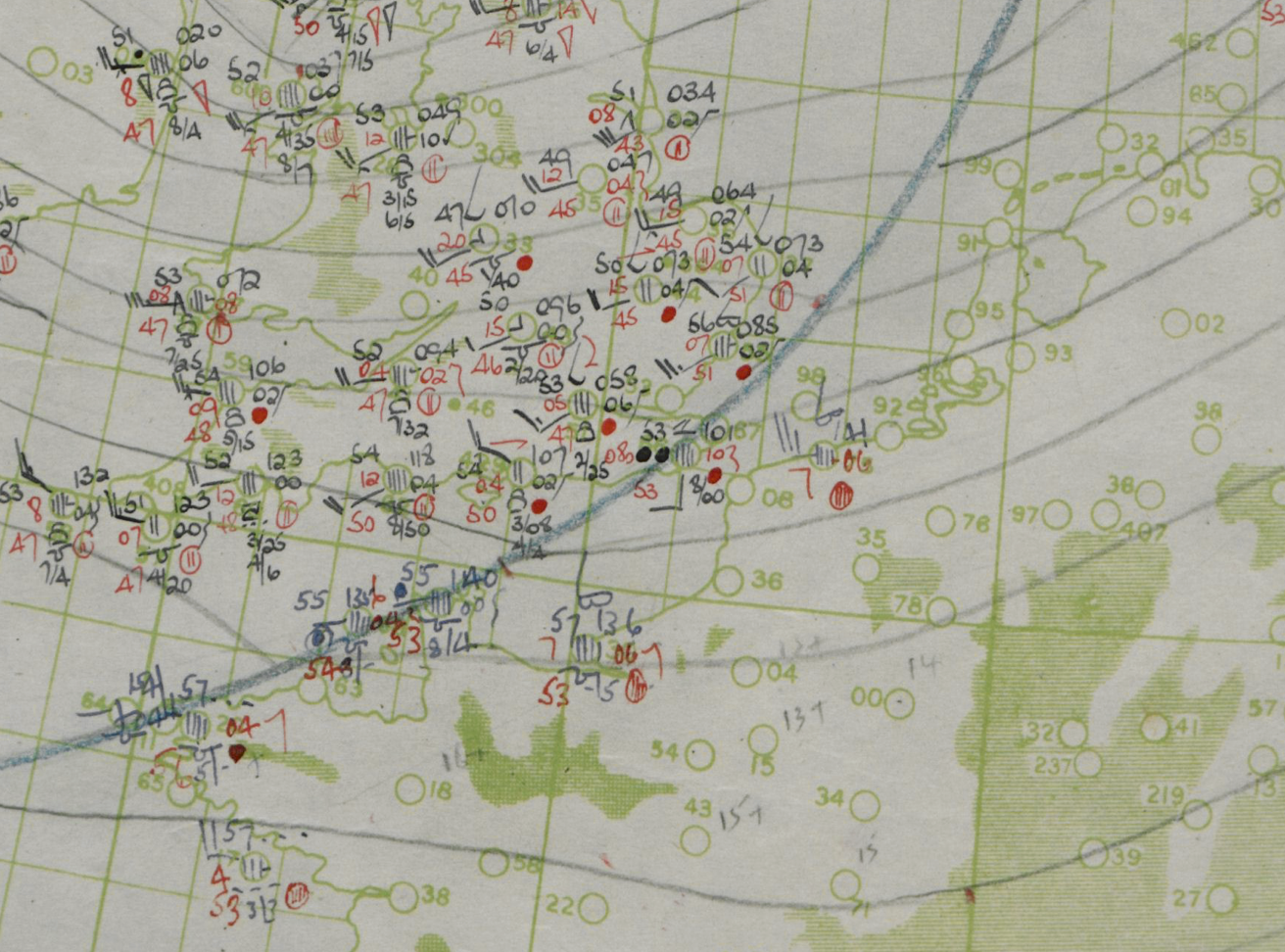
Figure: Forecast from Deutscher Wetterdienst on 5th June 1944. (detail from https://www.metoffice.gov.uk/research/library-and-archive/archive-hidden-treasures/d-day). Note the lack of measurements within the UK. THis is the direction that weather was coming from so the locaiton of weather fronts (and associated storms) was harder for the Deutscher Wetterdienst to predict than the Met Office.
This meant that more accurate forecasts were possible for D-Day for the Allies than for the defending forces. As a result, on the morning that Eisenhower invated, Rommel was back in Germany attending his wife’s 50th birthday party.
A Question of Trust
In Baroness Onora O’Neill’s Reeith Lectures from 2002, she raises the challenge of trust. There are many aspects to her arcuments, but one of the key points she makes is that we cannot trust without the notion of duty. O’Neill is bemoaning the substitution of duty with process. The idea is that processes and transparency are supposed to hold us to account by measuring outcomes. But these processes themselves overwhelm decision makers and undermine their professional duty to deliver the right outcome.
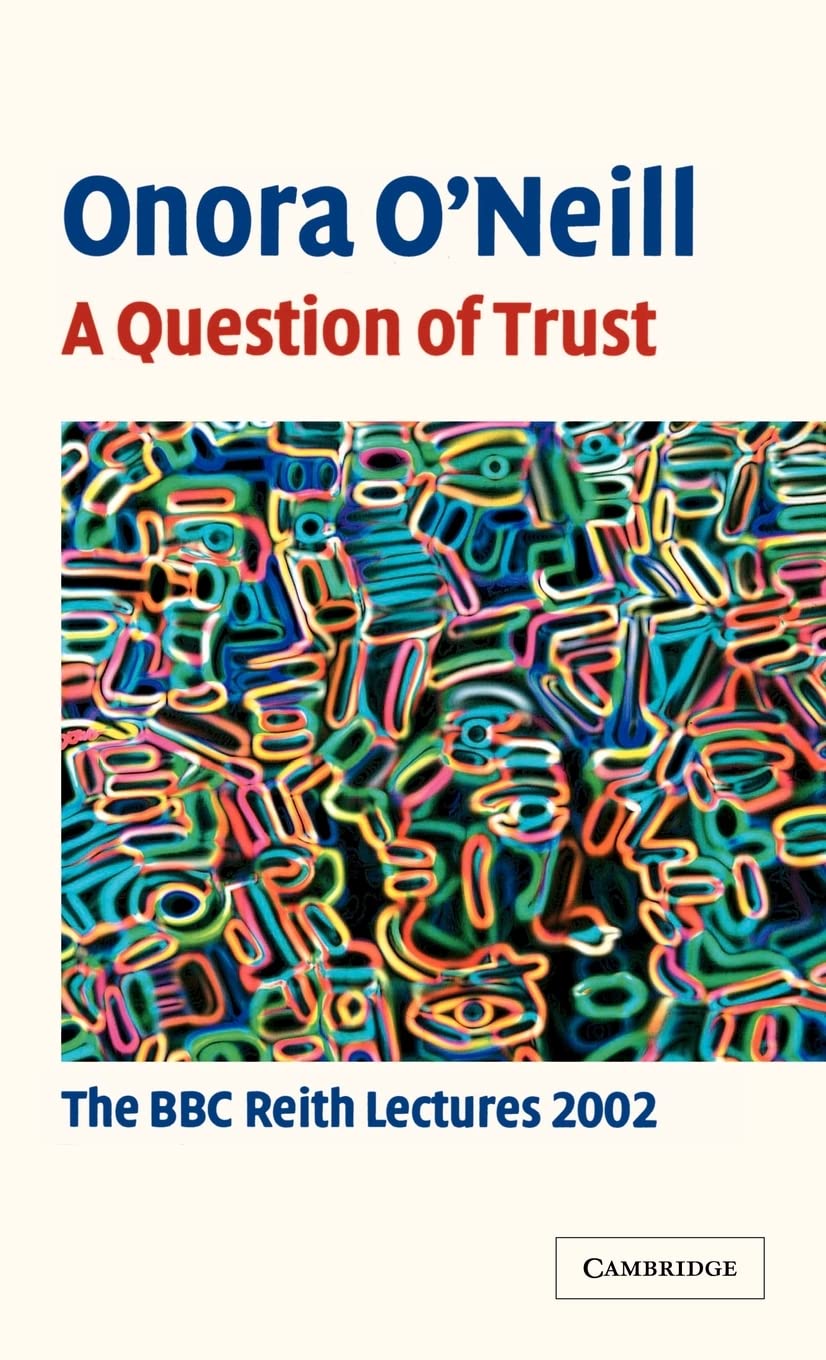
Figure: A Question of Trust by Onora O’Neil which examines the nature of trust and its role in society.
O’Neill is speaking in 2002, in the early days of the internet and before social media. Much of her thoughts are even more relevant for today than they were when she spoke. This is because the increased availability of information and machine driven decision-making makes the mistaken premise, that process is an adequate substitute for duty, more apparently plausible. But this undermines what O’Neill calls “intelligent accountability”, which is not accounting by the numbers, but through professional education and institutional safeguards.
NACA Langley
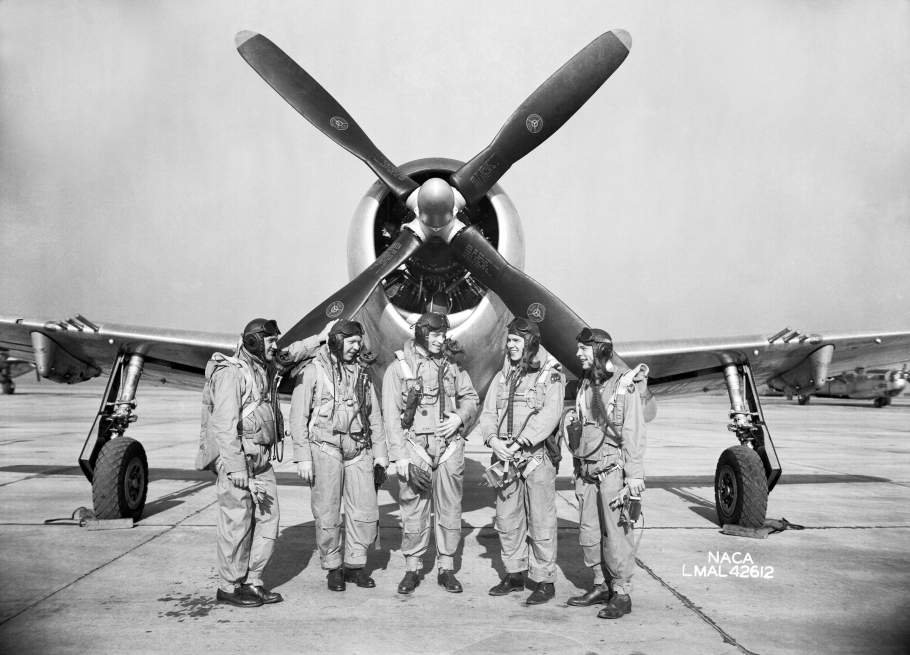
Figure: 1945 photo of the NACA test pilots, from left Mel Gough, Herb Hoover, Jack Reeder, Stefan Cavallo and Bill Gray (photo NASA, NACA LMAL 42612)
The NACA Langley Field proving ground tested US aircraft. Bob Gilruth worked on the flying qualities of aircraft. One of his collaborators suggested that
Hawker Hurricane airplane. A heavily armed fighter airplane noted for its role in the Battle of Britain, the Hurricane’s flying qualities were found to be generally satisfactory. The most notable deficiencies were heavy aileron forces at high speeds and large friction in the controls.
W. Hewitt Phillips1
and
Supermarine Spitfire airplane. A high-performance fighter noted for its role in the Battle of Britain and throughout WW II, the Spitfire had desirably light elevator control forces in maneuvers and near neutral longitudinal stability. Its greatest deficiency from the combat standpoint was heavy aileron forces and sluggish roll response at high speeds.
W. Hewitt Phillips2
Gilruth went beyond the reports of feel to characterise how the plane should respond to different inputs on the control stick. In other words he quantified that feel of the plane.
Innovating to serve science and society requires a pipeline of interventions. As well as advances in the technical capabilities of AI technologies, engineering knowhow is required to safely deploy and monitor those solutions in practice. Regulatory frameworks need to adapt to ensure trustworthy use of these technologies. Aligning technology development with public interests demands effective stakeholder engagement to bring diverse voices and expertise into technology design.
Building this pipeline will take coordination across research, engineering, policy and practice. It also requires action to address the digital divides that influence who benefits from AI advances. These include digital divides within the socioeconomic strata that need to be overcome – AI must not exacerbate existing equalities or create new ones. In addressing these challenges, we can be hindered by divides that exist between traditional academic disciplines. We need to develop common understanding of the problems and a shared knowledge of possible solutions.
Making AI equitable
AI@Cam is a new flagship University mission that seeks to address these challenges. It recognises that development of safe and effective AI-enabled innovations requires this mix of expertise from across research domains, businesses, policy-makers, civill society, and from affected communities. AI@Cam is setting out a vision for AI-enabled innovation that benefits science, citizens and society.
This vision will be achieved through leveraging the University’s vibrant interdisciplinary research community. AI@Cam will form partnerships between researchers, practitioners, and affected communities that embed equity and inclusion. It will develop new platforms for innovation and knowledge transfer. It will deliver innovative interdisciplinary teaching and learning for students, researchers, and professionals. It will build strong connections between the University and national AI priorities.
The University operates as both an engine of AI-enabled innovation and steward of those innovations.
AI is not a universal remedy. It is a set of tools, techniques and practices that correctly deployed can be leveraged to deliver societal benefit and mitigate social harm.
In that sense AI@Cam’s mission is close in spirit to that of Panacea’s elder sister Hygeia. It is focussed on building and maintaining the hygiene of a robust and equitable AI research ecosystem.
\(p\)-Fairness and \(n\)-Fairness
Again Univesities are to treat each applicant fairly on the basis of ability and promise, but they are supposed also to admit a socially more representative intake.
There’s no guarantee that the process meets the target.
Onora O’Neill A Question of Trust: Called to Account Reith Lectures 2002 O’Neill (2002)]
Figure: We seem to have two different aspects to fairness, which in practice can be in tension.
We’ve outlined \(n\)-fairness and \(p\)-fairness. By \(n\)-fairness we mean the sort of considerations that are associated with substantive equality of opportunity vs formal equality of opportunity. Formal equality of community is related to \(p\)-fairness. This is sometimes called procedural fairness and we might think of it as a performative form of fairness. It’s about clarity of rules, for example as applied in sport. \(n\)-Fairness is more nuanced. It’s a reflection of society’s normative judgment about how individuals may have been disadvantaged, e.g. due to their upbringing.
The important point here is that these forms of fairness are in tension. Good procedural fairness needs to be clear and understandable. It should be clear to everyone what the rules are, they shouldn’t be obscured by jargon or overly subtle concepts. \(p\)-Fairness should not be easily undermined by adversaries, it should be difficult to “cheat” good \(p\)-fairness. However, \(n\)-fairness requires nuance, understanding of the human condition, where we came from and how different individuals in our society have been advantaged or disadvantaged in their upbringing and their access to opportunity.
Pure \(n\)-fairness and pure \(p\)-fairness both have the feeling of dystopias. In practice, any decision making system needs to balance the two. The correct point of operation will depend on the context of the decision. Consider fair rules of a game of football, against fair distribution of social benefit. It is unlikely that there is ever an objectively correct balance between the two for any given context. Different individuals will favour \(p\) vs \(n\) according to their personal values.
Given the tension between the two forms of fairness, with \(p\) fairness requiring simple rules that are understandable by all, and \(n\) fairness requiring nuance and subtlety, how do we resolve this tension in practice?
Normally in human systems, significant decisions involve trained professionals. For example, judges, or accountants or doctors.
Training a professional involves lifting their “reflexive” response to a situation with “reflective” thinking about the consequences of their decision that rely not just on the professional’s expertise, but also their knowledge of what it is to be a human.
This marvellous resolution exploits the fact that while humans are increadibly complicated nuanced entities, other humans have an intuitive ability to understand their motivations and values. So the human is a complex entity that seems simple to other humans.
The Great AI Fallacy
There is a lot of variation in the use of the term artificial intelligence. I’m sometimes asked to define it, but depending on whether you’re speaking to a member of the public, a fellow machine learning researcher, or someone from the business community, the sense of the term differs.
However, underlying its use I’ve detected one disturbing trend. A trend I’m beginining to think of as “The Great AI Fallacy”.
The fallacy is associated with an implicit promise that is embedded in many statements about Artificial Intelligence. Artificial Intelligence, as it currently exists, is merely a form of automated decision making. The implicit promise of Artificial Intelligence is that it will be the first wave of automation where the machine adapts to the human, rather than the human adapting to the machine.
How else can we explain the suspension of sensible business judgment that is accompanying the hype surrounding AI?
This fallacy is particularly pernicious because there are serious benefits to society in deploying this new wave of data-driven automated decision making. But the AI Fallacy is causing us to suspend our calibrated skepticism that is needed to deploy these systems safely and efficiently.
The problem is compounded because many of the techniques that we’re speaking of were originally developed in academic laboratories in isolation from real-world deployment.
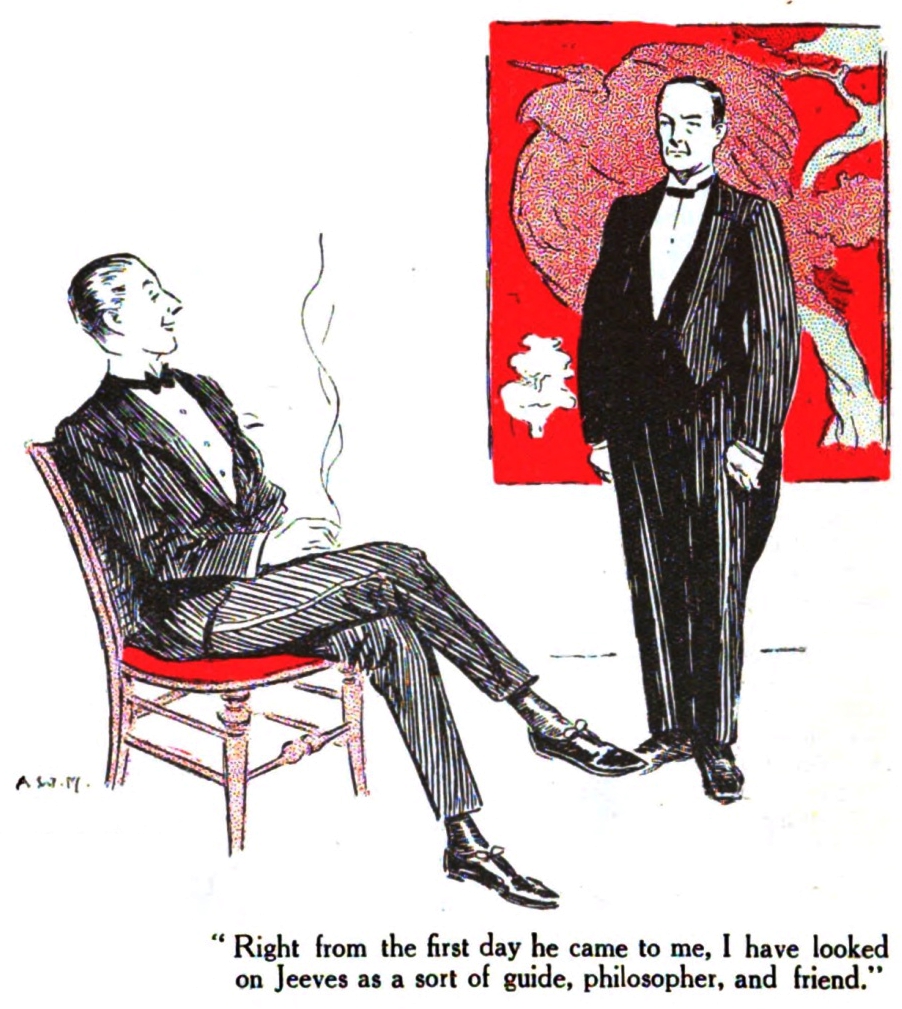
Figure: We seem to have fallen for a perspective on AI that suggests it will adapt to our schedule, rather in the manner of a 1930s manservant.
The Accelerate Programme
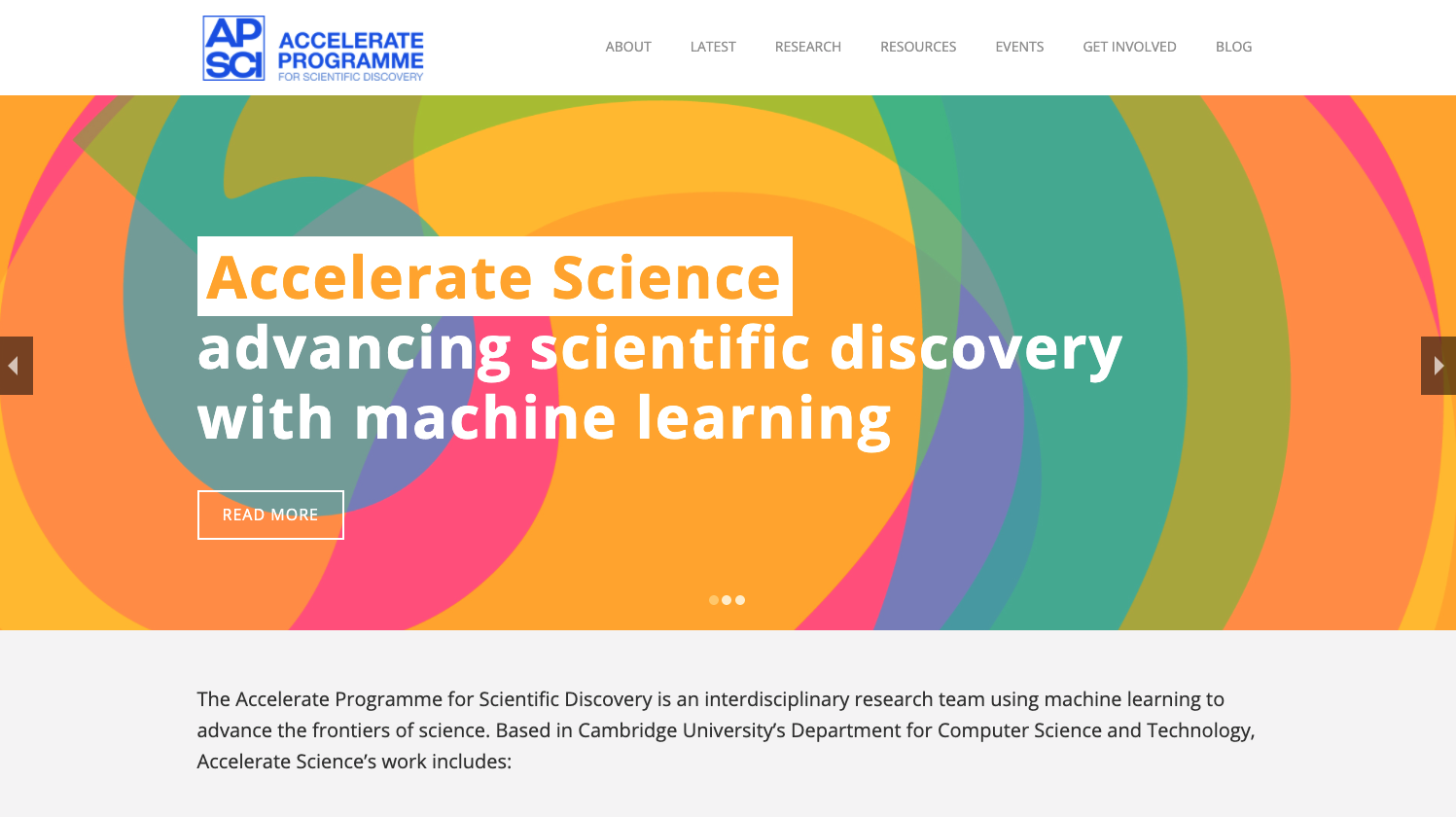
Figure: The Accelerate Programme for Scientific Discovery covers research, education and training, engagement. Our aim is to bring about a step change in scientific discovery through AI. http://acceleratescience.github.io
We’re now in a new phase of the development of computing, with rapid advances in machine learning. But we see some of the same issues – researchers across disciplines hope to make use of machine learning, but need access to skills and tools to do so, while the field machine learning itself will need to develop new methods to tackle some complex, ‘real world’ problems.
It is with these challenges in mind that the Computer Lab has started the Accelerate Programme for Scientific Discovery. This new Programme is seeking to support researchers across the University to develop the skills they need to be able to use machine learning and AI in their research.
To do this, the Programme is developing three areas of activity:
Research: we’re developing a research agenda that develops and applies cutting edge machine learning methods to scientific challenges, with three Accelerate Research fellows working directly on issues relating to computational biology, psychiatry, and string theory. While we’re concentrating on STEM subjects for now, in the longer term our ambition is to build links with the social sciences and humanities.
Teaching and learning: building on the teaching activities already delivered through University courses, we’re creating a pipeline of learning opportunities to help PhD students and postdocs better understand how to use data science and machine learning in their work. Our programme with Spark is one element of this, and we’ll be announcing further activities soon.
Engagement: we hope that Accelerate will help build a community of researchers working across the University at the interface on machine learning and the sciences, helping to share best practice and new methods, and support each other in advancing their research. Over the coming years, we’ll be running a variety of events and activities in support of this, and would welcome your ideas about what might be most useful.
Personal Data Trusts
The machine learning solutions we are dependent on to drive automated decision making are dependent on data. But with regard to personal data there are important issues of privacy. Data sharing brings benefits, but also exposes our digital selves. From the use of social media data for targeted advertising to influence us, to the use of genetic data to identify criminals, or natural family members. Control of our virtual selves maps on to control of our actual selves.
The feudal system that is implied by current data protection legislation has significant power asymmetries at its heart, in that the data controller has a duty of care over the data subject, but the data subject may only discover failings in that duty of care when it’s too late. Data controllers also may have conflicting motivations, and often their primary motivation is not towards the data-subject, but that is a consideration in their wider agenda.
Personal Data Trusts (Delacroix and Lawrence, 2018; Edwards, 2004; Lawrence, 2016) are a potential solution to this problem. Inspired by land societies that formed in the 19th century to bring democratic representation to the growing middle classes. A land society was a mutual organization where resources were pooled for the common good.
A Personal Data Trust would be a legal entity where the trustees’ responsibility was entirely to the members of the trust. So the motivation of the data-controllers is aligned only with the data-subjects. How data is handled would be subject to the terms under which the trust was convened. The success of an individual trust would be contingent on it satisfying its members with appropriate balancing of individual privacy with the benefits of data sharing.
Formation of Data Trusts became the number one recommendation of the Hall-Presenti report on AI, but unfortunately, the term was confounded with more general approaches to data sharing that don’t necessarily involve fiduciary responsibilities or personal data rights. It seems clear that we need to better characterize the data sharing landscape as well as propose mechanisms for tackling specific issues in data sharing.
It feels important to have a diversity of approaches, and yet it feels important that any individual trust would be large enough to be taken seriously in representing the views of its members in wider negotiations.
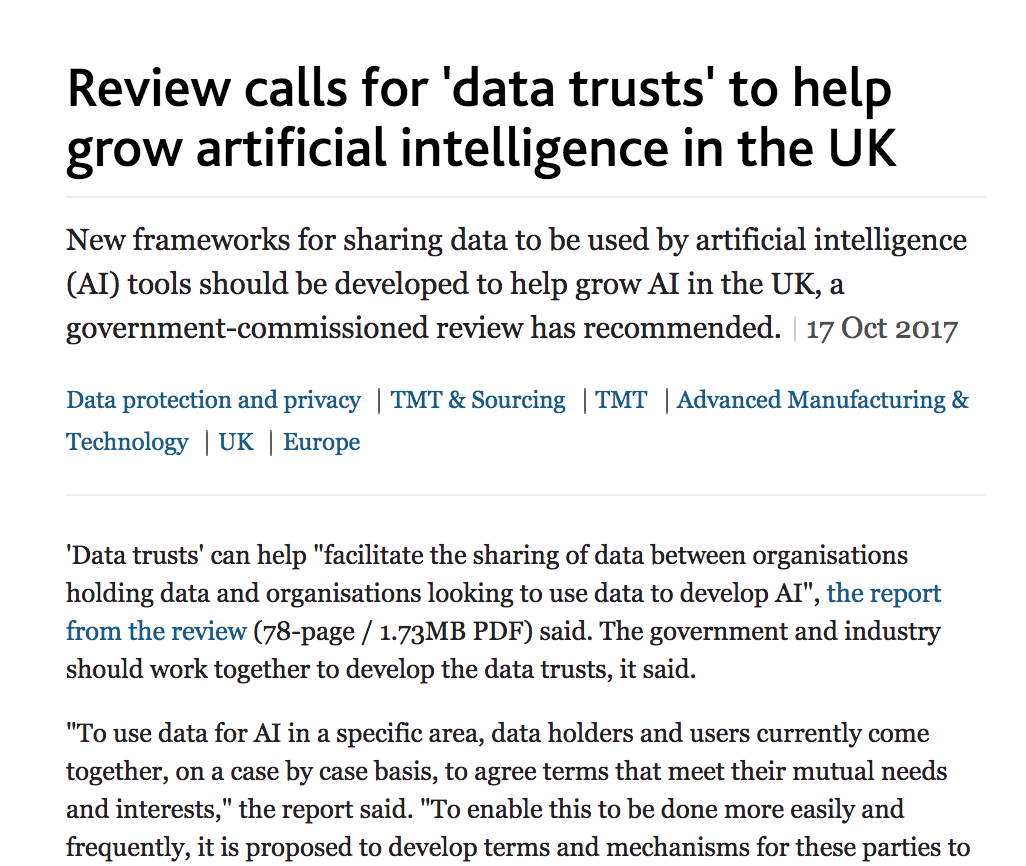
Figure: Data Trusts were the first recommendation of the Hall-Presenti Report. Unfortunately, since then the role of data trusts vs other data sharing mechanisms in the UK has been somewhat confused.
See Guardian article on Digital Oligarchies and Guardian article on Information Feudalism.
Data Trusts Initiative
The Data Trusts Initiative, funded by the Patrick J. McGovern Foundation is supporting three pilot projects that consider how bottom-up empowerment can redress the imbalance associated with the digital oligarchy.
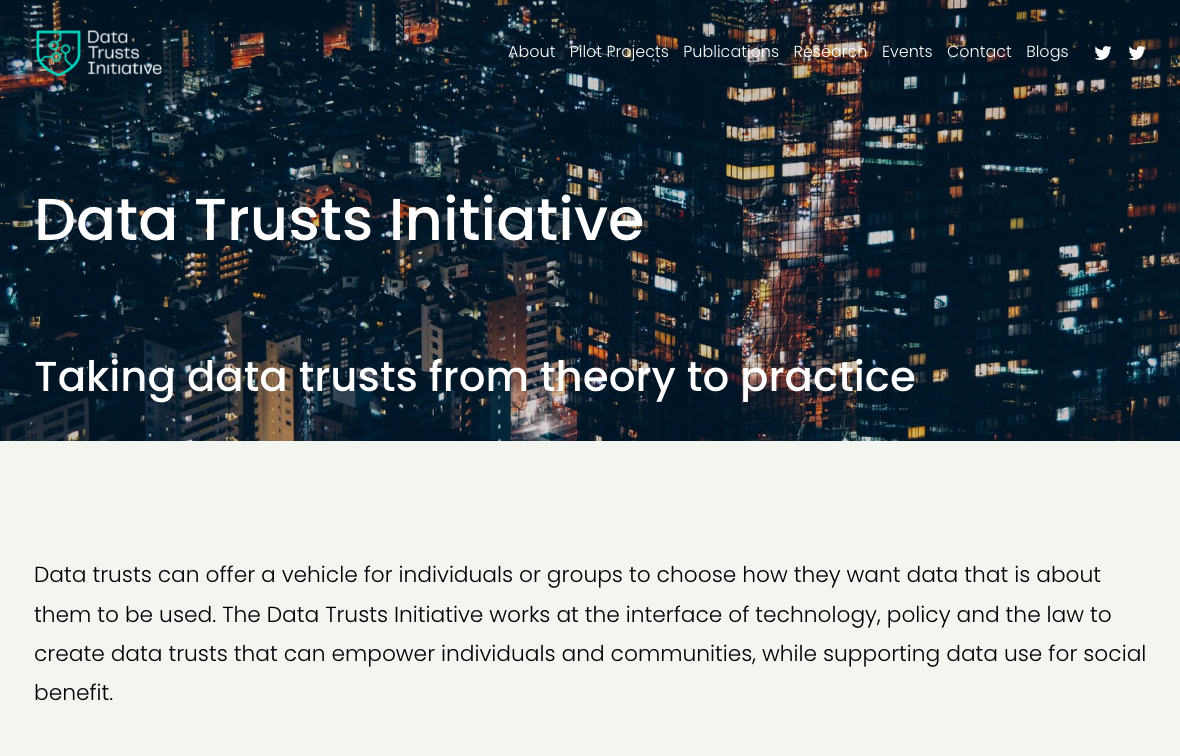
Figure: The Data Trusts Initiative (http://datatrusts.uk) hosts blog posts helping build understanding of data trusts and supports research and pilot projects.
Progress So Far
In its first 18 months of operation, the Initiative has:
Convened over 200 leading data ethics researchers and practitioners;
Funded 7 new research projects tackling knowledge gaps in data trust theory and practice;
Supported 3 real-world data trust pilot projects establishing new data stewardship mechanisms.
AI@Cam
Finally, we are working across the University to empower the diversity ofexpertise and capability we have to focus on these broad societal problems. We will recently launched AI@Cam with a vision document that outlines these challenges for the University.
Thanks!
For more information on these subjects and more you might want to check the following resources.
- twitter: @lawrennd
- podcast: The Talking Machines
- newspaper: Guardian Profile Page
- blog: http://inverseprobability.com