Human-Machine Collaboration in the AI Era
Abstract
The latest wave of machine learning technology doesn’t just represent advancements in algorithms and capabilities - it represents a fundamental shift in how humans and machines can interact. For decades, we’ve adapted our workflows and thinking to accommodate what computers could do. Now, we’re entering an era where machines can adapt to us, where natural language interfaces allow humans to express their needs directly, and where the collaboration between humans and machines can reach new heights of productivity and creativity.
This talk explores how financial institutions and investors can leverage these technologies to enhance decision-making, reduce cognitive burden, and create more intuitive workflows that put humans back in control of technology.
The Great AI Fallacy
There is a lot of variation in the use of the term artificial intelligence. I’m sometimes asked to define it, but depending on whether you’re speaking to a member of the public, a fellow machine learning researcher, or someone from the business community, the sense of the term differs.
However, underlying its use I’ve detected one disturbing trend. A trend I’m beginining to think of as “The Great AI Fallacy”.
The fallacy is associated with an implicit promise that is embedded in many statements about Artificial Intelligence. Artificial Intelligence, as it currently exists, is merely a form of automated decision making. The implicit promise of Artificial Intelligence is that it will be the first wave of automation where the machine adapts to the human, rather than the human adapting to the machine.
How else can we explain the suspension of sensible business judgment that is accompanying the hype surrounding AI?
This fallacy is particularly pernicious because there are serious benefits to society in deploying this new wave of data-driven automated decision making. But the AI Fallacy is causing us to suspend our calibrated skepticism that is needed to deploy these systems safely and efficiently.
The problem is compounded because many of the techniques that we’re speaking of were originally developed in academic laboratories in isolation from real-world deployment.
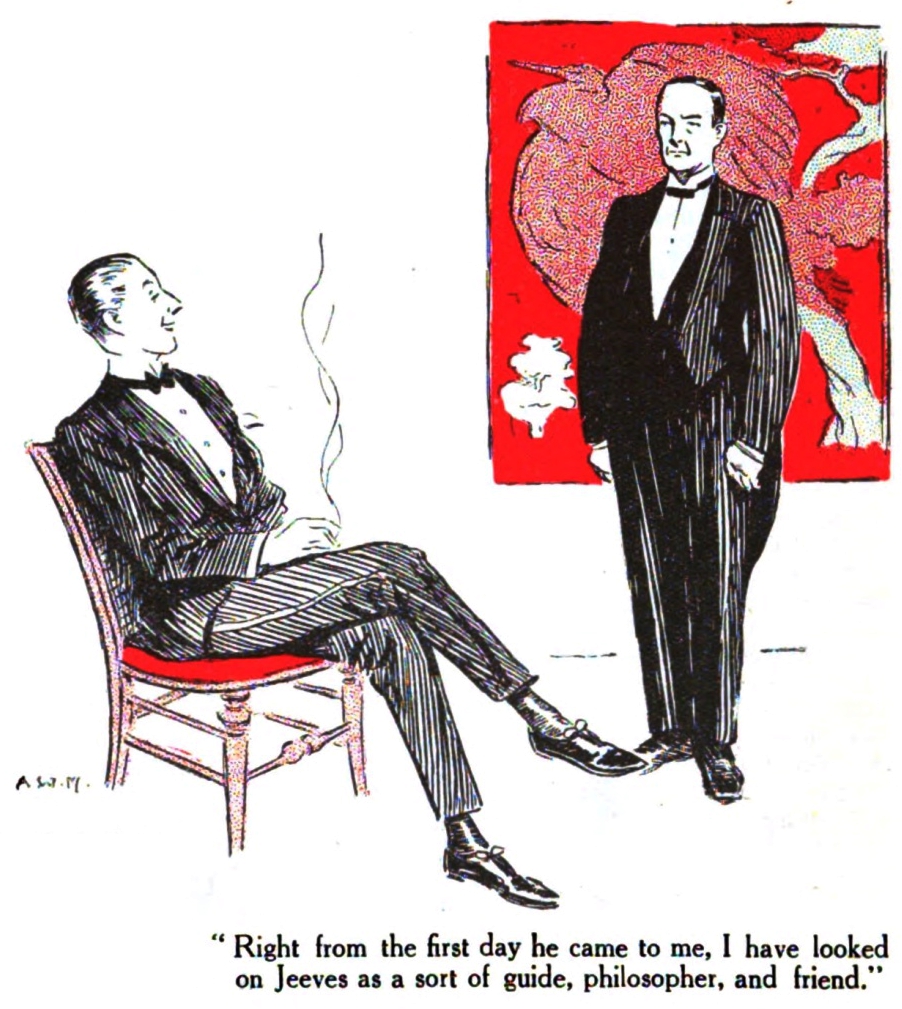
Figure: We seem to have fallen for a perspective on AI that suggests it will adapt to our schedule, rather in the manner of a 1930s manservant.
The Great Digital Inversion
We’re witnessing what I call “The Great Digital Inversion” - a fundamental shift in the relationship between humans and machines.
Since the dawn of computing, humans have had to adapt to machines. We’ve learned programming languages, memorized commands, navigated complex interfaces, and generally molded our thinking to match the rigid logic of computers. The burden of translation has always fallen on humans.
The latest wave of machine learning technology is inverting this relationship. For the first time, we’re creating systems that can understand and adapt to human expressions, preferences, and ways of thinking. Instead of humans learning machine languages, machines are learning human languages. Instead of humans adapting to computer workflows, computers can adapt to human workflows.
This inversion represents perhaps the most significant shift in computing since the graphical user interface. It’s not just about making technology more accessible - it’s about fundamentally changing who bears the cognitive burden in the human-machine relationship.
For decades, our relationship with computers has been fundamentally asymmetric. Humans had to learn the languages of machines, adapt to their limitations, and translate our intentions into forms computers could understand. This created a significant cognitive burden and made technology inaccessible to many.
The early days of computing required specialized knowledge just to interact with machines. Over time, graphical user interfaces made computers more accessible, but still required humans to adapt to the machine’s way of thinking - navigating menus, remembering commands, and organizing work around the computer’s capabilities rather than human thought patterns.
The exciting thing about this new wave of machine learning technology is that it can bring people closer to machines, give humans more control over what machines are doing. Imagine a world where we’re asking the computer to do the thing we need instead of adapting to what it can do.
This represents a fundamental inversion of the traditional human-computer relationship. Instead of humans learning machine languages, machines are now learning human languages. Instead of humans adapting to computer workflows, computers can adapt to human workflows.
Computer Conversations
Figure: Conversation relies on internal models of other individuals.
Figure: Misunderstanding of context and who we are talking to leads to arguments.
Similarly, we find it difficult to comprehend how computers are making decisions. Because they do so with more data than we can possibly imagine.
In many respects, this is not a problem, it’s a good thing. Computers and us are good at different things. But when we interact with a computer, when it acts in a different way to us, we need to remember why.
Just as the first step to getting along with other humans is understanding other humans, so it needs to be with getting along with our computers.
Embodiment factors explain why, at the same time, computers are so impressive in simulating our weather, but so poor at predicting our moods. Our complexity is greater than that of our weather, and each of us is tuned to read and respond to one another.
Their intelligence is different. It is based on very large quantities of data that we cannot absorb. Our computers don’t have a complex internal model of who we are. They don’t understand the human condition. They are not tuned to respond to us as we are to each other.
Embodiment factors encapsulate a profound thing about the nature of humans. Our locked in intelligence means that we are striving to communicate, so we put a lot of thought into what we’re communicating with. And if we’re communicating with something complex, we naturally anthropomorphize them.
We give our dogs, our cats, and our cars human motivations. We do the same with our computers. We anthropomorphize them. We assume that they have the same objectives as us and the same constraints. They don’t.
This means, that when we worry about artificial intelligence, we worry about the wrong things. We fear computers that behave like more powerful versions of ourselves that will struggle to outcompete us.
In reality, the challenge is that our computers cannot be human enough. They cannot understand us with the depth we understand one another. They drop below our cognitive radar and operate outside our mental models.
The real danger is that computers don’t anthropomorphize. They’ll make decisions in isolation from us without our supervision because they can’t communicate truly and deeply with us.
Applications in Finance and Investment
In the financial sector, this inversion creates enormous opportunities:
- Decision support systems that explain their reasoning in natural language, allowing financial analysts to have a dialog with their data
- Investment research that can be queried conversationally, bringing insights to the surface faster
- Risk assessment systems that provide explanations anyone can understand, not just quants
- Client-facing tools that adapt to different levels of financial literacy and provide personalized guidance
The key shift is that financial professionals no longer need to become pseudo-programmers to extract value from their technology systems. The systems can meet them where they are.
Consider how this transformation might play out in wealth management:
Currently, advisors often spend significant time navigating complex systems to generate insights and recommendations. They must translate client needs into technical queries and then translate system outputs back into client-relevant recommendations.
With conversational AI systems, advisors could simply express client needs directly: “Show me investment options for a risk-averse client concerned about inflation who wants to retire in 15 years.” The system could generate appropriate recommendations, explain its reasoning, and allow the advisor to refine the approach through natural dialogue.
This shift allows advisors to spend more time on relationship-building and complex client needs, while the technology adapts to them rather than the other way around.
Embodiment Factors
bits/min | billions | 2,000 |
billion calculations/s |
~100 | a billion |
embodiment | 20 minutes | 5 billion years |
Figure: Embodiment factors are the ratio between our ability to compute and our ability to communicate. Relative to the machine we are also locked in. In the table we represent embodiment as the length of time it would take to communicate one second’s worth of computation. For computers it is a matter of minutes, but for a human, it is a matter of thousands of millions of years. See also “Living Together: Mind and Machine Intelligence” Lawrence (2017)
There is a fundamental limit placed on our intelligence based on our ability to communicate. Claude Shannon founded the field of information theory. The clever part of this theory is it allows us to separate our measurement of information from what the information pertains to.1
Shannon measured information in bits. One bit of information is the amount of information I pass to you when I give you the result of a coin toss. Shannon was also interested in the amount of information in the English language. He estimated that on average a word in the English language contains 12 bits of information.
Given typical speaking rates, that gives us an estimate of our ability to communicate of around 100 bits per second (Reed and Durlach, 1998). Computers on the other hand can communicate much more rapidly. Current wired network speeds are around a billion bits per second, ten million times faster.
When it comes to compute though, our best estimates indicate our computers are slower. A typical modern computer can process make around 100 billion floating-point operations per second, each floating-point operation involves a 64 bit number. So the computer is processing around 6,400 billion bits per second.
It’s difficult to get similar estimates for humans, but by some estimates the amount of compute we would require to simulate a human brain is equivalent to that in the UK’s fastest computer (Ananthanarayanan et al., 2009), the MET office machine in Exeter, which in 2018 ranked as the 11th fastest computer in the world. That machine simulates the world’s weather each morning, and then simulates the world’s climate in the afternoon. It is a 16-petaflop machine, processing around 1,000 trillion bits per second.
See Lawrence (2024) embodiment factor p. 13, 29, 35, 79, 87, 105, 197, 216-217, 249, 269, 353, 369.
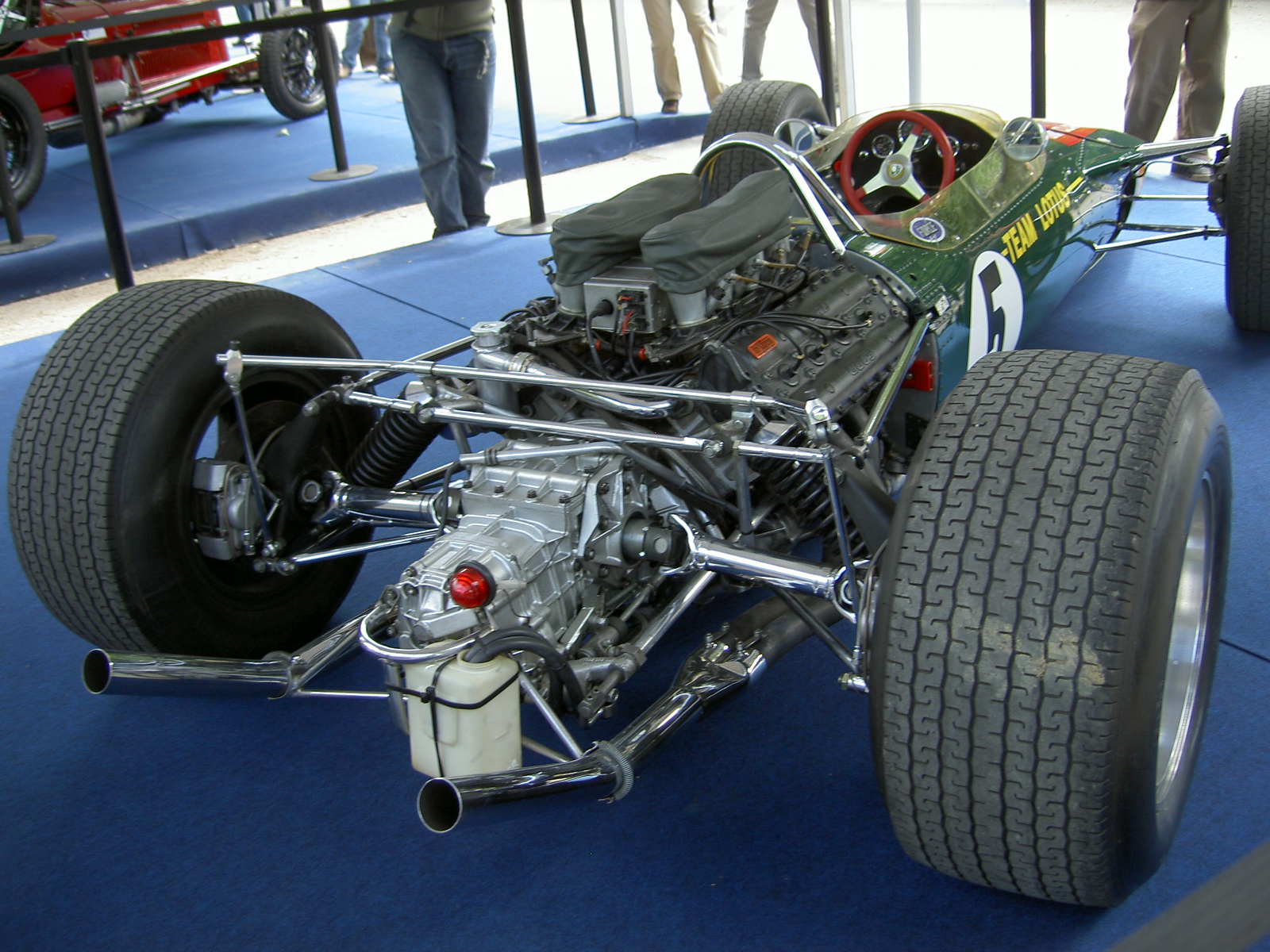
Figure: The Lotus 49, view from the rear. The Lotus 49 was one of the last Formula One cars before the introduction of aerodynamic aids.
So, when it comes to our ability to compute we are extraordinary, not compute in our conscious mind, but the underlying neuron firings that underpin both our consciousness, our subconsciousness as well as our motor control etc.
If we think of ourselves as vehicles, then we are massively overpowered. Our ability to generate derived information from raw fuel is extraordinary. Intellectually we have formula one engines.
But in terms of our ability to deploy that computation in actual use, to share the results of what we have inferred, we are very limited. So, when you imagine the F1 car that represents a psyche, think of an F1 car with bicycle wheels.
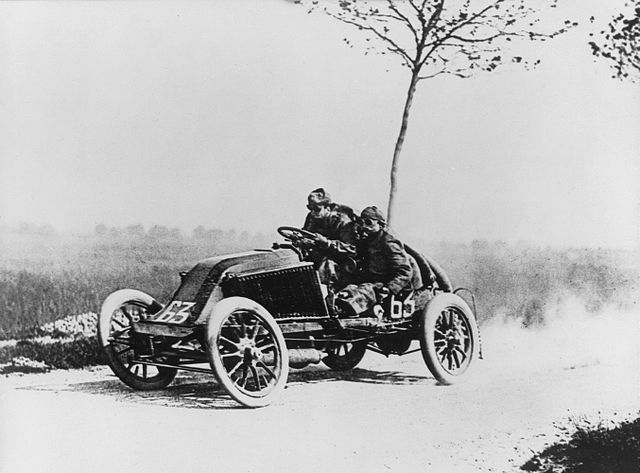
Figure: Marcel Renault races a Renault 40 cv during the Paris-Madrid race, an early Grand Prix, in 1903. Marcel died later in the race after missing a warning flag for a sharp corner at Couhé Vérac, likely due to dust reducing visibility.
Just think of the control a driver would have to have to deploy such power through such a narrow channel of traction. That is the beauty and the skill of the human mind.
In contrast, our computers are more like go-karts. Underpowered, but with well-matched tires. They can communicate far more fluidly. They are more efficient, but somehow less extraordinary, less beautiful.
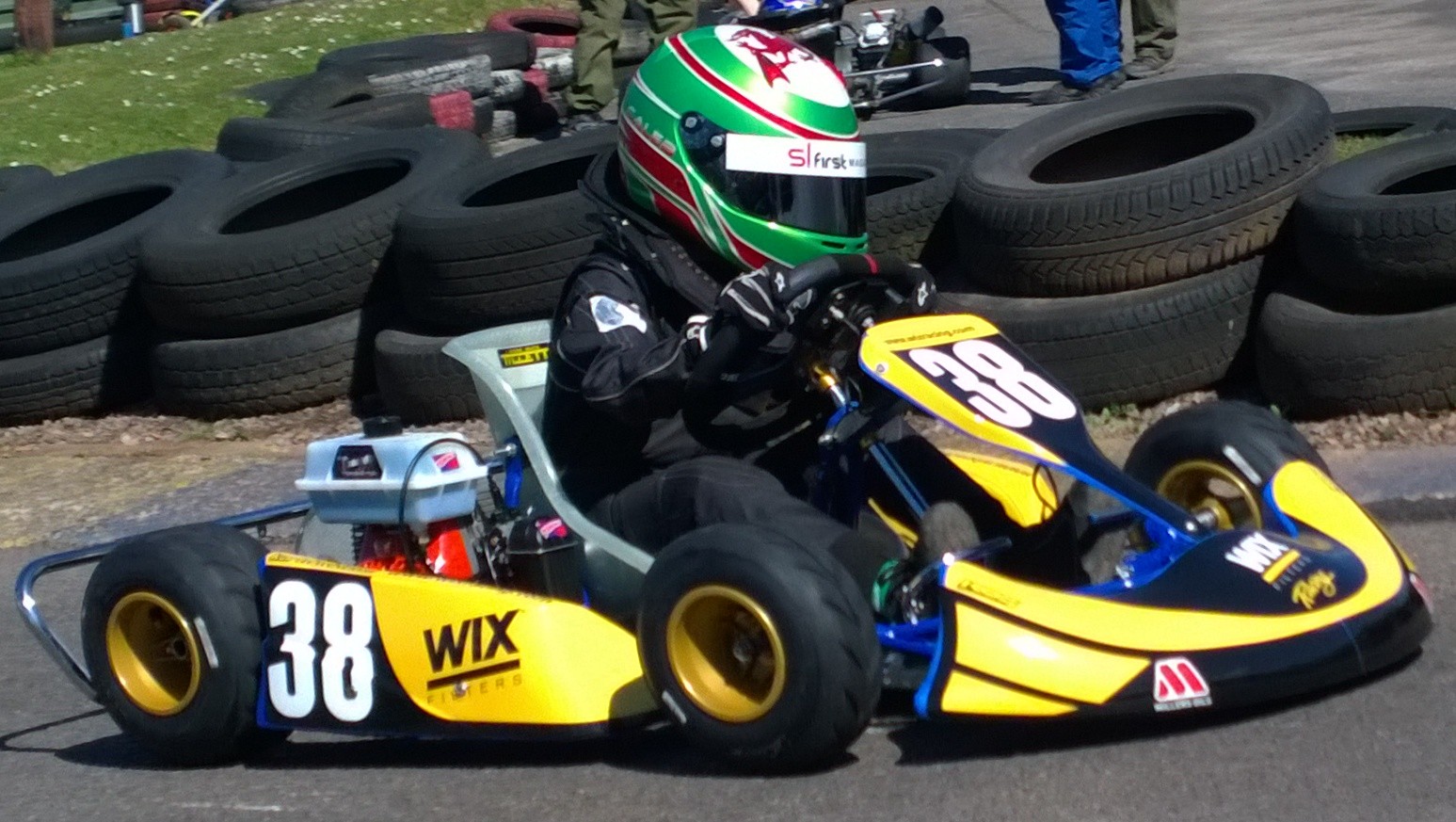
Figure: Caleb McDuff driving for WIX Silence Racing.
For humans, that means much of our computation should be dedicated to considering what we should compute. To do that efficiently we need to model the world around us. The most complex thing in the world around us is other humans. So, it is no surprise that we model them. We second guess what their intentions are, and our communication is only necessary when they are departing from how we model them. Naturally, for this to work well, we need to understand those we work closely with. It is no surprise that social communication, social bonding, forms so much of a part of our use of our limited bandwidth.
There is a second effect here, our need to anthropomorphize objects around us. Our tendency to model our fellow humans extends to when we interact with other entities in our environment. To our pets as well as inanimate objects around us, such as computers or even our cars. This tendency to over interpret could be a consequence of our limited ability to communicate.2
For more details see this paper “Living Together: Mind and Machine Intelligence”, and this TEDx talk and Chapter 1 in Lawrence (2024).
This new paradigm brings its own challenges:
- Finding the right balance between automation and human judgment, particularly in regulated industries like finance
- Ensuring that systems remain transparent even as they become more sophisticated
- Addressing regulatory considerations around AI use in financial decision-making
- Maintaining appropriate human oversight while leveraging the efficiency of AI systems
The organizations that will thrive are those that view AI not as a replacement for human expertise, but as a way to amplify that expertise and make it more accessible.
Machine Learning in Society and Organisations
When organizations think about deploying ML and AI, there’s a tendency to focus primarily on the technology implementation. However, the most successful deployments recognize that this is as much a cultural transformation as it is a technological one.
The question shouldn’t be “How do we automate this process?” but rather “How do we empower our people to achieve better outcomes?” This requires a balanced approach that recognizes the unique strengths of both humans and machines.
Humans excel at contextual understanding, ethical judgment, creative problem-solving, and emotional intelligence. Machines excel at pattern recognition across vast datasets, consistency, tireless operation, and rapid information processing. The true potential emerges when we design systems that combine these complementary strengths.
For most institutions, this means creating systems that augment human judgment rather than replace it - systems that provide analysts, advisors, and executives with enhanced capabilities while keeping them firmly in control of the decision-making process.
The organisations that will thrive in this new era are those that approach AI deployment with a human-centered mindset, focused on amplifying human capabilities rather than simply automating existing processes.
For financial institutions, the strategic implications are significant:
- Competitive advantage will increasingly come from providing superior user experiences that reduce cognitive burden
- Employee satisfaction and productivity can increase as systems become more intuitive to use
- Training time for new employees can decrease as systems become more self-explanatory
- Client satisfaction can improve as services become more personalized and responsive
The winners in this new era won’t necessarily be those with the most advanced AI models, but those who best integrate these capabilities into human-centered workflows that put users back in control.
Building the Future
As we build this future, here are the principles that should guide us:
- Start with human needs and workflows, then determine how technology can support them
- Create systems that explain their conclusions and recommendations in human terms
- Design for collaboration between human expertise and machine capabilities
- Ensure that humans remain in control of key decisions, with AI serving as a powerful tool
The exciting thing about this new wave of machine learning technology is that it can bring people closer to machines, give humans more control over what machines are doing. We’re entering an era where we can ask computers to do what we need, rather than adapting ourselves to what computers can do.
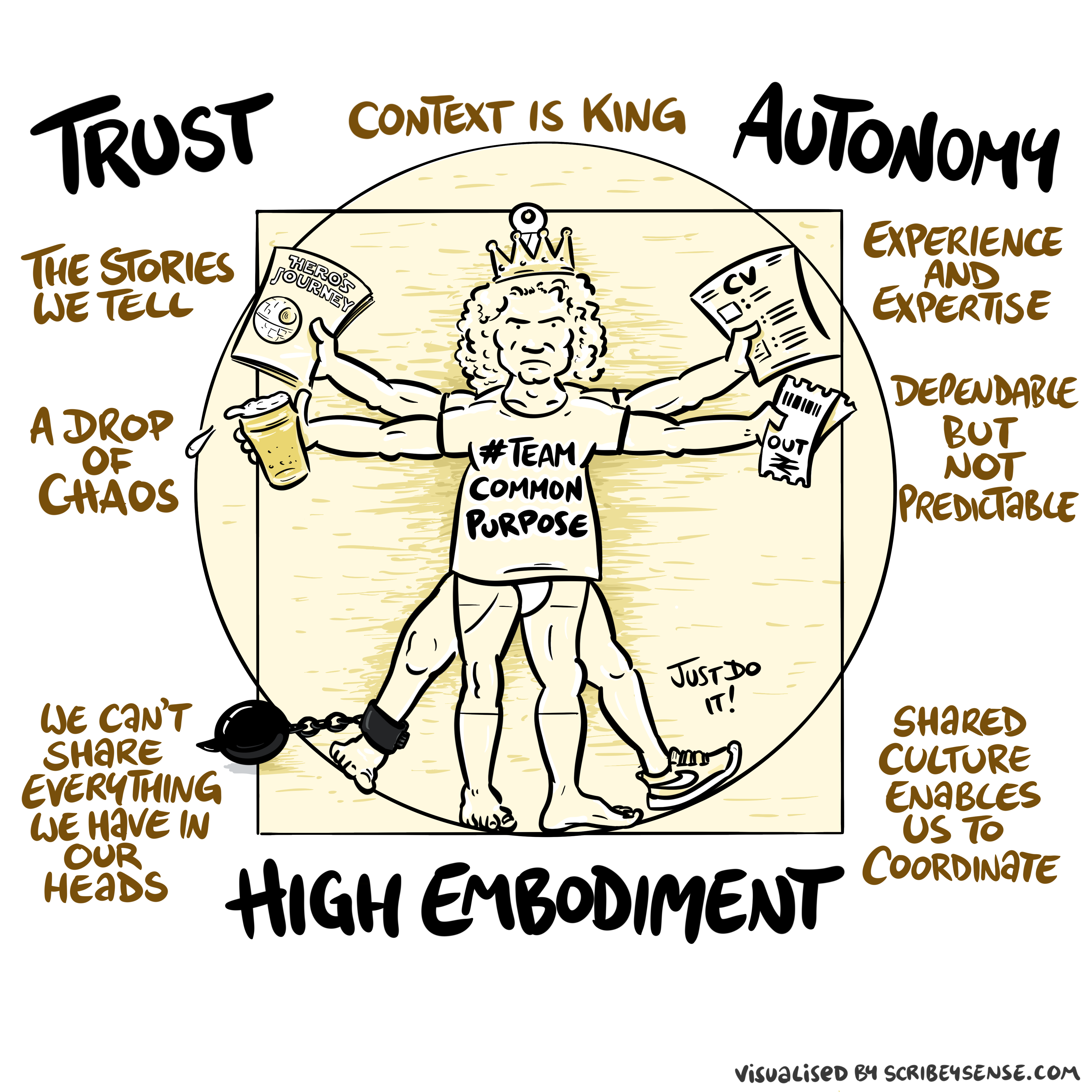
Figure: The relationships between trust, autonomy and embodiment are key to understanding how to properly deploy AI systems in a way that avoids digital autocracy. (Illustration by Dan Andrews inspired by Chapter 3 “Intent” of “The Atomic Human” Lawrence (2024))
This illustration was created by Dan Andrews after reading Chapter 3 “Intent” of “The Atomic Human” book. The chapter explores the concept of intent in AI systems and how trust, autonomy, and embodiment interact to shape our relationship with technology. Dan’s drawing captures these complex relationships and the balance needed for responsible AI deployment.
See blog post on Dan Andrews image from Chapter 3.
See blog post on Dan Andrews image from Chapter 3..
Conclusion
The true revolution in artificial intelligence isn’t about creating machines that think like humans. It’s about creating machines that can understand human thinking and adapt to it.
For financial institutions and investors, this represents a once-in-a-generation opportunity to reimagine how humans and machines work together to achieve better outcomes.
The future belongs to those who recognize this shift and leverage it to create more intuitive, more responsive, and more human-centered systems that put people back in control of technology.