The AI Value Chain: Research and Policy Priorities
Abstract
A healthy relationship between society and technology would be one where both shape each. As innovation proceeds so policymakers face complex decisions about how to govern these technologies. This keynote examines the AI value chain from technical research through development, deployment, and societal impact.
The talk explores the dynamics that shape AI governance: the bandwidth disparity between human and machine, the evolution of societal information flow and the necessary shift from productivity-driven to attention-driven innovation models. These dynamics create power imbalances that traditional policy approaches struggle to address.
By comparing the traditional productivity flywheel with the emerging attention reinvestment cycle, we identify why conventional macroeconomic interventions may not connect effectively to microeconomic incentives in AI markets. The talk concludes with research and policy priorities that can help bridge this gap, ensuring AI development serves broader societal goals through both technical solutions and democratic engagement.
Introduction: The AI Value Chain and Information Flows
Artificial General Vehicle
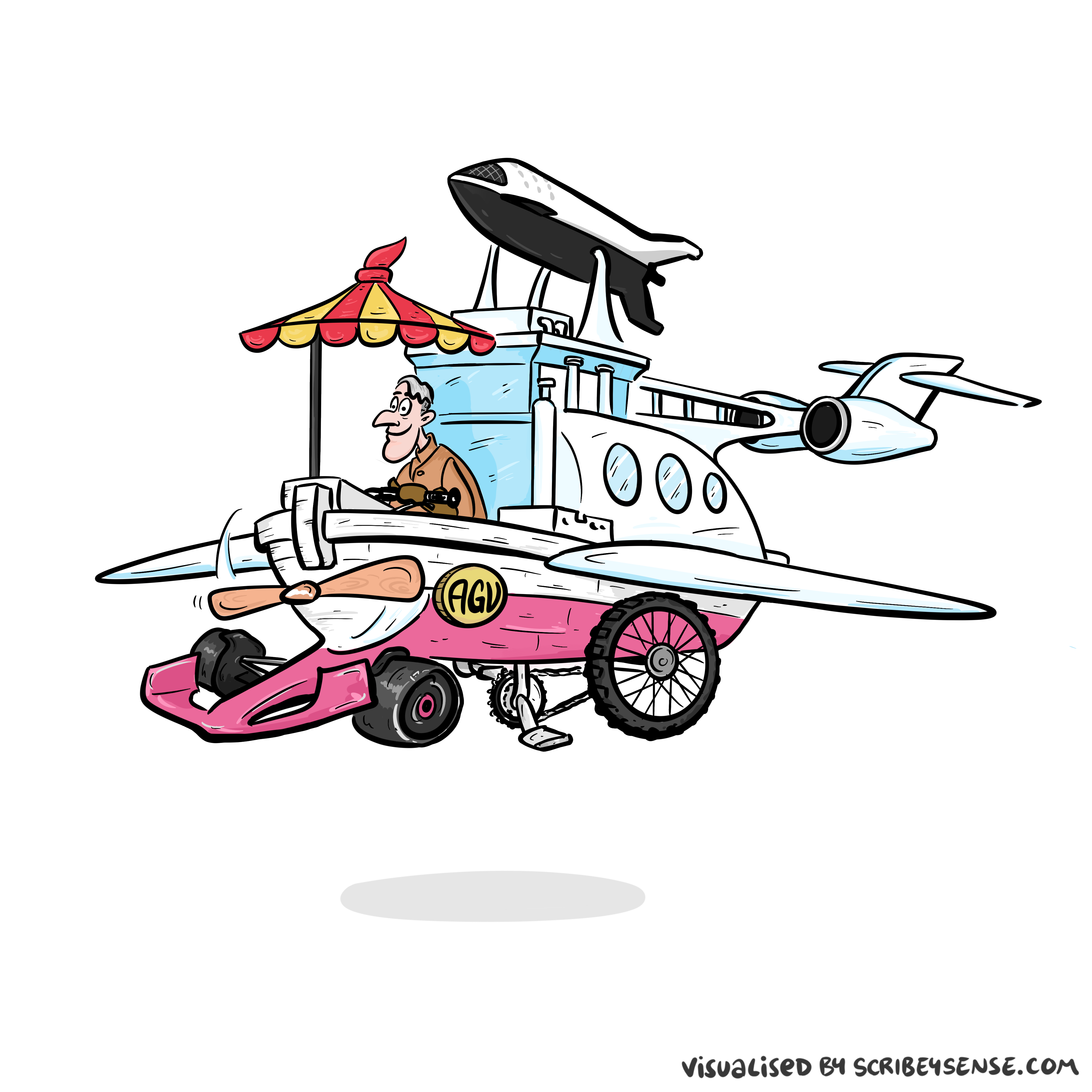
Figure: The notion of artificial general intelligence is as absurd as the notion of an artifical general vehicle.
I often turn up to talks with my Brompton bicycle. Embarrassingly I even took it to Google which is only a 30 second walk from King’s Cross station. That made me realise it’s become a sort of security blanket. I like having it because it’s such a flexible means of transport.
But is the Brompton an “artificial general vehicle”? A vehicle that can do everything? Unfortunately not, for example it’s not very good for flying to the USA. There is no artificial general vehicle that is optimal for every journey. Similarly there is no such thing as artificial general intelligence. The idea is artificial general nonsense.
That doesn’t mean there aren’t different principles to intelligence we can look at. Just like vehicles have principles that apply to them. When designing vehicles we need to think about air resistance, friction, power. We have developed solutions such as wheels, different types of engines and wings that are deployed across different vehicles to achieve different results.
Intelligence is similar. The notion of artificial general intelligence is fundamentally eugenic. It builds on Spearman’s term “general intelligence” which is part of a body of literature that was looking to assess intelligence in the way we assess height. The objective then being to breed greater intelligences (Lyons, 2022).
The notion of “artificial general intelligence” permeates discussions of AI, leading to misguided efforts to regulate hypothetical superintelligent systems while neglecting the real-world impacts of existing digital systems. To develop effective policy for the AI value chain, we need to move beyond misconceptions and understand the fundamental differences between human and machine intelligence.
As artificial intelligence increasingly reshapes our society and economy, policymakers face complex questions about how to govern these rapidly evolving technologies. To make wise decisions, we need a clear understanding of the “AI value chain” - the spectrum from research and development through deployment to societal impact.
Locked-In Intelligence: The Human Condition
Before discussing the challenges of AI governance, we need to understand a fundamental aspect of human experience: we all have what I call “locked-in intelligence.” Like Jean-Dominique Bauby, the editor of Elle magazine who suffered a stroke leaving him only able to communicate by blinking one eye, we all experience the world through limited interfaces.
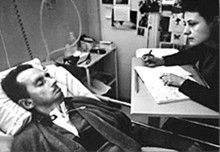
Figure: Jean Dominique Bauby was the Editor in Chief of the French Elle Magazine, he suffered a stroke that destroyed his brainstem, leaving him only capable of moving one eye. Jean Dominique became a victim of locked in syndrome.
Incredibly, Jean Dominique wrote his book after he became locked in. It took him 10 months of four hours a day to write the book. Each word took two minutes to write.
The idea behind embodiment factors is that we are all in that situation. While not as extreme as for Bauby, we all have somewhat of a locked in intelligence.
See Lawrence (2024) Bauby, Jean Dominique p. 9–11, 18, 90, 99-101, 133, 186, 212–218, 234, 240, 251–257, 318, 368–369.
While our situation is not as extreme as Bauby’s, we are all constrained by our embodiment - by our senses, by our physical form, and most importantly by our limited ability to communicate our thoughts and ideas to others. This constraint is fundamental to understanding the bandwidth disparity between humans and machines.
The Communication Bandwidth Disparity
At the foundation of the AI value chain is a disparity: the vast difference in communication rates between humans and digital systems. While machines can process information at rates measured in gigabits or terabits per second, humans are limited to just a few bits per second through reading, viewing, or listening.
This communication bandwidth gap creates structural power imbalances that are pervasive in the digital value chain. Digital systems can process, analyze, and act upon information at speeds humans simply cannot match - creating asymmetries in knowledge, decision-making ability, and power.
Bandwidth vs Complexity
The computer communicates in Gigabits per second, One way of imagining just how much slower we are than the machine is to look for something that communicates in nanobits per second.
![]() |
|||
bits/min | \(100 \times 10^{-9}\) | \(2,000\) | \(600 \times 10^9\) |
Figure: When we look at communication rates based on the information passing from one human to another across generations through their genetics, we see that computers watching us communicate is roughly equivalent to us watching organisms evolve. Estimates of germline mutation rates taken from Scally (2016).
Figure: Bandwidth vs Complexity.
The challenge we face is that while speed is on the side of the machine, complexity is on the side of our ecology. Many of the risks we face are associated with the way our speed undermines our ecology and the machines speed undermines our human culture.
See Lawrence (2024) Human evolution rates p. 98-99. See Lawrence (2024) Psychological representation of Ecologies p. 323-327.
Changing Flows of Information
The bandwidth disparity has transformed how information flows in our society, with profound implications for the AI value chain.
New Flow of Information
Classically the field of statistics focused on mediating the relationship between the machine and the human. Our limited bandwidth of communication means we tend to over-interpret the limited information that we are given, in the extreme we assign motives and desires to inanimate objects (a process known as anthropomorphizing). Much of mathematical statistics was developed to help temper this tendency and understand when we are valid in drawing conclusions from data.
Figure: The trinity of human, data, and computer, and highlights the modern phenomenon. The communication channel between computer and data now has an extremely high bandwidth. The channel between human and computer and the channel between data and human is narrow. New direction of information flow, information is reaching us mediated by the computer. The focus on classical statistics reflected the importance of the direct communication between human and data. The modern challenges of data science emerge when that relationship is being mediated by the machine.
Data science brings new challenges. In particular, there is a very large bandwidth connection between the machine and data. This means that our relationship with data is now commonly being mediated by the machine. Whether this is in the acquisition of new data, which now happens by happenstance rather than with purpose, or the interpretation of that data where we are increasingly relying on machines to summarize what the data contains. This is leading to the emerging field of data science, which must not only deal with the same challenges that mathematical statistics faced in tempering our tendency to over interpret data but must also deal with the possibility that the machine has either inadvertently or maliciously misrepresented the underlying data.
This represents the first major shift in how information flows - from humans to digital systems that process it at scale, then back to decision-makers. This has created significant power imbalances, as organisations with access to data and processing capabilities have gained unprecedented insights into human behavior.
In the UK, policy responses to these imbalances include the Online Safety Bill, which aims to regulate harmful content online, and the Digital Markets, Competition and Consumer Act, which addresses market dominance in digital spaces. While these interventions represent important steps, they are still catching up to the rapidly evolving reality of information flows in the AI era.
Human Analogue Machines and Information Flow
HAM
The Human-Analogue Machine or HAM therefore provides a route through which we could better understand our world through improving the way we interact with machines.
Figure: The trinity of human, data, and computer, and highlights the modern phenomenon. The communication channel between computer and data now has an extremely high bandwidth. The channel between human and computer and the channel between data and human is narrow. New direction of information flow, information is reaching us mediated by the computer. The focus on classical statistics reflected the importance of the direct communication between human and data. The modern challenges of data science emerge when that relationship is being mediated by the machine.
The HAM can provide an interface between the digital computer and the human allowing humans to work closely with computers regardless of their understandin gf the more technical parts of software engineering.
Figure: The HAM now sits between us and the traditional digital computer.
Of course this route provides new routes for manipulation, new ways in which the machine can undermine our autonomy or exploit our cognitive foibles. The major challenge we face is steering between these worlds where we gain the advantage of the computer’s bandwidth without undermining our culture and individual autonomy.
See Lawrence (2024) human-analogue machine (HAMs) p. 343-347, 359-359, 365-368.
The advent of generative AI - what I call Human Analogue Machines or HAMs - further transforms information flows. These systems don’t just process data statistically; they can generate human-like responses, creating a new information pathway that has the potential to redress or reinforce power imbalances.
Systems like the Horizon Post Office software demonstrate how technical systems can override human agency in ways that can be catastrophic. When machines process information faster than humans can verify it, and those machines are not socially vested, the results can be devastating for individuals caught in the system.
Trustworthiness, Intelligent Accountability, and the AI Value Chain
When considering how to govern the AI value chain, we would do well to reflect on Baroness Onora O’Neill’s insights on trust from her 2002 Reith Lectures. O’Neill argues that our modern obsession with transparency and processes often undermines rather than enhances trust.
A Question of Trust
In Baroness Onora O’Neill’s Reeith Lectures from 2002, she raises the challenge of trust. There are many aspects to her arcuments, but one of the key points she makes is that we cannot trust without the notion of duty. O’Neill is bemoaning the substitution of duty with process. The idea is that processes and transparency are supposed to hold us to account by measuring outcomes. But these processes themselves overwhelm decision makers and undermine their professional duty to deliver the right outcome.
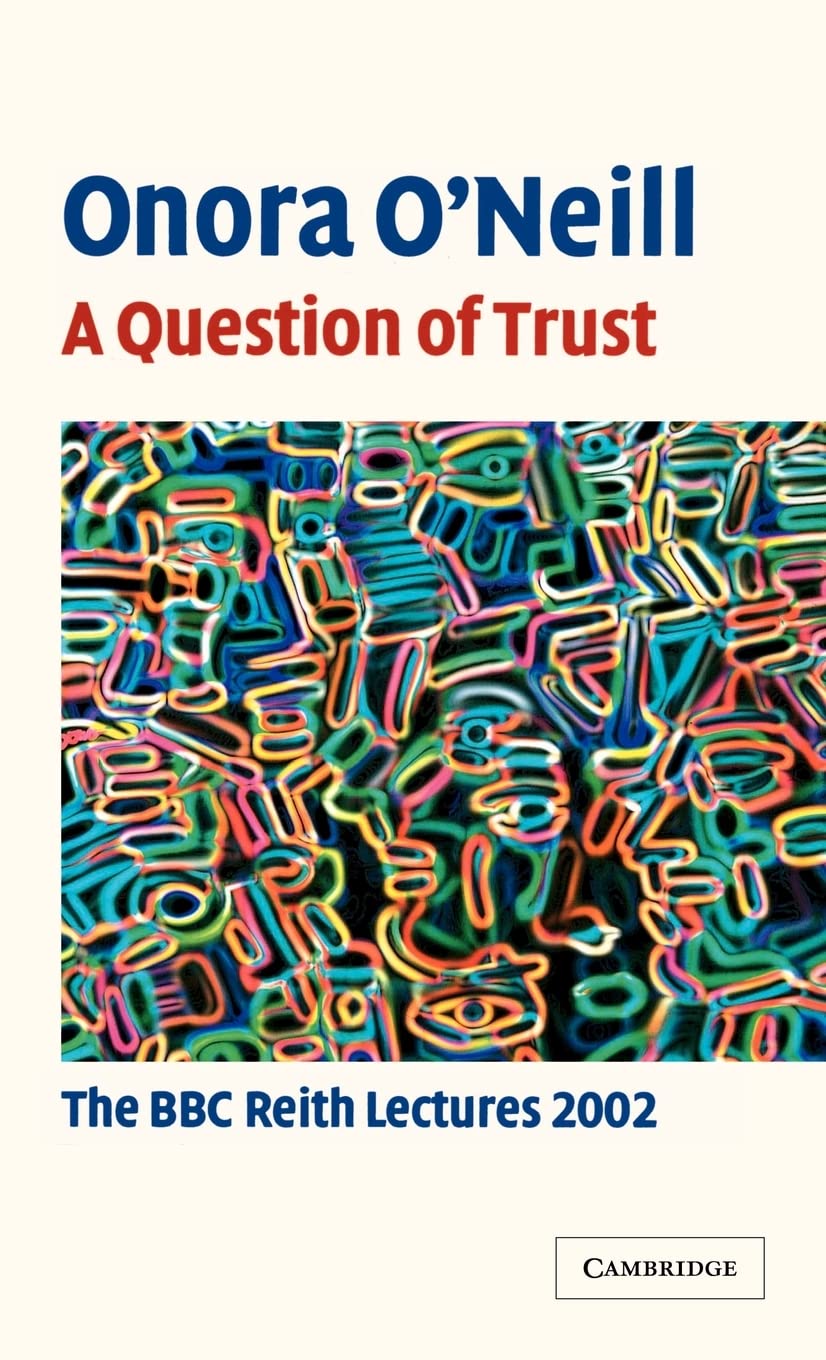
Figure: A Question of Trust by Onora O’Neil which examines the nature of trust and its role in society.
Again Univesities are to treat each applicant fairly on the basis of ability and promise, but they are supposed also to admit a socially more representative intake.
There’s no guarantee that the process meets the target.
Onora O’Neill A Question of Trust: Called to Account Reith Lectures 2002 O’Neill (2002)]
O’Neill is speaking in 2002, in the early days of the internet and before social media. Much of her thoughts are even more relevant for today than they were when she spoke. This is because the increased availability of information and machine driven decision-making makes the mistaken premise, that process is an adequate substitute for duty, more apparently plausible. But this undermines what O’Neill calls “intelligent accountability”, which is not accounting by the numbers, but through professional education and institutional safeguards.
See Lawrence (2024) O’Neill, Baroness Onora: ‘A question of trust’ lecture series (2002) p. 352, 363.
Our reflexive response to the power asymmetries created by digital systems is often to create more processes - algorithmic impact assessments, transparency requirements, ethics checklists. While these have value, they risk substituting “process” for what O’Neill calls “intelligent accountability.”
True accountability in the AI value chain cannot come through mechanical compliance with transparency requirements. It requires institutions with clear duties toward the public good, professionals educated to uphold those duties, and governance structures that foster trustworthiness rather than merely demanding trust.
The Horizon scandal illustrates this point clearly. The process of computerization projected trustworthiness, but the system lacked the institutional safeguards and professional duties that would have made it truly trustworthy. The result was catastrophic for those affected.
Public engagement isn’t merely a matter of building social license for AI systems. It’s about creating the conditions for trustworthiness throughout the AI value chain. When systems profoundly affect people’s lives, those people must have meaningful input into how the systems are designed, deployed, and governed.
Public Dialogue on AI in Public Services
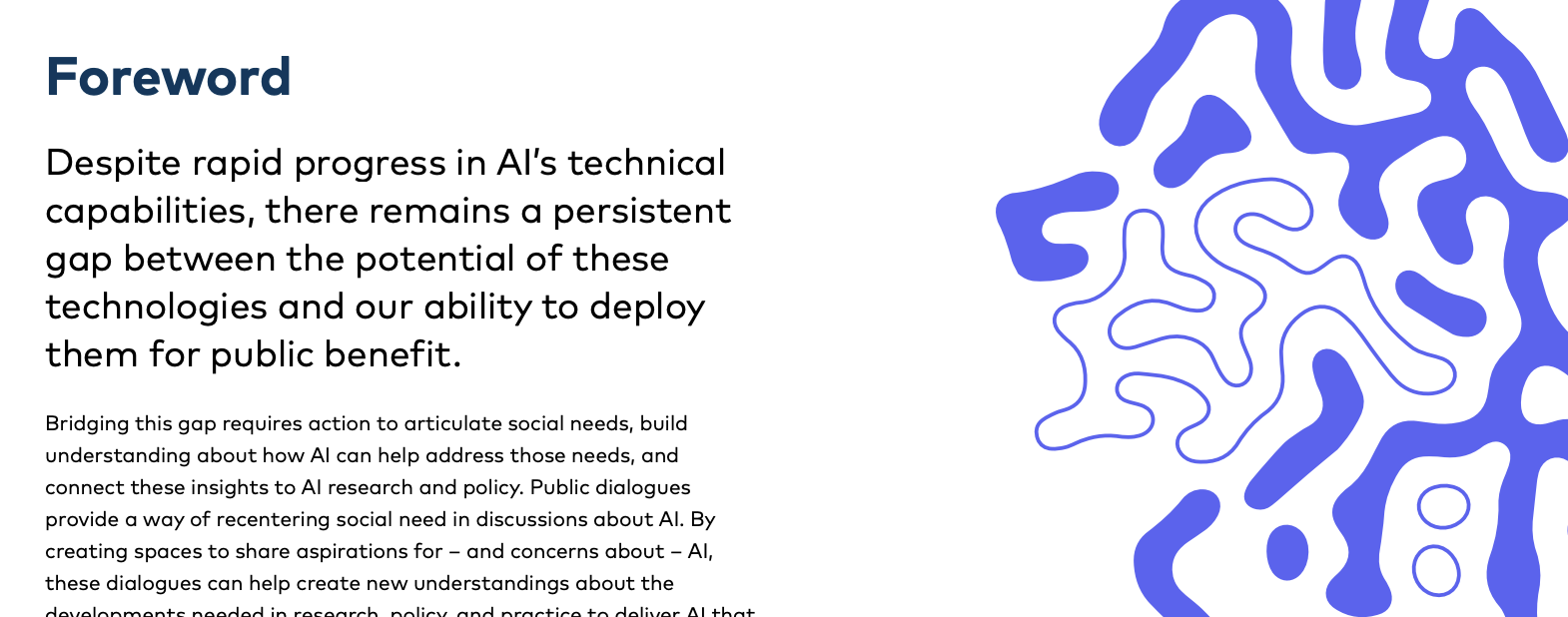
Figure: In September 2024, ai@cam convened a series of public dialogues to understand perspectives on the role of AI in delivering policy agendas.
In September 2024, ai@cam convened a series of public dialogues to understand perspectives on the role of AI in delivering priority policy agendas. Through workshops in Cambridge and Liverpool, 40 members of the public shared their views on how AI could support delivery of four key government missions around health, crime and policing, education, and energy and net zero.
The dialogue revealed a pragmatic public view that sees clear benefits from AI in reducing administrative burdens and improving service efficiency, while emphasizing the importance of maintaining human-centered services and establishing robust governance frameworks.
Key participant quotes illustrate this balanced perspective:
“It must be so difficult for GPs to keep track of how medication reacts with other medication on an individual basis. If there’s some database that shows all that, then it can only help, can’t it?”
Public Participant, Liverpool pg 10 ai@cam and Hopkins Van Mil (2024)
“I think a lot of the ideas need to be about AI being like a co-pilot to someone. I think it has to be that. So not taking the human away.”
Public Participant, Liverpool pg 15 ai@cam and Hopkins Van Mil (2024)
Work with the Royal Society showed that the public has nuanced and thoughtful views on AI technologies. They’re not technophobic - they want AI that solves real problems in healthcare, education, and other domains. But they’re concerned about transparency, accountability, and the distribution of benefits.
AI in Healthcare: Public Perspectives
In healthcare discussions, participants saw clear opportunities for AI to support NHS administration and improve service delivery, while expressing caution about AI involvement in direct patient care and diagnosis.
Participants identified several key aspirations for AI in healthcare. A major focus was on reducing the administrative workload that currently burdens healthcare professionals, allowing them to spend more time on direct patient care. There was strong support for AI’s potential in early diagnosis and preventive care, where it could help identify health issues before they become severe. The public also saw significant value in AI accelerating medical research and drug development processes, potentially leading to new treatments more quickly. Finally, participants recognized AI’s capability to help manage complex medical conditions by analyzing large amounts of patient data and identifying optimal treatment strategies. These aspirations reflect a pragmatic view of AI as a tool to enhance healthcare delivery while maintaining the central role of human medical professionals.
Illustrative quotes show the nuanced views.
“My wife [an NHS nurse] says that the paperwork side takes longer than the actual care.”
Public Participant, Liverpool pg 9 ai@cam and Hopkins Van Mil (2024)
“I wouldn’t just want to rely on the technology for something big like that, because obviously it’s a lifechanging situation.”
Public Participant, Cambridge pg 10 ai@cam and Hopkins Van Mil (2024)
Concerns focused particularly on maintaining human involvement in healthcare decisions and protecting patient privacy.
O’Neill would likely argue that earning public trust in AI requires more than transparency - it requires demonstrating trustworthiness through institutions with clear duties and the capacity to fulfill them. In the AI value chain, this means creating governance structures that can bridge between high-level values and concrete practices, while maintaining the agency and professional judgment needed for intelligent accountability.
Innovation Models: From Productivity to Attention
To understand how AI is reshaping markets and institutions, we need to compare traditional innovation models with what’s emerging in the AI economy.
The Traditional Productivity Flywheel
Productivity Flywheel
Figure: The productivity flywheel suggests technical innovation is reinvested.
The productivity flywheel should return the gains released by productivity through funding. This relies on the economic value mapping the underlying value.
This traditional model of innovation focused on improving productivity - producing more output with the same input. Government interventions at the macro level (R&D funding, infrastructure, education) connected clearly to micro-level incentives for businesses and entrepreneurs. When this system worked well, it created broadly shared prosperity.
New Productivity Paradox
Thus we face a new productivity paradox. The classical tools of economic intervention cannot map hard-to-measure supply and demand of quality human attention. So how do we build a new economy that utilises our lead in human capital and delivers the digital future we aspire to?
One answer is to look at the human capital index. This measures the quality and quantity of the attention economy via the health and education of our population.
We need to value this and find a way to reinvest human capital, returning the value of the human back into the system when considering productivity gains from technology like AI.
This means a tighter mapping between what the public want and what the innovation economy delivers. It means more agile policy that responds to public dialogue with tangible solutions co-created with the people who are doing the actual work. It means, for example, freeing up a nurse’s time with technology tools and allowing them to spend that time with patients.
To deliver this, our academic institutions need to step up. Too often in the past we have been distant from the difficulties that society faces. We have been too remote from the real challenges of everyday lives — challenges that don’t make the covers of prestige science magazines. People are rightly angry that innovations like AI have yet to address the problems they face, including in health, social care and education.
Of course, universities cannot fix this on their own, but academics can operate as honest brokers that bridge gaps between public and private considerations, convene different groups and understand, celebrate and empower the contributions of individuals.
This requires people who are prepared to dedicate their time to improving each other’s lives, developing new best practices and sharing them with colleagues and coworkers.
To preserve our human capital and harness our potential, we need the AI alchemists to provide us with solutions that can serve both science and society.
The Attention Reinvestment Cycle
The Attention Reinvestment Cycle
AI creates time savings for professionals
Freed attention reinvested in knowledge sharing
Organic growth through professional networks
Solutions spread through peer-to-peer learning
Frontline workers become technology champions
Sustainable, scalable implementation
The attention reinvestment cycle offers a sustainable model for AI adoption. When AI tools save professional time, the key is reinvesting some of that saved attention in sharing knowledge and mentoring colleagues. This allows solutions to spread organically through professional networks.
Unlike top-down implementation, this approach builds momentum through peer-to-peer learning. Frontline workers become both beneficiaries and champions of technology, creating a sustainable cycle of improvement and adoption.
This model has proven particularly effective in healthcare and local government settings, where professional networks are strong and peer learning is already embedded in organizational culture.
In the AI economy, we’re seeing a shift from the productivity flywheel to what I call the attention reinvestment cycle. Here, the key asset is not just productive capacity but human attention and data. Organizations capture attention, which generates data, which improves AI systems, which better captures attention…and the cycle continues.
Attention Reinvestment Cycle
Figure: The attention flywheel focusses on reinvesting human capital.
While the traditional productivity flywheel focuses on reinvesting financial capital, the attention flywheel focuses on reinvesting human capital - our most precious resource in an AI-augmented world. This requires deliberately creating systems that capture the value of freed attention and channel it toward human-centered activities that machines cannot replicate.
This shift creates a fundamental challenge for policy. Traditional macroeconomic interventions don’t necessarily connect to the microeconomic incentives in an attention-driven economy. Investments in infrastructure or education don’t automatically translate to broadly shared benefits when the core dynamic is capturing and monetizing attention.
Policy Implications for the AI Value Chain
Given these dynamics - the bandwidth disparity, changed information flows, and the shift from productivity to attention - what does this mean for policy across the AI value chain?
First, policy must recognize data as the critical resource flowing through the AI value chain. The concentration of data collection and processing capabilities creates power imbalances that need to be addressed through thoughtful governance.
Data Readiness Levels
Data Readiness Levels (Lawrence, 2017) are an attempt to develop a language around data quality that can bridge the gap between technical solutions and decision makers such as managers and project planners. They are inspired by Technology Readiness Levels which attempt to quantify the readiness of technologies for deployment.
See this blog post on Data Readiness Levels.
Three Grades of Data Readiness
Data-readiness describes, at its coarsest level, three separate stages of data graduation.
- Grade C - accessibility
- Transition: data becomes electronically available
- Grade B - validity
- Transition: pose a question to the data.
- Grade A - usability
The important definitions are at the transition. The move from Grade C data to Grade B data is delimited by the electronic availability of the data. The move from Grade B to Grade A data is delimited by posing a question or task to the data (Lawrence, 2017).
Second, we need institutional innovations that can bridge between macro-level policy interventions and micro-level incentives in an attention economy. This might include data trusts, platform governance models, and other mechanisms that align corporate incentives with public benefits.
Supply Chain of Ideas
Model is “supply chain of ideas” framework, particularly in the context of information technology and AI solutions like machine learning and large language models. You suggest that this idea flow, from creation to application, is similar to how physical goods move through economic supply chains.
In the realm of IT solutions, there’s been an overemphasis on macro-economic “supply-side” stimulation - focusing on creating new technologies and ideas - without enough attention to the micro-economic “demand-side” - understanding and addressing real-world needs and challenges.
Imagining the supply chain rather than just the notion of the Innovation Economy allows the conceptualisation of the gaps between macro and micro economic issues, enabling a different way of thinking about process innovation.
Phrasing things in terms of a supply chain of ideas suggests that innovation requires both characterisation of the demand and the supply of ideas. This leads to four key elements:
- Multiple sources of ideas (diversity)
- Efficient delivery mechanisms
- Quick deployment capabilities
- Customer-driven prioritization
The next priority is mapping the demand for ideas to the supply of ideas. This is where much of our innovation system is failing. In supply chain optimisaiton a large effort is spent on understanding current stock and managing resources to bring the supply to map to the demand. This includes shaping the supply as well as managing it.
The objective is to create a system that can generate, evaluate, and deploy ideas efficiently and effectively, while ensuring that people’s needs and preferences are met. The customer here depends on the context - it could be the public, it could be a business, it could be a government department but very often it’s individual citizens. The loss of their voice in the innovation economy is a trigger for the gap between the innovation supply (at a macro level) and the innovation demand (at a micro level).
Third, we need governance models that incorporate public dialogue and values throughout the AI value chain. Technical standards alone won’t ensure AI systems serve societal goals - we need democratic processes to define those goals and hold systems accountable.
Research and Policy Priorities
To guide effective policy development, several key research priorities emerge from this analysis of the AI value chain.
We need research into how policy interventions can effectively shape incentives in the AI economy. Traditional approaches that worked for industrial innovation may not work in an attention-driven economy with fundamentally different dynamics.
We need to develop and test new institutional models for data governance that can rebalance power in the AI value chain. This includes data trusts, cooperative models, and other approaches that give individuals and communities more control over how their data is used.
And we need better methods for incorporating public values and priorities into AI governance across the value chain. This isn’t just about consultation but about creating legitimate processes for societal direction-setting in technology development.
Innovation Economy Challenges
Innovating to serve science and society requires a pipeline of interventions. As well as advances in the technical capabilities of AI technologies, engineering knowhow is required to safely deploy and monitor those solutions in practice. Regulatory frameworks need to adapt to ensure trustworthy use of these technologies. Aligning technology development with public interests demands effective stakeholder engagement to bring diverse voices and expertise into technology design.
Building this pipeline will take coordination across research, engineering, policy and practice. It also requires action to address the digital divides that influence who benefits from AI advances. These include digital divides within the socioeconomic strata that need to be overcome – AI must not exacerbate existing equalities or create new ones. In addressing these challenges, we can be hindered by divides that exist between traditional academic disciplines. We need to develop common understanding of the problems and a shared knowledge of possible solutions.
Digital Failure Examples
The Horizon Scandal
In the UK we saw these effects play out in the Horizon scandal: the accounting system of the national postal service was computerized by Fujitsu and first installed in 1999, but neither the Post Office nor Fujitsu were able to control the system they had deployed. When it went wrong individual sub postmasters were blamed for the systems’ errors. Over the next two decades they were prosecuted and jailed leaving lives ruined in the wake of the machine’s mistakes.
See Lawrence (2024) Horizon scandal p. 371.
The Lorenzo Scandal
The Lorenzo scandal is the National Programme for IT which was intended to allow the NHS to move towards electronic health records.
The oral transcript can be found at https://publications.parliament.uk/pa/cm201012/cmselect/cmpubacc/1070/11052302.htm.
One quote from 16:54:33 in the committee discussion captures the top-down nature of the project.
Q117 Austin Mitchell: You said, Sir David, the problems came from the middle range, but surely they were implicit from the start, because this project was rushed into. The Prime Minister [Tony Blair] was very keen, the delivery unit was very keen, it was very fashionable to computerise things like this. An appendix indicating the cost would be £5 billion was missed out of the original report as published, so you have a very high estimate there in the first place. Then, Richard Granger, the Director of IT, rushed through, without consulting the professions. This was a kind of computer enthusiast’s bit, was it not? The professionals who were going to have to work it were not consulted, because consultation would have made it clear that they were going to ask more from it and expect more from it, and then contracts for £1 billion were let pretty well straightaway, in May 2003. That was very quick. Now, why were the contracts let before the professionals were consulted?
A further sense of the bullish approach taken can be found in this report from digitalhealth.net (dated May 2008). https://www.digitalhealth.net/2008/02/six-years-since-blair-seminar-began-npfit/
An analysis of the problems was published by Justinia (2017). Based on the paper, the key challenges faced in the UK’s National Programme for IT (NPfIT) included:
Lack of adequate end user engagement, particularly with frontline healthcare staff and patients. The program was imposed from the top-down without securing buy-in from stakeholders.
Absence of a phased change management approach. The implementation was rushed without proper planning for organizational and cultural changes.
Underestimating the scale and complexity of the project. The centralized, large-scale approach was overambitious and difficult to manage.
Poor project management, including unrealistic timetables, lack of clear leadership, and no exit strategy.
Insufficient attention to privacy and security concerns regarding patient data.
Lack of local ownership. The centralized approach meant local healthcare providers felt no ownership over the systems.
Communication issues, including poor communication with frontline staff about the program’s benefits.
Technical problems, delays in delivery, and unreliable software.
Failure to recognize the socio-cultural challenges were as significant as the technical ones.
Lack of flexibility to adapt to changing requirements over the long timescale.
Insufficient resources and inadequate methodologies for implementation.
Low morale among NHS staff responsible for implementation due to uncertainties and unrealistic timetables.
Conflicts between political objectives and practical implementation needs.
The paper emphasizes that while technical competence is necessary, the organizational, human, and change management factors were more critical to the program’s failure than purely technological issues. The top-down, centralized approach and lack of stakeholder engagement were particularly problematic.
Reports at the Time
Report https://publications.parliament.uk/pa/cm201012/cmselect/cmpubacc/1070/1070.pdf
Commonalities
Both the Horizon and Lorenzo scandals highlight fundamental disconnects between macro-level policy decisions and micro-level operational realities. The projects failed to properly account for how systems would actually be used in practice, with devastating consequences.
The key failures can be summarized in four main points:
The failures stemmed from insufficient consideration of local needs, capabilities, and existing systems.
There was a lack of effective feedback mechanisms from the micro to macro level.
The implementations suffered from overly rigid, top-down approaches that didn’t allow for local adaptation.
In both cases, there was insufficient engagement with end-users and local stakeholders.
These systemic failures demonstrate how large-scale digital transformations can go catastrophically wrong when there is a disconnect between high-level strategy and ground-level implementation. Future digital initiatives must bridge this macro-micro gap through meaningful stakeholder engagement and adaptable implementation approaches.
These examples provide valuable lessons for bridging the macro-micro gap in innovation. Success requires comprehensive stakeholder engagement at all levels, with system designs that can flex and adapt to local needs. Effective feedback mechanisms between implementation and policy levels are crucial, supported by phased rollouts that allow for learning and adjustment. Technical competence must be ensured across both policy-making and implementation teams, with realistic timelines based on operational realities. Independent, technically competent oversight can provide valuable guidance and accountability.
Innovation Economy Conclusion
ai@cam’s approach aims to address the macro-micro disconnects in AI innovation through several key strategies. We are building bridges between macro and micro levels, fostering interdisciplinary collaboration, engaging diverse stakeholders and voices, and providing crucial resources and training. Through these efforts, ai@cam is working to create a more integrated and effective AI innovation ecosystem.
Our implementation approach emphasizes several critical elements learned from past IT implementation failures. We focus on flexibility to adapt to changing needs, phased rollout of initiatives to manage risk, establishing continuous feedback loops for improvement, and maintaining a learning mindset throughout the process.
Looking to the future, we recognize that AI technologies and their applications will continue to evolve rapidly. This evolution requires strategic agility and a continued focus on effective implementation. We will need to remain adaptable, continuously assessing and adjusting our strategies while working to bridge capability gaps between high-level AI capabilities and on-the-ground implementation challenges.
Conclusion: Serving People, Science and Society
The AI value chain represents both remarkable opportunities and significant challenges for our society. The bandwidth disparity between humans and machines, changing information flows, and the shift from productivity to attention create new power dynamics that policy must address.
By understanding these dynamics, we can develop governance approaches that connect macro-level interventions to micro-level incentives in ways that promote broadly shared benefits. This requires rethinking traditional policy tools and developing new institutional models.
Throughout this process, public engagement is not optional but essential. Only by incorporating diverse perspectives and values can we ensure AI truly serves people, science, and society.
Thanks!
For more information on these subjects and more you might want to check the following resources.
- book: The Atomic Human
- twitter: @lawrennd
- podcast: The Talking Machines
- newspaper: Guardian Profile Page
- blog: http://inverseprobability.com