Data First Culture
Abstract
Digital transformation has offered the promise of moving from a manual decision-making world to a world where decisions can be rational, data-driven and automated. The first step to digital transformation is mapping the world of atoms (material, customers, logistic networks) into the world of bits. But the real challenges may start once this is complete. In this talk we consider the challenge of bringing technology to bear on the problems we care about, how to “bridge the innovation economy”.
Henry Ford’s Faster Horse
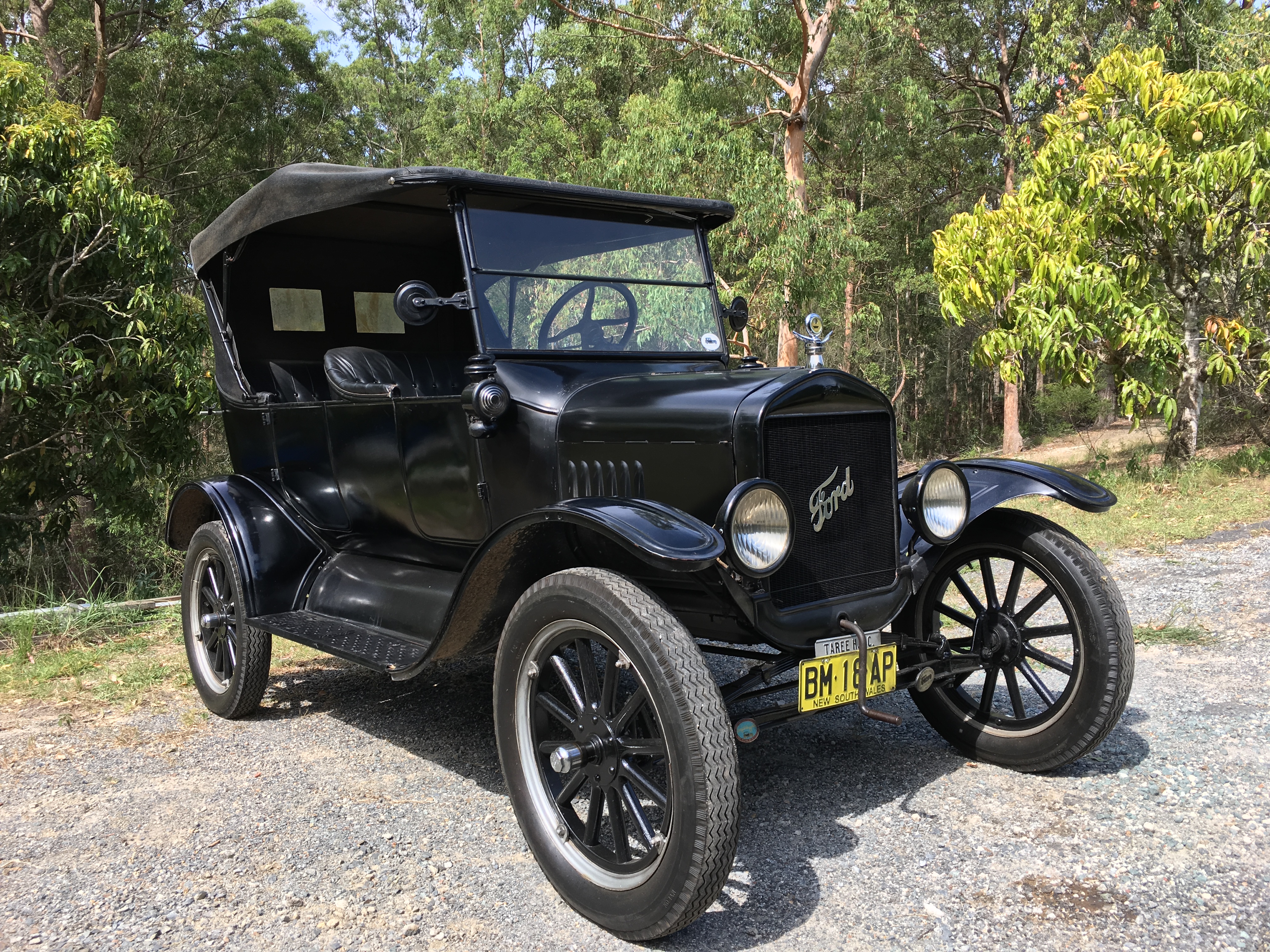
Figure: A 1925 Ford Model T built at Henry Ford’s Highland Park Plant in Dearborn, Michigan. This example now resides in Australia, owned by the founder of FordModelT.net. From https://commons.wikimedia.org/wiki/File:1925_Ford_Model_T_touring.jpg
It’s said that Henry Ford’s customers wanted a “a faster horse”. If Henry Ford was selling us artificial intelligence today, what would the customer call for, “a smarter human”? That’s certainly the picture of machine intelligence we find in science fiction narratives, but the reality of what we’ve developed is much more mundane.
Car engines produce prodigious power from petrol. Machine intelligences deliver decisions derived from data. In both cases the scale of consumption enables a speed of operation that is far beyond the capabilities of their natural counterparts. Unfettered energy consumption has consequences in the form of climate change. Does unbridled data consumption also have consequences for us?
If we devolve decision making to machines, we depend on those machines to accommodate our needs. If we don’t understand how those machines operate, we lose control over our destiny. Our mistake has been to see machine intelligence as a reflection of our intelligence. We cannot understand the smarter human without understanding the human. To understand the machine, we need to better understand ourselves.
Panacea
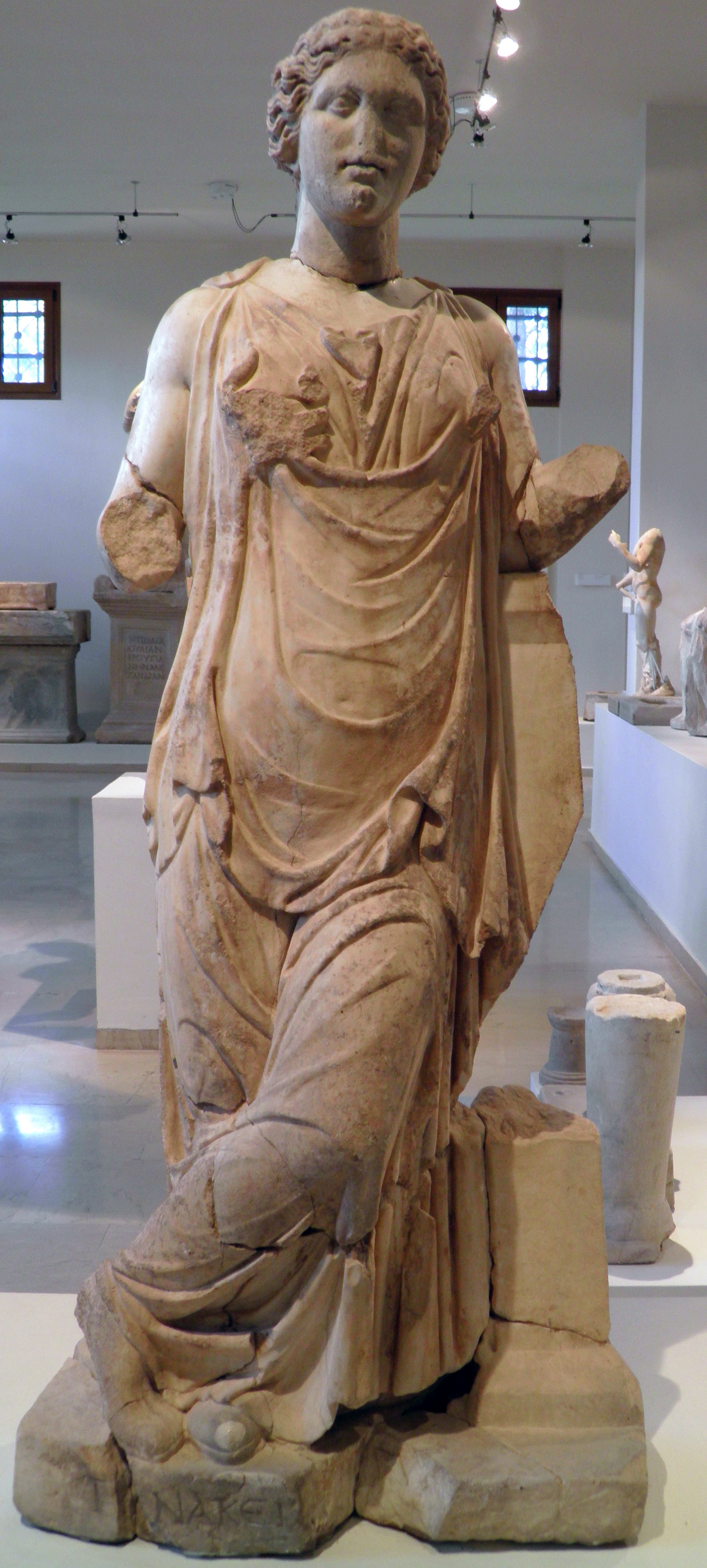
Figure: Panacea the goddess of the universal remedy.
In Greek mythology, Panacea was the goddess of the universal remedy. One consequence of the pervasive potential of technology is that it is positioned, like Panacea, as the purveyor of a universal solution. Whether it is overcoming industry’s productivity challenges, or as a salve for strained public sector services, or a remedy for pressing global challenges in sustainable development, technology is presented as an elixir to resolve society’s problems.
In practice, translation of technology into practical benefit is not simple. Moreover, a growing body of evidence shows that risks and benefits from innovations are unevenly distributed across society.
When carelessly deployed, technology risks exacerbating existing social and economic inequalities.
Introduction
The Atomic Human
Information and Embodiment
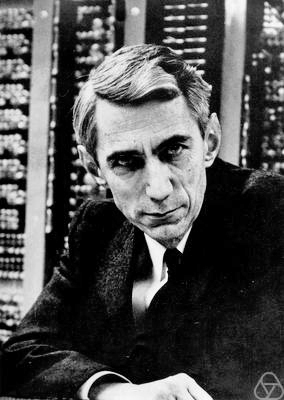
Figure: Claude Shannon (1916-2001)
bits/min | billions | 2,000 |
billion calculations/s |
~100 | a billion |
embodiment | 20 minutes | 5 billion years |
Figure: Embodiment factors are the ratio between our ability to compute and our ability to communicate. Relative to the machine we are also locked in. In the table we represent embodiment as the length of time it would take to communicate one second’s worth of computation. For computers it is a matter of minutes, but for a human, it is a matter of thousands of millions of years.
A Six Word Novel
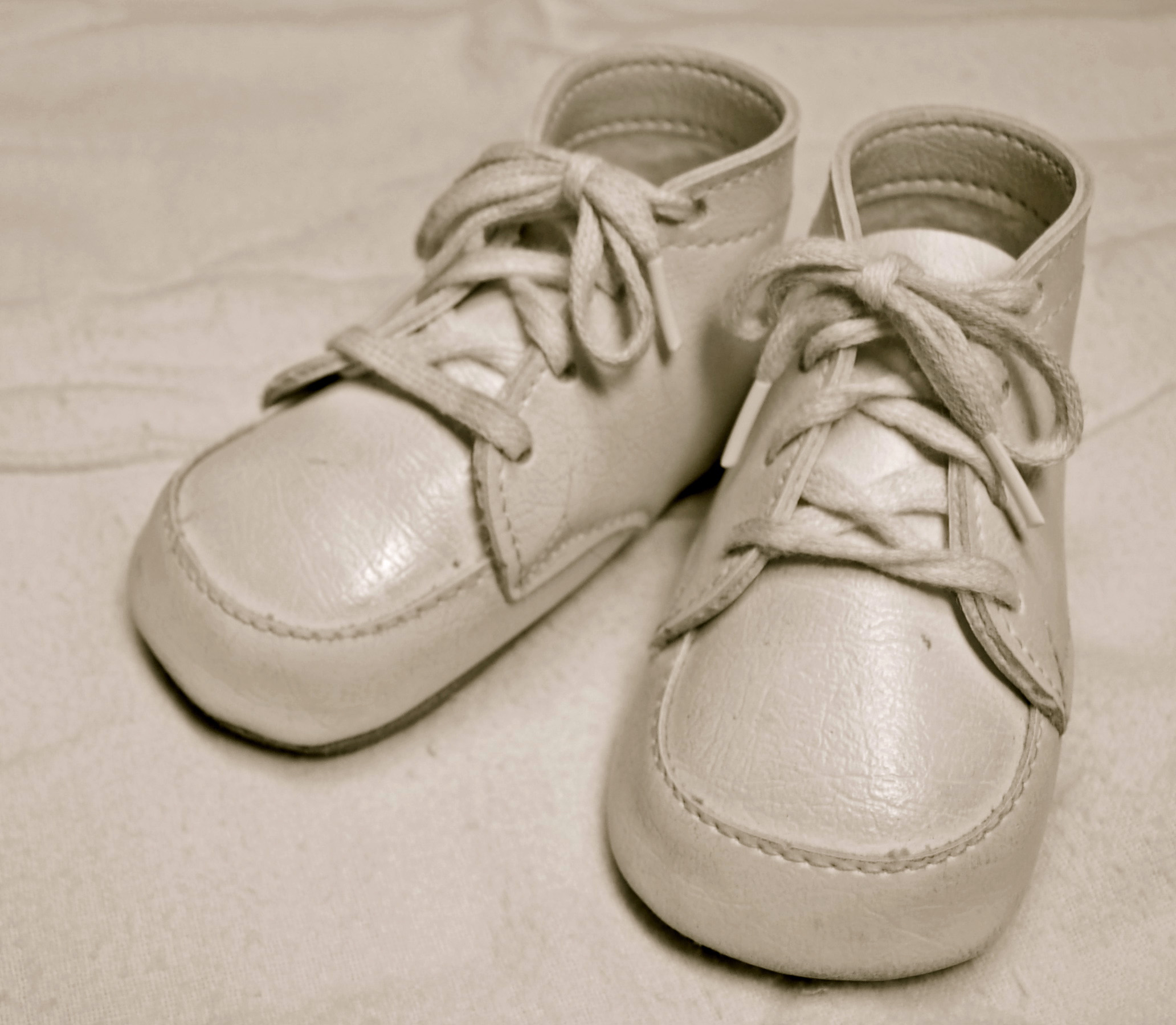
Figure: Consider the six-word novel, apocryphally credited to Ernest Hemingway, “For sale: baby shoes, never worn”. To understand what that means to a human, you need a great deal of additional context. Context that is not directly accessible to a machine that has not got both the evolved and contextual understanding of our own condition to realize both the implication of the advert and what that implication means emotionally to the previous owner.
See Lawrence (2024) baby shoes p. 368.
But this is a very different kind of intelligence than ours. A computer cannot understand the depth of the Ernest Hemingway’s apocryphal six-word novel: “For Sale, Baby Shoes, Never worn”, because it isn’t equipped with that ability to model the complexity of humanity that underlies that statement.
Bandwidth Constrained Conversations
Figure: Conversation relies on internal models of other individuals.
Figure: Misunderstanding of context and who we are talking to leads to arguments.
Embodiment factors imply that, in our communication between humans, what is not said is, perhaps, more important than what is said. To communicate with each other we need to have a model of who each of us are.
To aid this, in society, we are required to perform roles. Whether as a parent, a teacher, an employee or a boss. Each of these roles requires that we conform to certain standards of behaviour to facilitate communication between ourselves.
Control of self is vitally important to these communications.
The high availability of data available to humans undermines human-to-human communication channels by providing new routes to undermining our control of self.
The consequences between this mismatch of power and delivery are to be seen all around us. Because, just as driving an F1 car with bicycle wheels would be a fine art, so is the process of communication between humans.
If I have a thought and I wish to communicate it, I first need to have a model of what you think. I should think before I speak. When I speak, you may react. You have a model of who I am and what I was trying to say, and why I chose to say what I said. Now we begin this dance, where we are each trying to better understand each other and what we are saying. When it works, it is beautiful, but when mis-deployed, just like a badly driven F1 car, there is a horrible crash, an argument.
Computer Conversations
Figure: Conversation relies on internal models of other individuals.
Figure: Misunderstanding of context and who we are talking to leads to arguments.
Similarly, we find it difficult to comprehend how computers are making decisions. Because they do so with more data than we can possibly imagine.
In many respects, this is not a problem, it’s a good thing. Computers and us are good at different things. But when we interact with a computer, when it acts in a different way to us, we need to remember why.
Just as the first step to getting along with other humans is understanding other humans, so it needs to be with getting along with our computers.
Embodiment factors explain why, at the same time, computers are so impressive in simulating our weather, but so poor at predicting our moods. Our complexity is greater than that of our weather, and each of us is tuned to read and respond to one another.
Their intelligence is different. It is based on very large quantities of data that we cannot absorb. Our computers don’t have a complex internal model of who we are. They don’t understand the human condition. They are not tuned to respond to us as we are to each other.
Embodiment factors encapsulate a profound thing about the nature of humans. Our locked in intelligence means that we are striving to communicate, so we put a lot of thought into what we’re communicating with. And if we’re communicating with something complex, we naturally anthropomorphize them.
We give our dogs, our cats, and our cars human motivations. We do the same with our computers. We anthropomorphize them. We assume that they have the same objectives as us and the same constraints. They don’t.
This means, that when we worry about artificial intelligence, we worry about the wrong things. We fear computers that behave like more powerful versions of ourselves that will struggle to outcompete us.
In reality, the challenge is that our computers cannot be human enough. They cannot understand us with the depth we understand one another. They drop below our cognitive radar and operate outside our mental models.
The real danger is that computers don’t anthropomorphize. They’ll make decisions in isolation from us without our supervision because they can’t communicate truly and deeply with us.
New Flow of Information
Classically the field of statistics focused on mediating the relationship between the machine and the human. Our limited bandwidth of communication means we tend to over-interpret the limited information that we are given, in the extreme we assign motives and desires to inanimate objects (a process known as anthropomorphizing). Much of mathematical statistics was developed to help temper this tendency and understand when we are valid in drawing conclusions from data.
Figure: The trinity of human, data, and computer, and highlights the modern phenomenon. The communication channel between computer and data now has an extremely high bandwidth. The channel between human and computer and the channel between data and human is narrow. New direction of information flow, information is reaching us mediated by the computer. The focus on classical statistics reflected the importance of the direct communication between human and data. The modern challenges of data science emerge when that relationship is being mediated by the machine.
Data science brings new challenges. In particular, there is a very large bandwidth connection between the machine and data. This means that our relationship with data is now commonly being mediated by the machine. Whether this is in the acquisition of new data, which now happens by happenstance rather than with purpose, or the interpretation of that data where we are increasingly relying on machines to summarize what the data contains. This is leading to the emerging field of data science, which must not only deal with the same challenges that mathematical statistics faced in tempering our tendency to over interpret data but must also deal with the possibility that the machine has either inadvertently or maliciously misrepresented the underlying data.
Intellectual Debt
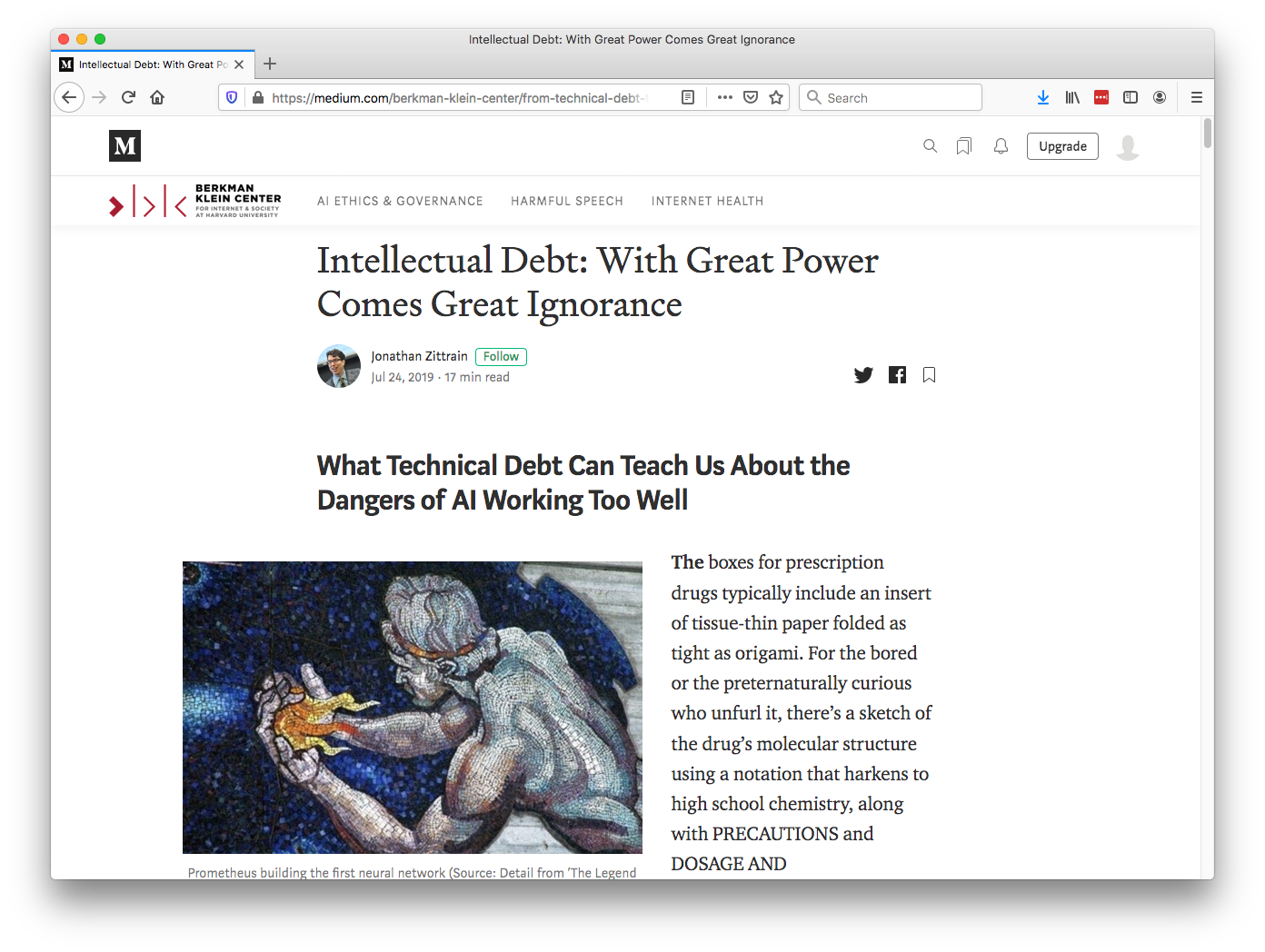
Figure: Jonathan Zittrain’s term to describe the challenges of explanation that come with AI is Intellectual Debt.
Revolution
Arguably the information revolution we are experiencing is unprecedented in history. But changes in the way we share information have a long history. Over 5,000 years ago in the city of Uruk, on the banks of the Euphrates, communities which relied on the water to irrigate their corps developed an approach to recording transactions in clay. Eventually the system of recording system became sophisticated enough that their oral histories could be recorded in the form of the first epic: Gilgamesh.
See Lawrence (2024) cuneiform p. 337, 360, 390.
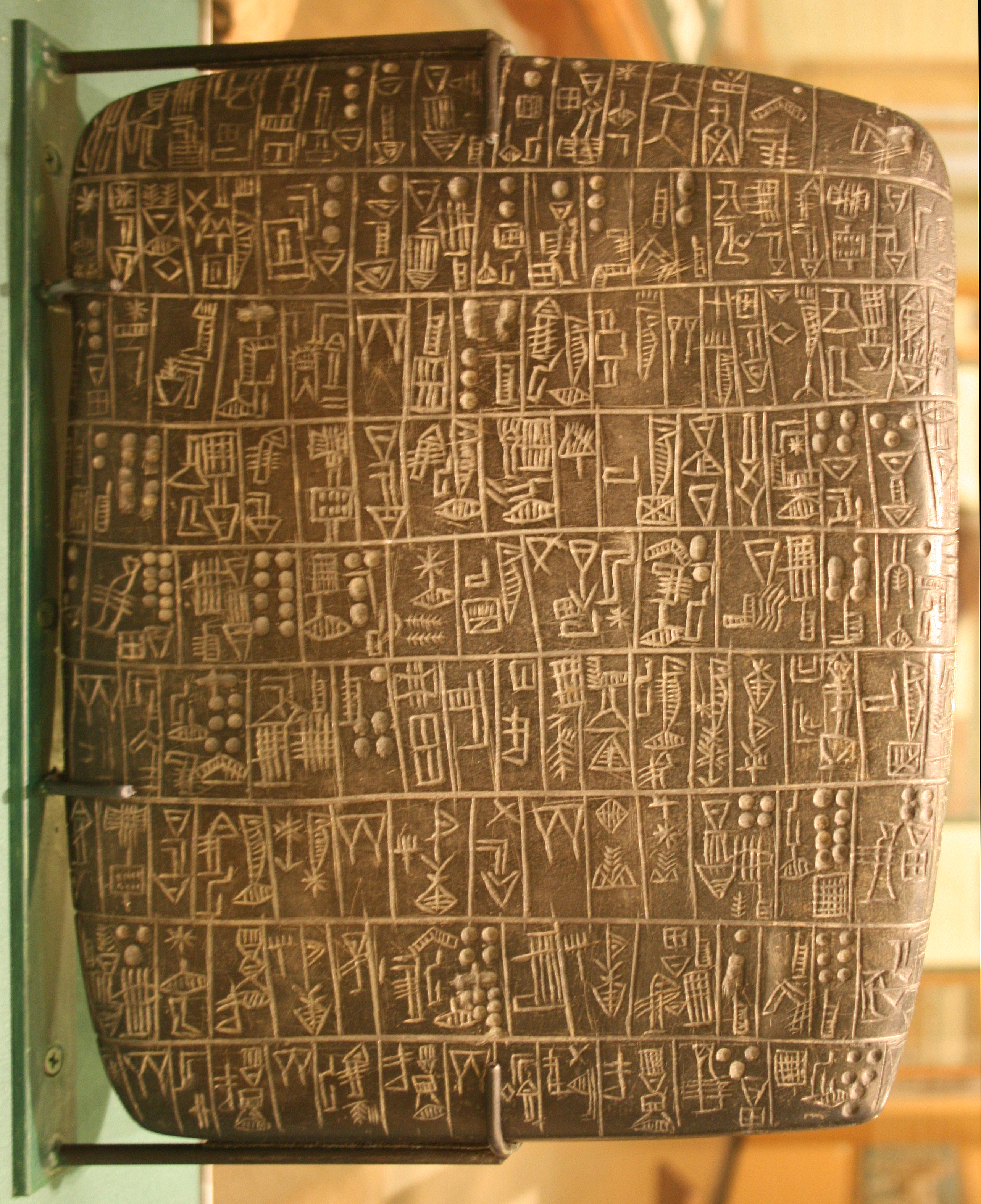
Figure: Chicago Stone, side 2, recording sale of a number of fields, probably from Isin, Early Dynastic Period, c. 2600 BC, black basalt
It was initially developed for people as a record of who owed what to whom, expanding individuals’ capacity to remember. But over a five hundred year period writing evolved to become a tool for literature as well. More pithily put, writing was invented by accountants not poets (see e.g. this piece by Tim Harford).
In some respects today’s revolution is different, because it involves also the creation of stories as well as their curation. But in some fundamental ways we can see what we have produced as another tool for us in the information revolution.
Coin Pusher
Disruption of society is like a coin pusher, it’s those who are already on the edge who are most likely to be effected by disruption.
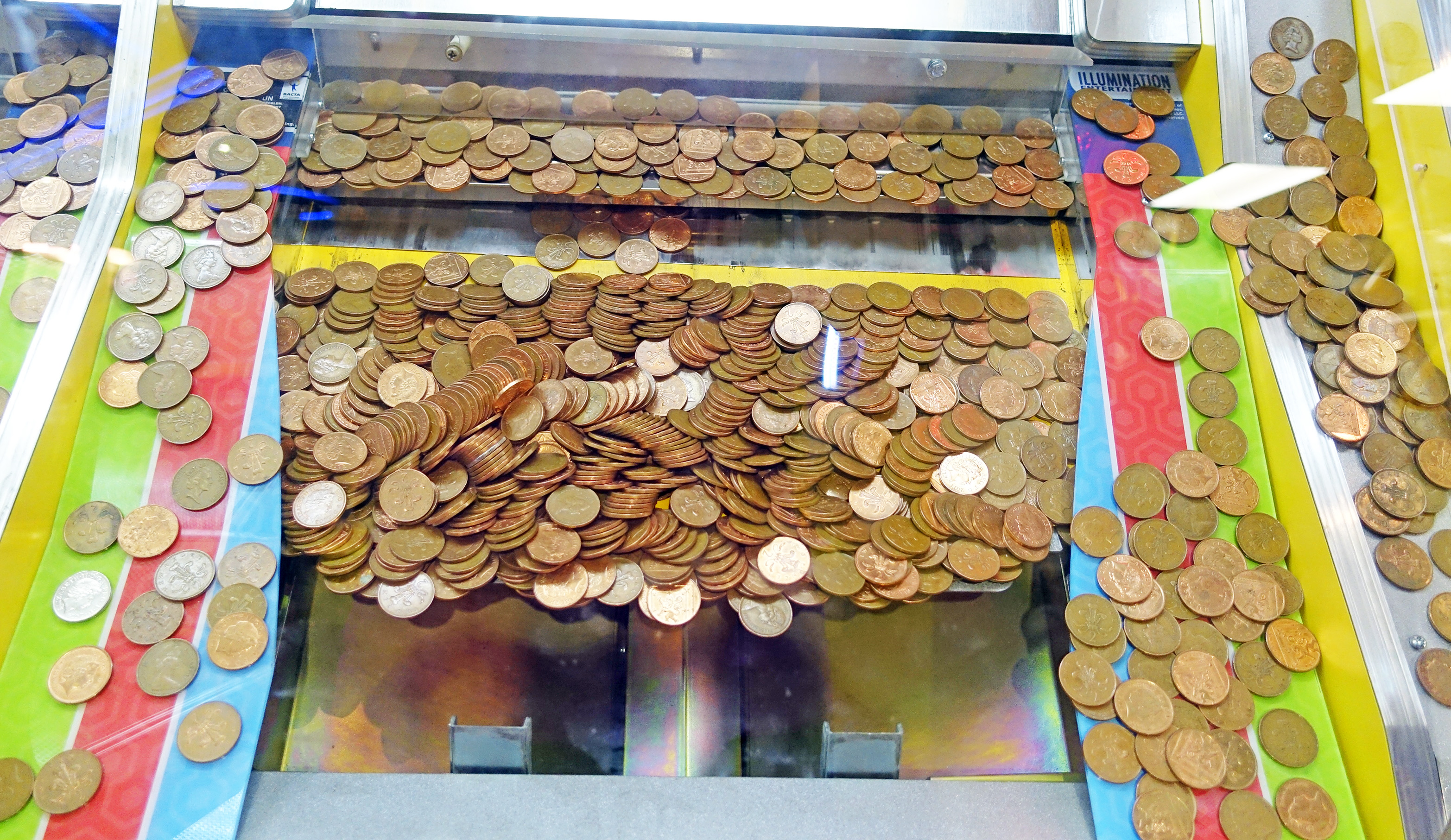
Figure: A coin pusher is a game where coins are dropped into th etop of the machine, and they disrupt those on the existing steps. With any coin drop, many coins move, but it is those on the edge, who are often only indirectly effected, but also most traumatically effected by the change.
One danger of the current hype around ChatGPT is that we are overly focussing on the fact that it seems to have significant effect on professional jobs, people are naturally asking the question “what does it do for my role?”. No doubt, there will be disruption, but the coin pusher hypothesis suggests that that disruption will likely involve movement on the same step. However it is those on the edge already, who are often not working directly in the information economy, who often have less of a voice in the policy conversation who are likely to be most disrupted.
Royal Society Report
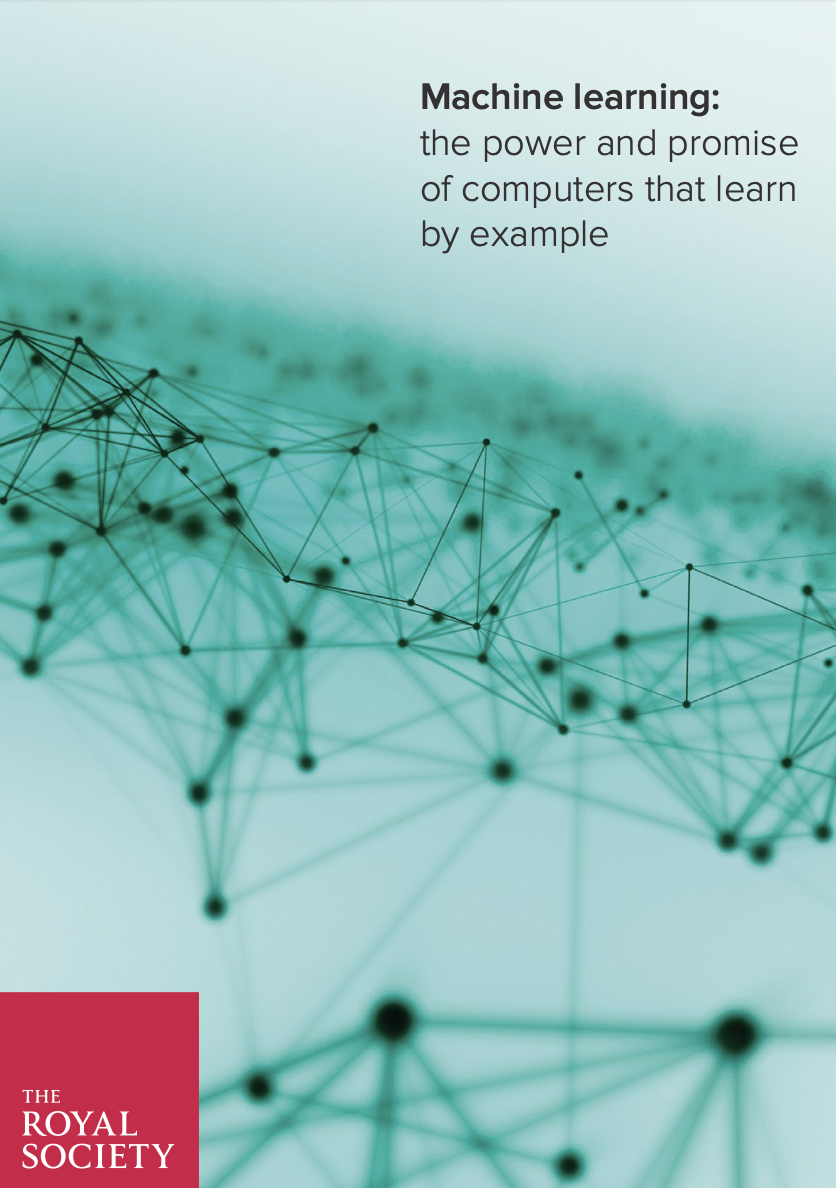
Figure: The Royal Society report on Machine Learning was released on 25th April 2017
A useful reference for state of the art in machine learning is the UK Royal Society Report, Machine Learning: Power and Promise of Computers that Learn by Example.
See Lawrence (2024) Royal Society; machine learning review and p. 25, 321, 395.
Public Research
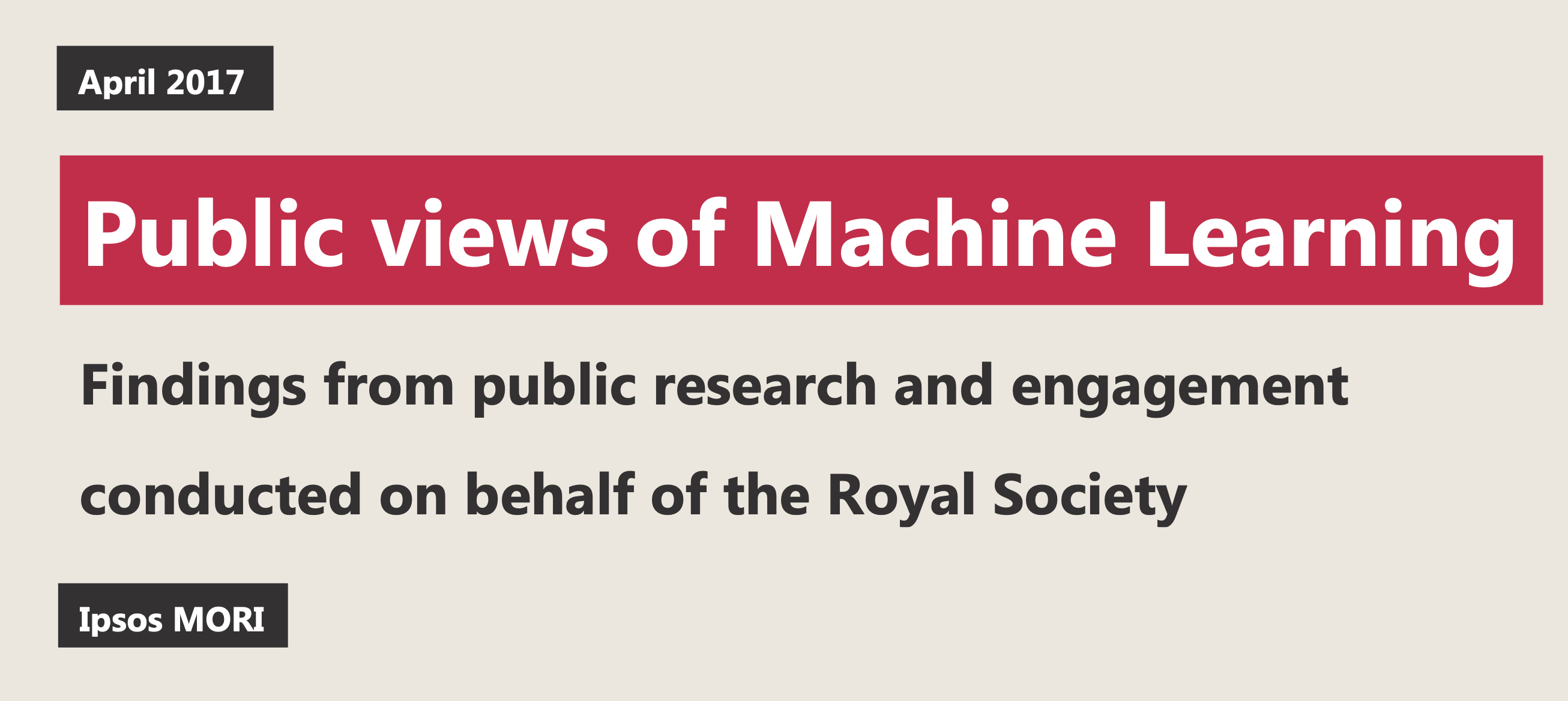
Figure: The Royal Society comissioned public research from Mori as part of the machine learning review.
As is often the cas when members of the public are well convened, they offered sensible opinions about the utility and challenges of machine learning methods.
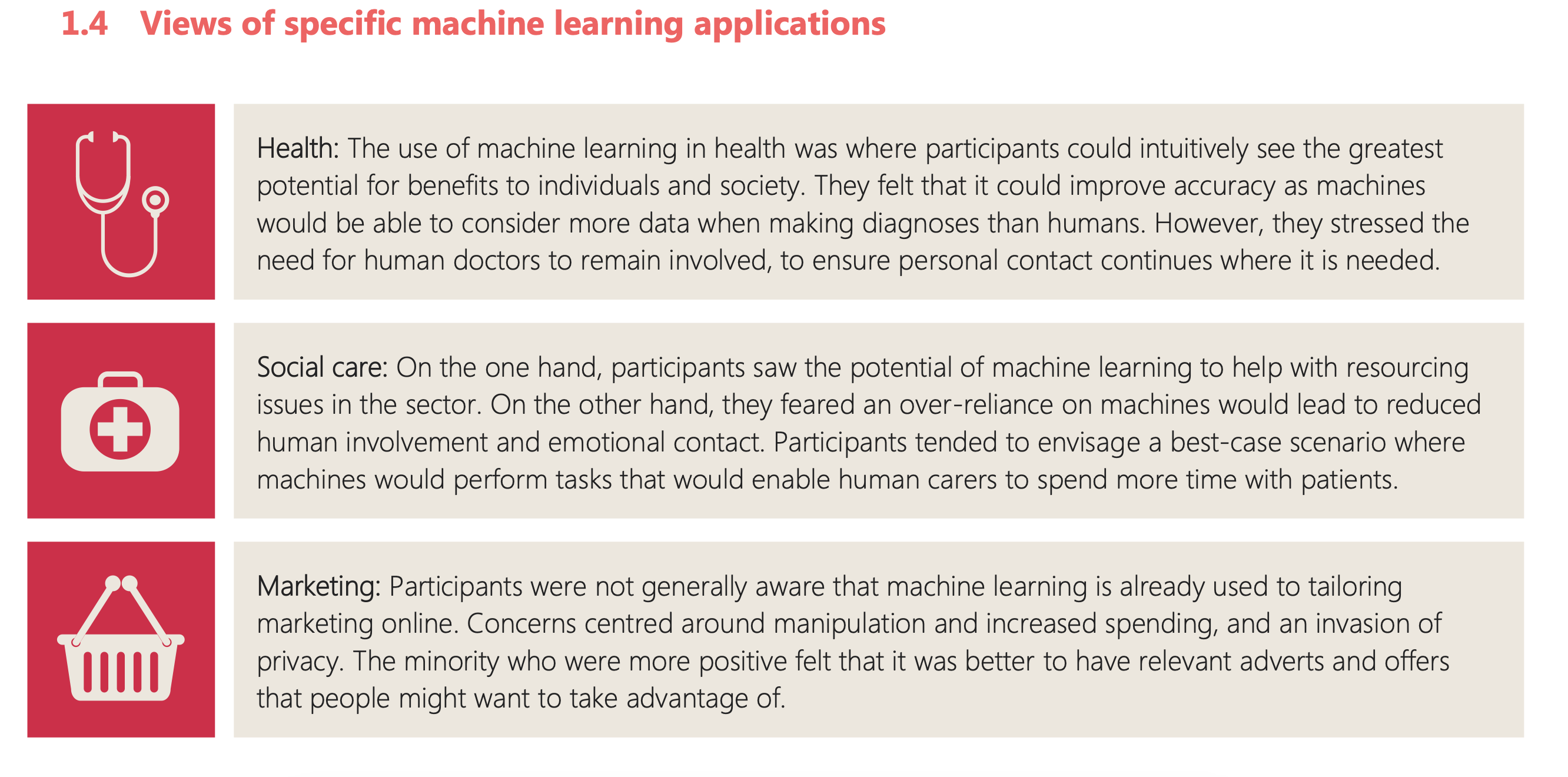
Figure: One of the questions focussed on machine learning applications.
A range of different scenaios were captured by this qualitative work.
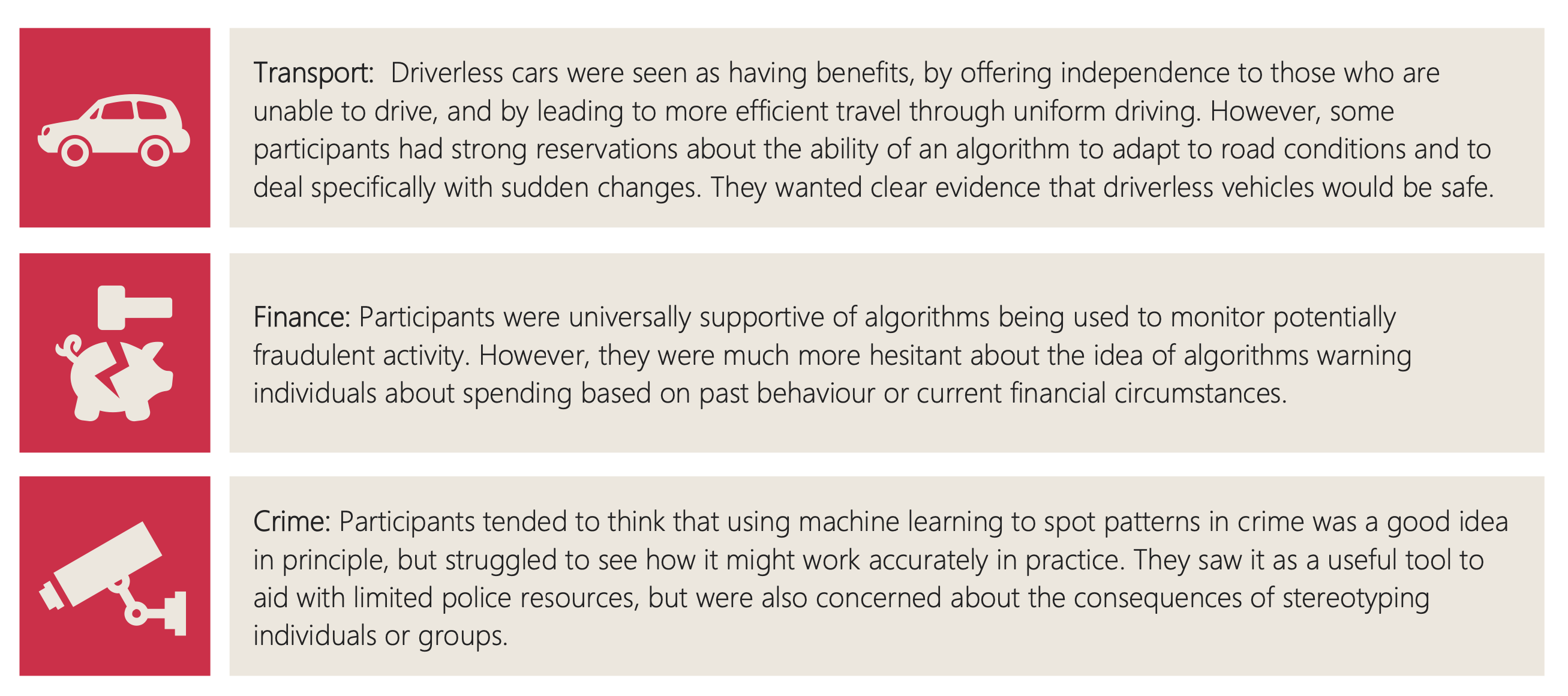
Figure: The public were broadly supportive of a range of application areas.
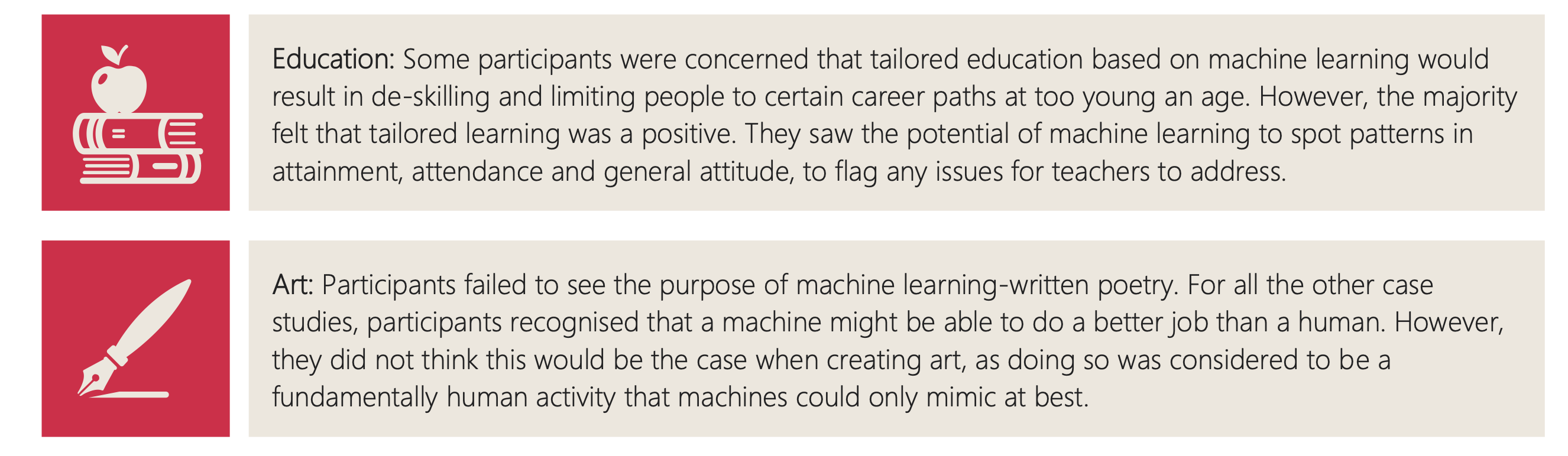
Figure: But they failed to see the point in AI’s that could produce poetry.
The one scenario where the public were unable to perceive any advantage in machine learning was an example from art, the creation of poetry. This was felt to be a fundamentally human activity that machines could only emulate at best.
Mercutio
It’s ironic that despite the public focus on transport, health, cities, crime and their confusion as to why we might want AI to do art, one area where significant progress has been made is on AIs that can create literature.
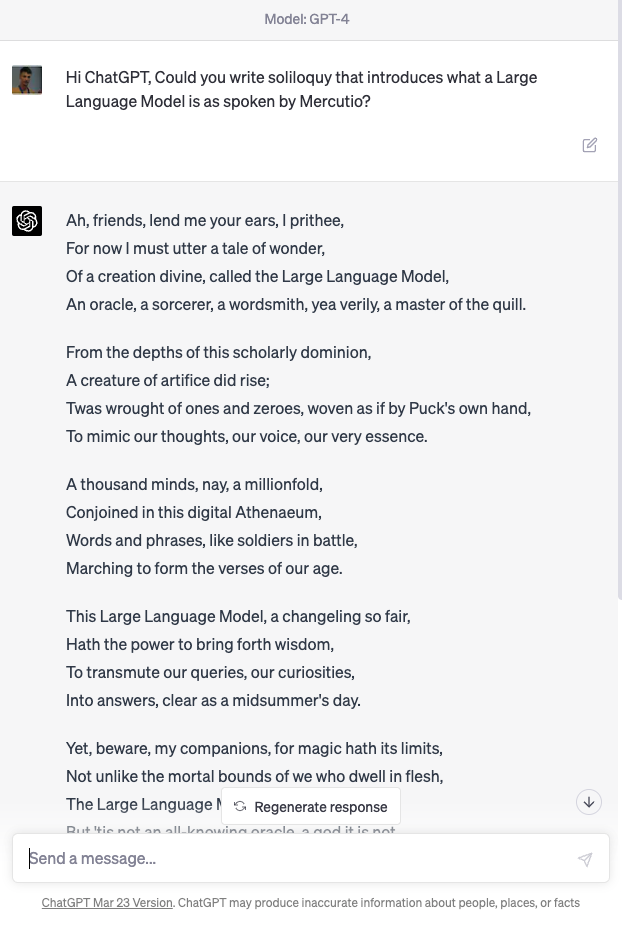
Figure: Despite the public’s desire to see challenges in health, finance, transport, crime solved by machine learning and their skepticism around poetry, one of the problems we have solved seems to be poetry.
User: Hi ChatGPT, Could you write soliloquy that introduces what a Large Language Model is as spoken by Mercutio?
Machine: Ah, friends, lend me your ears, I prithee, For now I must utter a tale of wonder, Of a creation divine, called the Large Language Model, An oracle, a sorcerer, a wordsmith, yea verily, a master of the quill.
From the depths of this scholarly dominion, A creature of artifice did rise; Twas wrought of ones and zeroes, woven as if by Puck’s own hand, To mimic our thoughts, our voice, our very essence.
A thousand minds, nay, a millionfold, Conjoined in this digital Athenaeum, Words and phrases, like soldiers in battle, Marching to form the verses of our age.
This Large Language Model, a changeling so fair, Hath the power to bring forth wisdom, To transmute our queries, our curiosities, Into answers, clear as a midsummer’s day.
Yet, beware, my companions, for magic hath its limits, Not unlike the mortal bounds of we who dwell in flesh, The Large Language Model hath its strengths, But ’tis not an all-knowing oracle, a god it is not.
As Mercutio, your humble servant, doth stand before thee, I marvel at the art and science that hath brought forth this wondrous creation, This Large Language Model, a testament to man’s yearning, To comprehend the world and make meaning of the great tapestry of existence.
In faith, dear friends, let us embrace the magic and beauty of this digital bard, And mayhaps, by its grace, we shall glean some wisdom, As we wander together, hand in hand, Through this boundless realm of knowledge and wonder.
ChatGPT transcript, 14th April 2023.
Public Dialogue on AI in Public Services
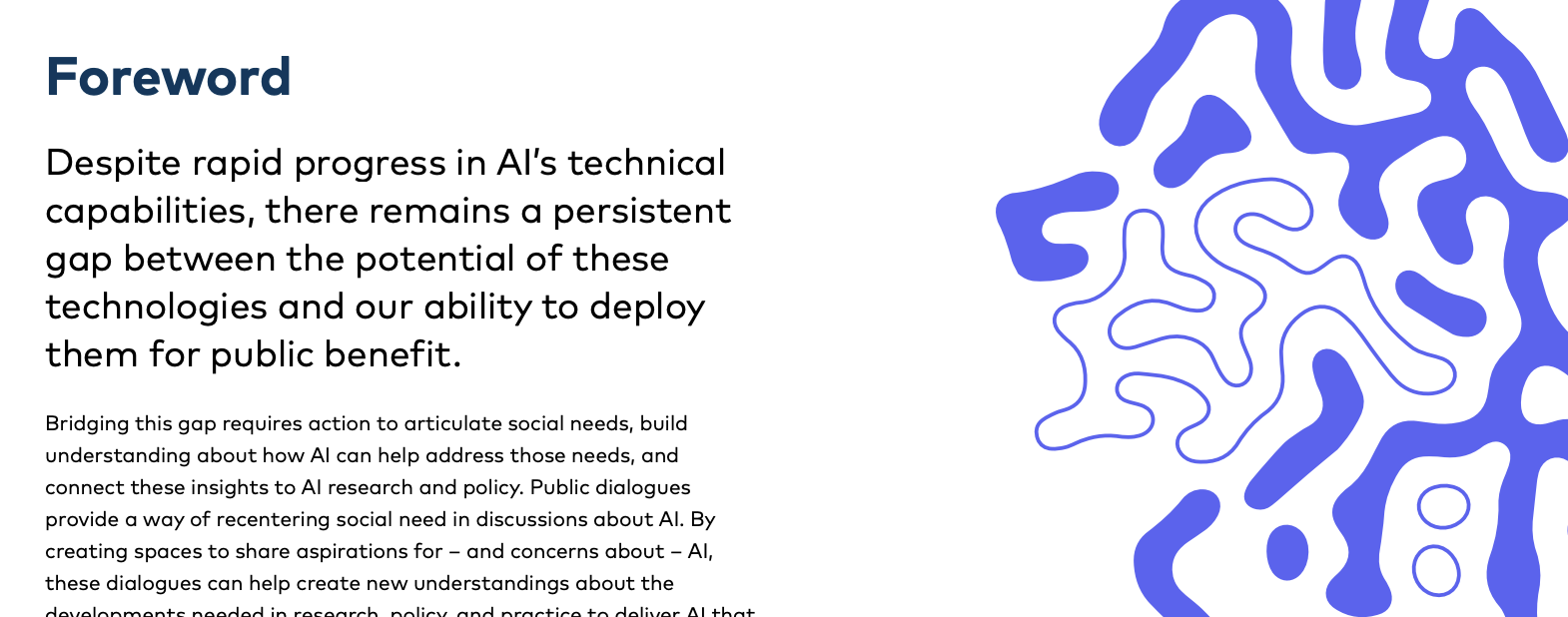
Figure: In September 2024, ai@cam convened a series of public dialogues to understand perspectives on the role of AI in delivering policy agendas.
In September 2024, ai@cam convened a series of public dialogues to understand perspectives on the role of AI in delivering priority policy agendas. Through workshops in Cambridge and Liverpool, 40 members of the public shared their views on how AI could support delivery of four key government missions around health, crime and policing, education, and energy and net zero.
The dialogue revealed a pragmatic public view that sees clear benefits from AI in reducing administrative burdens and improving service efficiency, while emphasizing the importance of maintaining human-centered services and establishing robust governance frameworks.
Key participant quotes illustrate this balanced perspective:
“It must be so difficult for GPs to keep track of how medication reacts with other medication on an individual basis. If there’s some database that shows all that, then it can only help, can’t it?”
Public Participant, Liverpool pg 10 ai@cam and Hopkins Van Mil (2024)
“I think a lot of the ideas need to be about AI being like a co-pilot to someone. I think it has to be that. So not taking the human away.”
Public Participant, Liverpool pg 15 ai@cam and Hopkins Van Mil (2024)
AI in Healthcare: Public Perspectives
In healthcare discussions, participants saw clear opportunities for AI to support NHS administration and improve service delivery, while expressing caution about AI involvement in direct patient care and diagnosis.
Key aspirations include:
- Reducing administrative burden on clinicians
- Supporting early diagnosis and prevention
- Improving research and drug development
- Better management of complex conditions
Illustrative quotes show the nuanced views:
“My wife [an NHS nurse] says that the paperwork side takes longer than the actual care.”
Public Participant, Liverpool pg 9 ai@cam and Hopkins Van Mil (2024)
“I wouldn’t just want to rely on the technology for something big like that, because obviously it’s a lifechanging situation.”
Public Participant, Cambridge pg 10 ai@cam and Hopkins Van Mil (2024)
Concerns focused particularly on maintaining human involvement in healthcare decisions and protecting patient privacy.
AI in Education: Public Perspectives
In education discussions, participants strongly supported AI’s potential to reduce teacher workload but expressed significant concerns about screen time and the importance of human interaction in learning.
A clear distinction emerged between support for AI in administrative tasks versus direct teaching roles. Participants emphasized that core aspects of education require human qualities that AI cannot replicate.
Key quotes illustrate these views:
“Education isn’t just about learning, it’s about preparing children for life, and you don’t do all of that in front of a screen.”
Public Participant, Cambridge ai@cam and Hopkins Van Mil (2024) pg 18
“Kids with ADHD or autism might prefer to interact with an iPad than they would a person, it could lighten the load for them.”
Public Participant, Liverpool ai@cam and Hopkins Van Mil (2024) pg 17
The dialogue revealed particular concern about the risk of AI increasing screen time and reducing social interaction, while acknowledging potential benefits for personalized learning support.
AI in Crime and Policing: Public Perspectives
Discussions around crime and policing revealed complex attitudes toward AI use, with support for analytical applications but strong concerns about surveillance and automated decision-making.
Participants emphasized that existing trust issues in policing need to be addressed before expanding AI use. They saw potential benefits in areas like crime pattern analysis but worried about bias and privacy implications.
Key quotes reflect these concerns:
“Trust in the police has been undermined by failures in vetting and appalling misconduct of some officers. I think AI can help this, because the fact is that we, as a society, we know how to compile information.”
Public Participant, Liverpool pg 14 ai@cam and Hopkins Van Mil (2024)
“I’m brown skinned and my mouth will move a bit more or I’m constantly fiddling with my foot… I’ve got ADHD. If facial recognition would see my brown skin, and then I’m moving differently to other people, will they see me as a terrorist?”
Public Participant, Liverpool pg 15 ai@cam and Hopkins Van Mil (2024)
The dialogue highlighted strong public desire for transparency and accountability in how AI is used in policing, with particular emphasis on preventing discriminatory outcomes.
AI in Energy and Net Zero: Public Perspectives
The energy and net zero discussions revealed support for AI’s potential to optimize energy systems while raising questions about access and affordability.
Participants saw clear benefits in using AI to improve energy efficiency and grid management, but worried about creating new inequalities through technology access barriers.
Representative quotes include:
“Everybody being able to generate on their roofs or in their gardens, selling energy from your car back to the grid, power being thrown different ways at different times. You’ve got to be resilient and independent.”
Public Participant, Cambridge pg 20 ai@cam and Hopkins Van Mil (2024)
“Is the infrastructure not a more important aspect than putting in AI systems? Government for years now has known that we need that infrastructure, but it’s always been someone else’s problem, the next government to sort out.”
Public Participant, Liverpool pg 21 ai@cam and Hopkins Van Mil (2024)
A key theme was ensuring AI deployment in energy systems doesn’t distract from fundamental changes needed to address climate change or exacerbate existing inequalities.
Summary
The public dialogue revealed several cross-cutting themes about how AI should be deployed in public services:
- AI should enhance rather than replace human capabilities
- Strong governance frameworks need to be in place before deployment
- Public engagement and transparency are essential
- Benefits must be distributed fairly across society
- Human-centered service delivery must be maintained
A powerful theme throughout the dialogue was the desire to maintain human connection and expertise while leveraging AI’s capabilities to improve service efficiency and effectiveness. As one participant noted:
“We need to look at the causes, we need to do some more thinking and not just start using AI to plaster over them [societal issues].”
Public Participant, Cambridge pg 13 ai@cam and Hopkins Van Mil (2024)
A Question of Trust
In Baroness Onora O’Neill’s Reeith Lectures from 2002, she raises the challenge of trust. There are many aspects to her arcuments, but one of the key points she makes is that we cannot trust without the notion of duty. O’Neill is bemoaning the substitution of duty with process. The idea is that processes and transparency are supposed to hold us to account by measuring outcomes. But these processes themselves overwhelm decision makers and undermine their professional duty to deliver the right outcome.
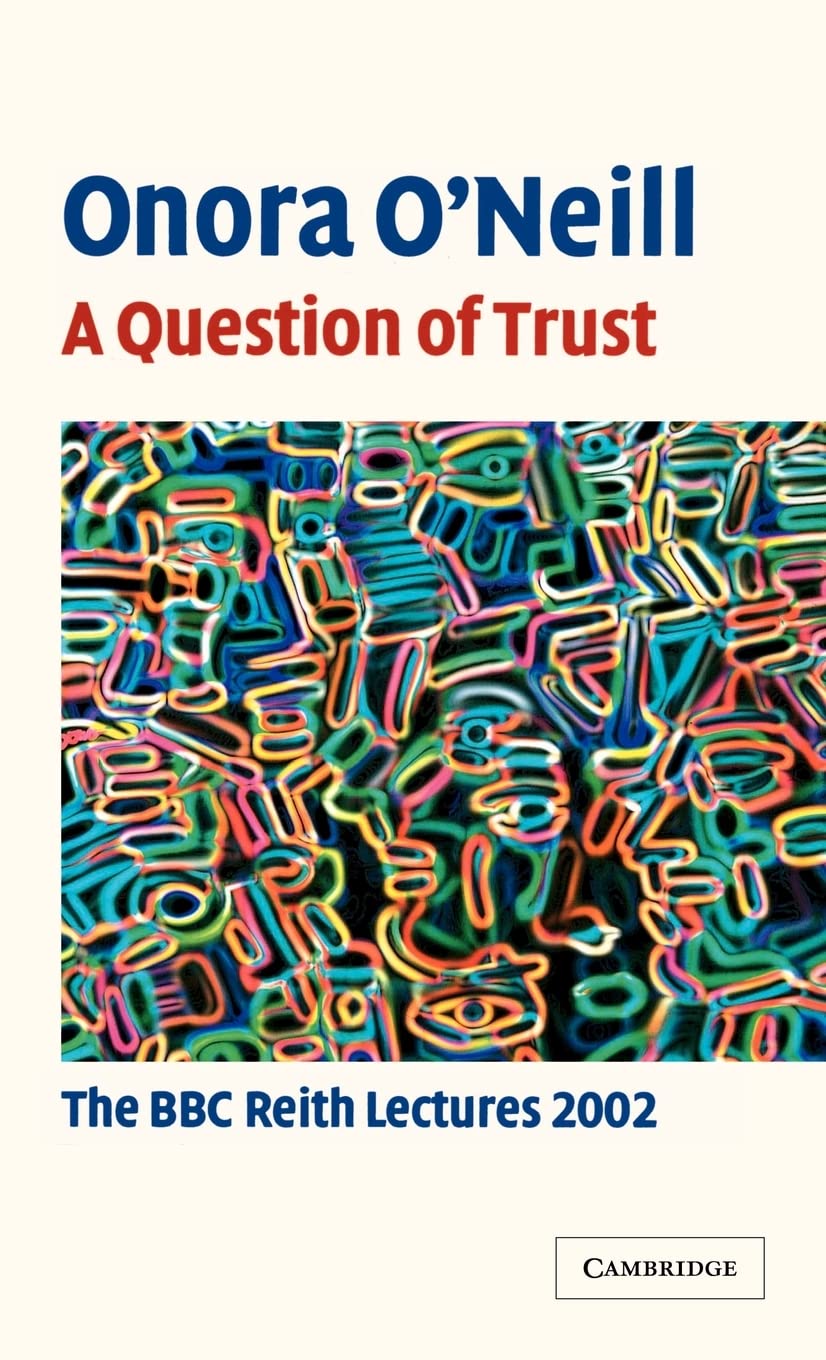
Figure: A Question of Trust by Onora O’Neil which examines the nature of trust and its role in society.
Again Univesities are to treat each applicant fairly on the basis of ability and promise, but they are supposed also to admit a socially more representative intake.
There’s no guarantee that the process meets the target.
Onora O’Neill A Question of Trust: Called to Account Reith Lectures 2002 O’Neill (2002)]
O’Neill is speaking in 2002, in the early days of the internet and before social media. Much of her thoughts are even more relevant for today than they were when she spoke. This is because the increased availability of information and machine driven decision-making makes the mistaken premise, that process is an adequate substitute for duty, more apparently plausible. But this undermines what O’Neill calls “intelligent accountability”, which is not accounting by the numbers, but through professional education and institutional safeguards.
See Lawrence (2024) O’Neill, Baroness Onora: ‘A question of trust’ lecture series (2002) p. 352, 363.
The Great AI Fallacy
There is a lot of variation in the use of the term artificial intelligence. I’m sometimes asked to define it, but depending on whether you’re speaking to a member of the public, a fellow machine learning researcher, or someone from the business community, the sense of the term differs.
However, underlying its use I’ve detected one disturbing trend. A trend I’m beginining to think of as “The Great AI Fallacy”.
The fallacy is associated with an implicit promise that is embedded in many statements about Artificial Intelligence. Artificial Intelligence, as it currently exists, is merely a form of automated decision making. The implicit promise of Artificial Intelligence is that it will be the first wave of automation where the machine adapts to the human, rather than the human adapting to the machine.
How else can we explain the suspension of sensible business judgment that is accompanying the hype surrounding AI?
This fallacy is particularly pernicious because there are serious benefits to society in deploying this new wave of data-driven automated decision making. But the AI Fallacy is causing us to suspend our calibrated skepticism that is needed to deploy these systems safely and efficiently.
The problem is compounded because many of the techniques that we’re speaking of were originally developed in academic laboratories in isolation from real-world deployment.
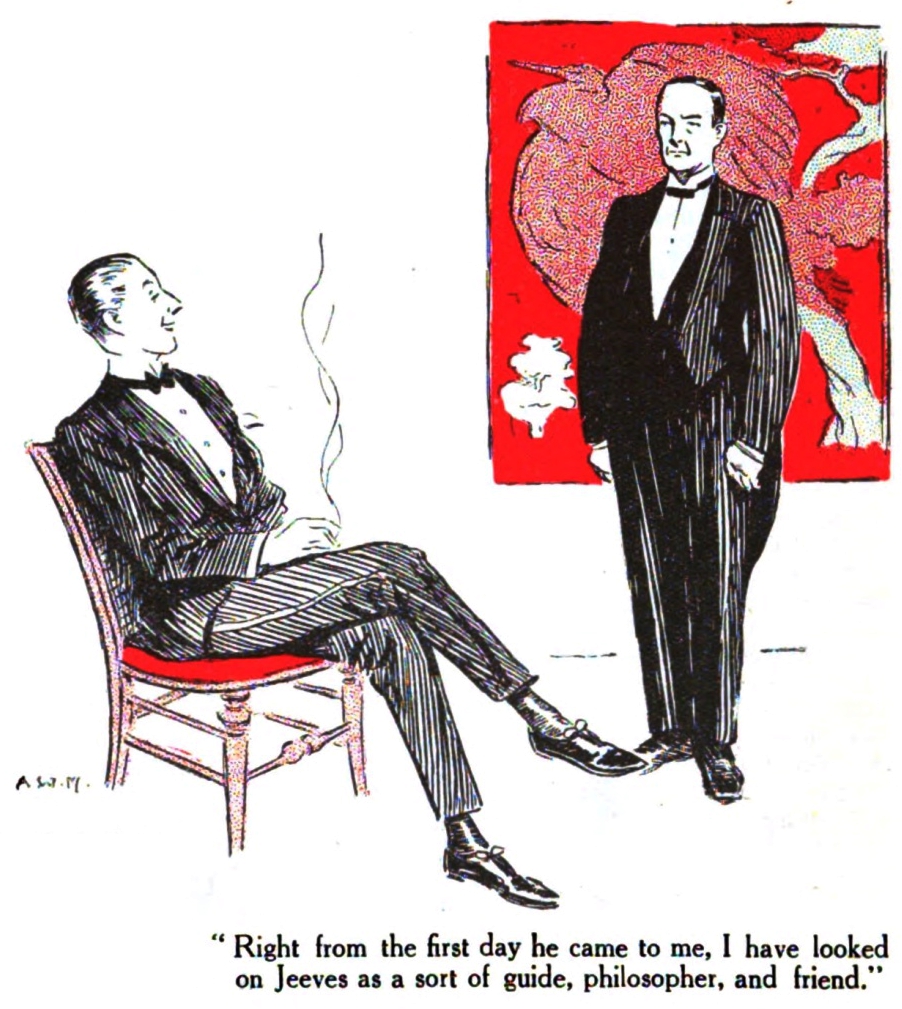
Figure: We seem to have fallen for a perspective on AI that suggests it will adapt to our schedule, rather in the manner of a 1930s manservant.
Digital Failure Examples
The Horizon Scandal
In the UK we saw these effects play out in the Horizon scandal: the accounting system of the national postal service was computerized by Fujitsu and first installed in 1999, but neither the Post Office nor Fujitsu were able to control the system they had deployed. When it went wrong individual sub postmasters were blamed for the systems’ errors. Over the next two decades they were prosecuted and jailed leaving lives ruined in the wake of the machine’s mistakes.
See Lawrence (2024) Horizon scandal p. 371.
The Lorenzo Scandal
The Lorenzo scandal is the National Programme for IT which was intended to allow the NHS to move towards electronic health records.
The oral transcript can be found at https://publications.parliament.uk/pa/cm201012/cmselect/cmpubacc/1070/11052302.htm.
One quote from 16:54:33 in the committee discussion captures the top-down nature of the project.
Q117 Austin Mitchell: You said, Sir David, the problems came from the middle range, but surely they were implicit from the start, because this project was rushed into. The Prime Minister [Tony Blair] was very keen, the delivery unit was very keen, it was very fashionable to computerise things like this. An appendix indicating the cost would be £5 billion was missed out of the original report as published, so you have a very high estimate there in the first place. Then, Richard Granger, the Director of IT, rushed through, without consulting the professions. This was a kind of computer enthusiast’s bit, was it not? The professionals who were going to have to work it were not consulted, because consultation would have made it clear that they were going to ask more from it and expect more from it, and then contracts for £1 billion were let pretty well straightaway, in May 2003. That was very quick. Now, why were the contracts let before the professionals were consulted?
An analysis of the problems was published by Justinia (2017). Based on the paper, the key challenges faced in the UK’s National Programme for IT (NPfIT) included:
Lack of adequate end user engagement, particularly with frontline healthcare staff and patients. The program was imposed from the top-down without securing buy-in from stakeholders.
Absence of a phased change management approach. The implementation was rushed without proper planning for organizational and cultural changes.
Underestimating the scale and complexity of the project. The centralized, large-scale approach was overambitious and difficult to manage.
Poor project management, including unrealistic timetables, lack of clear leadership, and no exit strategy.
Insufficient attention to privacy and security concerns regarding patient data.
Lack of local ownership. The centralized approach meant local healthcare providers felt no ownership over the systems.
Communication issues, including poor communication with frontline staff about the program’s benefits.
Technical problems, delays in delivery, and unreliable software.
Failure to recognize the socio-cultural challenges were as significant as the technical ones.
Lack of flexibility to adapt to changing requirements over the long timescale.
Insufficient resources and inadequate methodologies for implementation.
Low morale among NHS staff responsible for implementation due to uncertainties and unrealistic timetables.
Conflicts between political objectives and practical implementation needs.
The paper emphasizes that while technical competence is necessary, the organizational, human, and change management factors were more critical to the program’s failure than purely technological issues. The top-down, centralized approach and lack of stakeholder engagement were particularly problematic.
Reports at the Time
Report https://publications.parliament.uk/pa/cm201012/cmselect/cmpubacc/1070/1070.pdf
Commonalities
Key points: Both cases demonstrate a significant gap between high-level policy decisions (macro) and on-the-ground realities (micro).
- The failures stemmed from insufficient consideration of local needs, capabilities, and existing systems.
- There was a lack of effective feedback mechanisms from the micro to macro level.
- The implementations suffered from overly rigid, top-down approaches that didn’t allow for local adaptation.
- In both cases, there was insufficient engagement with end-users and local stakeholders.
Complexity in Action
As an exercise in understanding complexity, watch the following video. You will see the basketball being bounced around, and the players moving. Your job is to count the passes of those dressed in white and ignore those of the individuals dressed in black.
Figure: Daniel Simon’s famous illusion “monkey business”. Focus on the movement of the ball distracts the viewer from seeing other aspects of the image.
In a classic study Simons and Chabris (1999) ask subjects to count the number of passes of the basketball between players on the team wearing white shirts. Fifty percent of the time, these subjects don’t notice the gorilla moving across the scene.
The phenomenon of inattentional blindness is well known, e.g in their paper Simons and Charbris quote the Hungarian neurologist, Rezsö Bálint,
It is a well-known phenomenon that we do not notice anything happening in our surroundings while being absorbed in the inspection of something; focusing our attention on a certain object may happen to such an extent that we cannot perceive other objects placed in the peripheral parts of our visual field, although the light rays they emit arrive completely at the visual sphere of the cerebral cortex.
Rezsö Bálint 1907 (translated in Husain and Stein 1988, page 91)
When we combine the complexity of the world with our relatively low bandwidth for information, problems can arise. Our focus on what we perceive to be the most important problem can cause us to miss other (potentially vital) contextual information.
This phenomenon is known as selective attention or ‘inattentional blindness’.
Figure: For a longer talk on inattentional bias from Daniel Simons see this video.
Data Selective Attention Bias
We are going to see how inattention biases can play out in data analysis by going through a simple example. The analysis involves body mass index and activity information.
BMI Steps Data
The BMI Steps example is taken from Yanai and Lercher (2020). We are given a data set of body-mass index measurements against step counts. For convenience we have packaged the data so that it can be easily downloaded.
import pods
= pods.datasets.bmi_steps()
data = data['X']
X = data['Y'] y
It is good practice to give our variables interpretable names so that
the analysis may be clearly understood by others. Here the
steps
count is the first dimension of the covariate, the
bmi
is the second dimension and the gender
is
stored in y
with 1
for female and
0
for male.
= X[:, 0]
steps = X[:, 1]
bmi = y[:, 0] gender
We can check the mean steps and the mean of the BMI.
print('Steps mean is {mean}.'.format(mean=steps.mean()))
print('BMI mean is {mean}.'.format(mean=bmi.mean()))
BMI Steps Data Analysis
We can also separate out the means from the male and female populations. In python this can be done by setting male and female indices as follows.
= (gender==0)
male_ind = (gender==1) female_ind
And now we can extract the variables for the two populations.
= steps[male_ind]
male_steps = bmi[male_ind] male_bmi
And as before we compute the mean.
print('Male steps mean is {mean}.'.format(mean=male_steps.mean()))
print('Male BMI mean is {mean}.'.format(mean=male_bmi.mean()))
Similarly, we can get the same result for the female portion of the populaton.
= steps[female_ind]
female_steps = bmi[female_ind] female_bmi
print('Female steps mean is {mean}.'.format(mean=female_steps.mean()))
print('Female BMI mean is {mean}.'.format(mean=female_bmi.mean()))
Interesting, the female BMI average is slightly higher than the male BMI average. The number of steps in the male group is higher than that in the female group. Perhaps the steps and the BMI are anti-correlated. The more steps, the lower the BMI.
Python provides a statistics package. We’ll import this in
python
so that we can try and understand the correlation
between the steps
and the BMI
.
from scipy.stats import pearsonr
= pearsonr(steps, bmi)
corr, _ print("Pearson's overall correlation: {corr}".format(corr=corr))
= pearsonr(male_steps, male_bmi)
male_corr, _ print("Pearson's correlation for males: {corr}".format(corr=male_corr))
= pearsonr(female_steps, female_bmi)
female_corr, _ print("Pearson's correlation for females: {corr}".format(corr=female_corr))
A Hypothesis as a Liability
This analysis is from an article titled “A Hypothesis as a Liability” (Yanai and Lercher, 2020), they start their article with the following quite from Herman Hesse.
“ ‘When someone seeks,’ said Siddhartha, ‘then it easily happens that his eyes see only the thing that he seeks, and he is able to find nothing, to take in nothing. […] Seeking means: having a goal. But finding means: being free, being open, having no goal.’ ”
Hermann Hesse
Their idea is that having a hypothesis can constrain our thinking. However, in answer to their paper Felin et al. (2021) argue that some form of hypothesis is always necessary, suggesting that a hypothesis can be a liability
My view is captured in the introductory chapter to an edited volume on computational systems biology that I worked on with Mark Girolami, Magnus Rattray and Guido Sanguinetti.
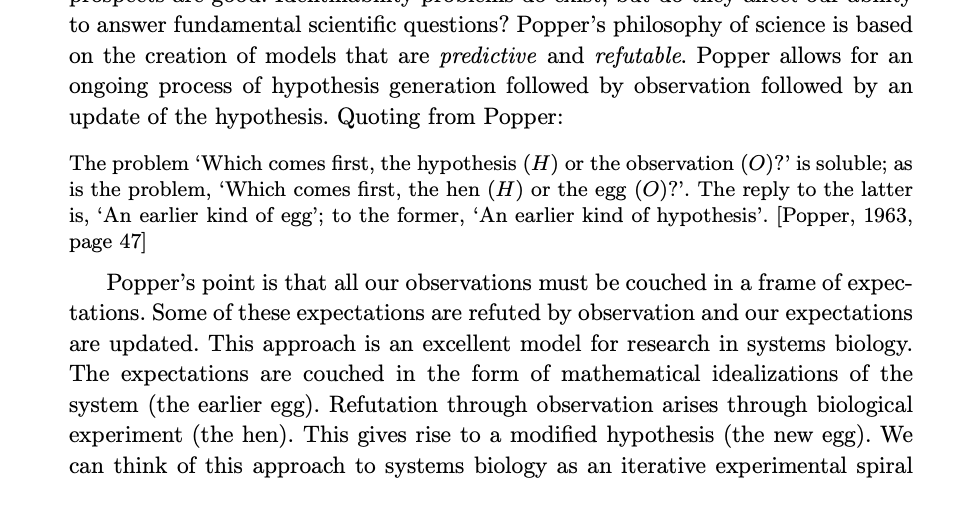
Figure: Quote from Lawrence (2010) highlighting the importance of interaction between data and hypothesis.
Popper nicely captures the interaction between hypothesis and data by relating it to the chicken and the egg. The important thing is that these two co-evolve.
Number Theatre
Unfortunately, we don’t always have time to wait for this process to converge to an answer we can all rely on before a decision is required.
Not only can we be misled by data before a decision is made, but sometimes we can be misled by data to justify the making of a decision. David Spiegelhalter refers to the phenomenon of “Number Theatre” in a conversation with Andrew Marr from May 2020 on the presentation of data.
Figure: Professor Sir David Spiegelhalter on Andrew Marr on 10th May 2020 speaking about some of the challengers around data, data presentation, and decision making in a pandemic. David mentions number theatre at 9 minutes 10 seconds.
Data Theatre
Data Theatre exploits data inattention bias to present a particular view on events that may misrepresents through selective presentation. Statisticians are one of the few groups that are trained with a sufficient degree of data skepticism. But it can also be combatted through ensuring there are domain experts present, and that they can speak freely.
Figure: The phenomenon of number theatre or data theatre was described by David Spiegelhalter and is nicely summarized by Martin Robbins in this sub-stack article https://martinrobbins.substack.com/p/data-theatre-why-the-digital-dashboards.
Sir David Spiegelhalter
The statistician’s craft is based on humility in front of data and developing the appropriate skeptical thinking around conclusions from data. The best individual I’ve seen at conveying and developing that sense is Sir David Spiegelhalter.
The Art of Statistics
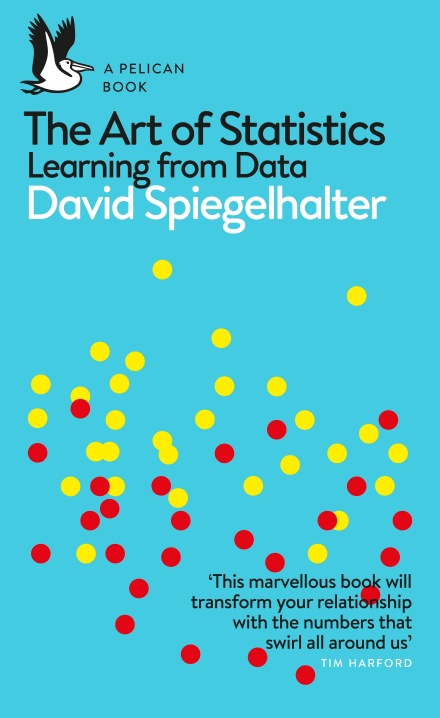
Figure: The Art of Statistics by David Spiegelhalter is an excellent read on the pitfalls of data interpretation.
The Art of Statistics (Spiegelhalter, 2019) brings important examples from statistics to life in an intelligent and entertaining way. It is highly readable and gives an opportunity to fast-track towards the important skill of data-skepticism that is the mark of a professional statistician.
The Art of Uncertainty
David has also released a new book that focusses on Uncertainty.
See (Spiegelhalter, 2024)
Blake’s Newton
William Blake’s rendering of Newton captures humans in a particular state. He is trance-like absorbed in simple geometric shapes. The feel of dreams is enhanced by the underwater location, and the nature of the focus is enhanced because he ignores the complexity of the sea life around him.
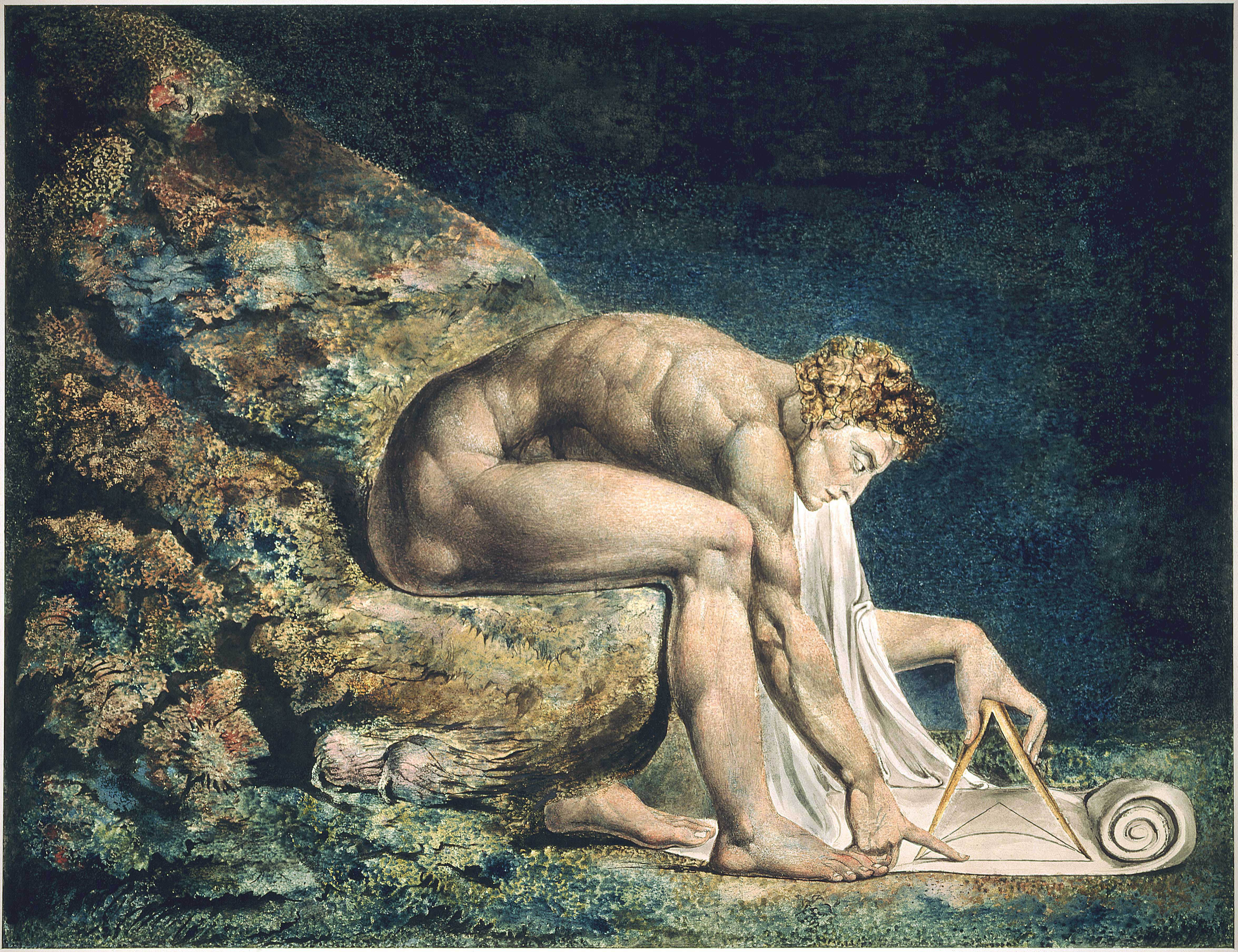
Figure: William Blake’s Newton. 1795c-1805
See Lawrence (2024) Blake, William Newton p. 121–123.
The caption in the Tate Britain reads:
Here, Blake satirises the 17th-century mathematician Isaac Newton. Portrayed as a muscular youth, Newton seems to be underwater, sitting on a rock covered with colourful coral and lichen. He crouches over a diagram, measuring it with a compass. Blake believed that Newton’s scientific approach to the world was too reductive. Here he implies Newton is so fixated on his calculations that he is blind to the world around him. This is one of only 12 large colour prints Blake made. He seems to have used an experimental hybrid of printing, drawing, and painting.
From the Tate Britain
See Lawrence (2024) Blake, William Newton p. 121–123, 258, 260, 283, 284, 301, 306.
The MONIAC
The MONIAC was an analogue computer designed to simulate the UK economy. Analogue comptuers work through analogy, the analogy in the MONIAC is that both money and water flow. The MONIAC exploits this through a system of tanks, pipes, valves and floats that represent the flow of money through the UK economy. Water flowed from the treasury tank at the top of the model to other tanks representing government spending, such as health and education. The machine was initially designed for teaching support but was also found to be a useful economic simulator. Several were built and today you can see the original at Leeds Business School, there is also one in the London Science Museum and one in the Unisversity of Cambridge’s economics faculty.
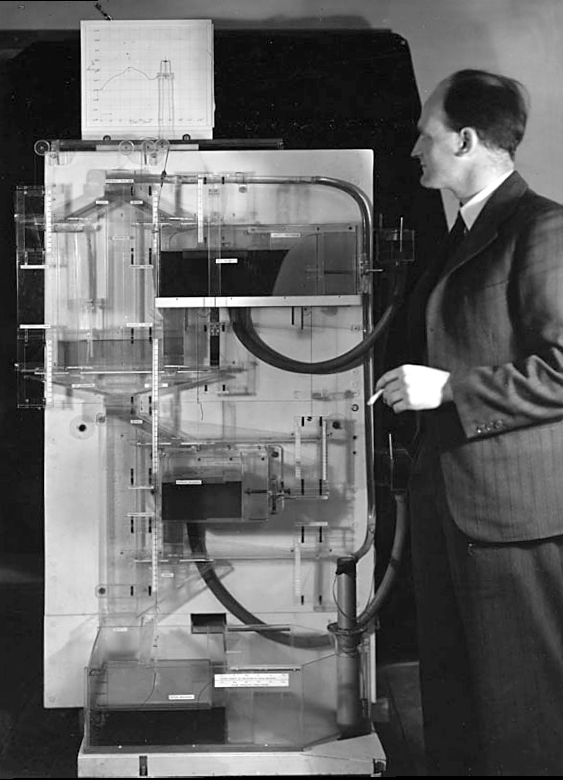
Figure: Bill Phillips and his MONIAC (completed in 1949). The machine is an analogue computer designed to simulate the workings of the UK economy.
See Lawrence (2024) MONIAC p. 232-233, 266, 343.
Human Analogue Machine
Recent breakthroughs in generative models, particularly large language models, have enabled machines that, for the first time, can converse plausibly with other humans.
The Apollo guidance computer provided Armstrong with an analogy when he landed it on the Moon. He controlled it through a stick which provided him with an analogy. The analogy is based in the experience that Amelia Earhart had when she flew her plane. Armstrong’s control exploited his experience as a test pilot flying planes that had control columns which were directly connected to their control surfaces.
Figure: The human analogue machine is the new interface that large language models have enabled the human to present. It has the capabilities of the computer in terms of communication, but it appears to present a “human face” to the user in terms of its ability to communicate on our terms. (Image quite obviously not drawn by generative AI!)
The generative systems we have produced do not provide us with the “AI” of science fiction. Because their intelligence is based on emulating human knowledge. Through being forced to reproduce our literature and our art they have developed aspects which are analogous to the cultural proxy truths we use to describe our world.
These machines are to humans what the MONIAC was the British economy. Not a replacement, but an analogue computer that captures some aspects of humanity while providing advantages of high bandwidth of the machine.
HAM
The Human-Analogue Machine or HAM therefore provides a route through which we could better understand our world through improving the way we interact with machines.
Figure: The trinity of human, data, and computer, and highlights the modern phenomenon. The communication channel between computer and data now has an extremely high bandwidth. The channel between human and computer and the channel between data and human is narrow. New direction of information flow, information is reaching us mediated by the computer. The focus on classical statistics reflected the importance of the direct communication between human and data. The modern challenges of data science emerge when that relationship is being mediated by the machine.
The HAM can provide an interface between the digital computer and the human allowing humans to work closely with computers regardless of their understandin gf the more technical parts of software engineering.
Figure: The HAM now sits between us and the traditional digital computer.
Of course this route provides new routes for manipulation, new ways in which the machine can undermine our autonomy or exploit our cognitive foibles. The major challenge we face is steering between these worlds where we gain the advantage of the computer’s bandwidth without undermining our culture and individual autonomy.
See Lawrence (2024) human-analogue machine (HAMs) p. 343-347, 359-359, 365-368.
Conclusion
See the Gorilla don’t be the Gorilla.
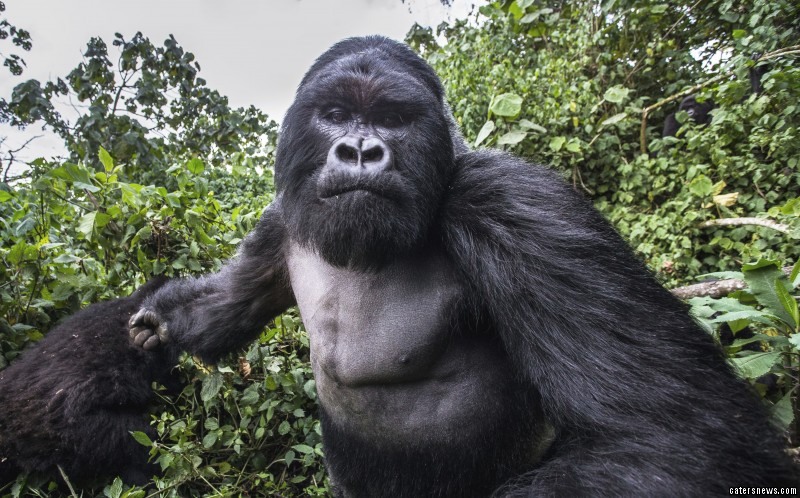
Figure: A famous quote from Mike Tyson before his fight with Evander Holyfield: “Everyone has a plan until they get punched in the mouth”. Don’t let the gorilla punch you in the mouth. See the gorilla, but don’t be the gorilla. Photo credit: https://www.catersnews.com/stories/animals/go-ape-unlucky-photographer-gets-punched-by-lairy-gorilla-drunk-from-eating-bamboo-shoots/
See Lawrence (2024) Tyson, Mike p. 92–93, 130, 193, 217, 225, 328, 348.
Thanks!
For more information on these subjects and more you might want to check the following resources.
- book: The Atomic Human
- twitter: @lawrennd
- podcast: The Talking Machines
- newspaper: Guardian Profile Page
- blog: http://inverseprobability.com