Achievement and the Atomic Human
Abstract
In this talk Neil will reflect on the political themes that arose in the book, the atomic human, where the challenges arise from and where the solutions might lie.
Broadly speaking we face challenges in the modern information topography from both corporate and government entities, the answers may lie in an improved form of democractic institutionalism but this in turn implies that the individuals that make up our society from citizens to professionals are empowered rather than disempowered by the technology.
Reminder: Essence of Human Intelligence
Bandwidth Constrained Conversations
Figure: Conversation relies on internal models of other individuals.
Figure: Misunderstanding of context and who we are talking to leads to arguments.
Embodiment factors imply that, in our communication between humans, what is not said is, perhaps, more important than what is said. To communicate with each other we need to have a model of who each of us are.
To aid this, in society, we are required to perform roles. Whether as a parent, a teacher, an employee or a boss. Each of these roles requires that we conform to certain standards of behaviour to facilitate communication between ourselves.
Control of self is vitally important to these communications.
The high availability of data available to humans undermines human-to-human communication channels by providing new routes to undermining our control of self.
The consequences between this mismatch of power and delivery are to be seen all around us. Because, just as driving an F1 car with bicycle wheels would be a fine art, so is the process of communication between humans.
If I have a thought and I wish to communicate it, I first need to have a model of what you think. I should think before I speak. When I speak, you may react. You have a model of who I am and what I was trying to say, and why I chose to say what I said. Now we begin this dance, where we are each trying to better understand each other and what we are saying. When it works, it is beautiful, but when mis-deployed, just like a badly driven F1 car, there is a horrible crash, an argument.
New Flow of Information
Classically the field of statistics focused on mediating the relationship between the machine and the human. Our limited bandwidth of communication means we tend to over-interpret the limited information that we are given, in the extreme we assign motives and desires to inanimate objects (a process known as anthropomorphizing). Much of mathematical statistics was developed to help temper this tendency and understand when we are valid in drawing conclusions from data.
Figure: The trinity of human, data, and computer, and highlights the modern phenomenon. The communication channel between computer and data now has an extremely high bandwidth. The channel between human and computer and the channel between data and human is narrow. New direction of information flow, information is reaching us mediated by the computer. The focus on classical statistics reflected the importance of the direct communication between human and data. The modern challenges of data science emerge when that relationship is being mediated by the machine.
Data science brings new challenges. In particular, there is a very large bandwidth connection between the machine and data. This means that our relationship with data is now commonly being mediated by the machine. Whether this is in the acquisition of new data, which now happens by happenstance rather than with purpose, or the interpretation of that data where we are increasingly relying on machines to summarize what the data contains. This is leading to the emerging field of data science, which must not only deal with the same challenges that mathematical statistics faced in tempering our tendency to over interpret data but must also deal with the possibility that the machine has either inadvertently or maliciously misrepresented the underlying data.
Importance of Narratives
A Six Word Novel
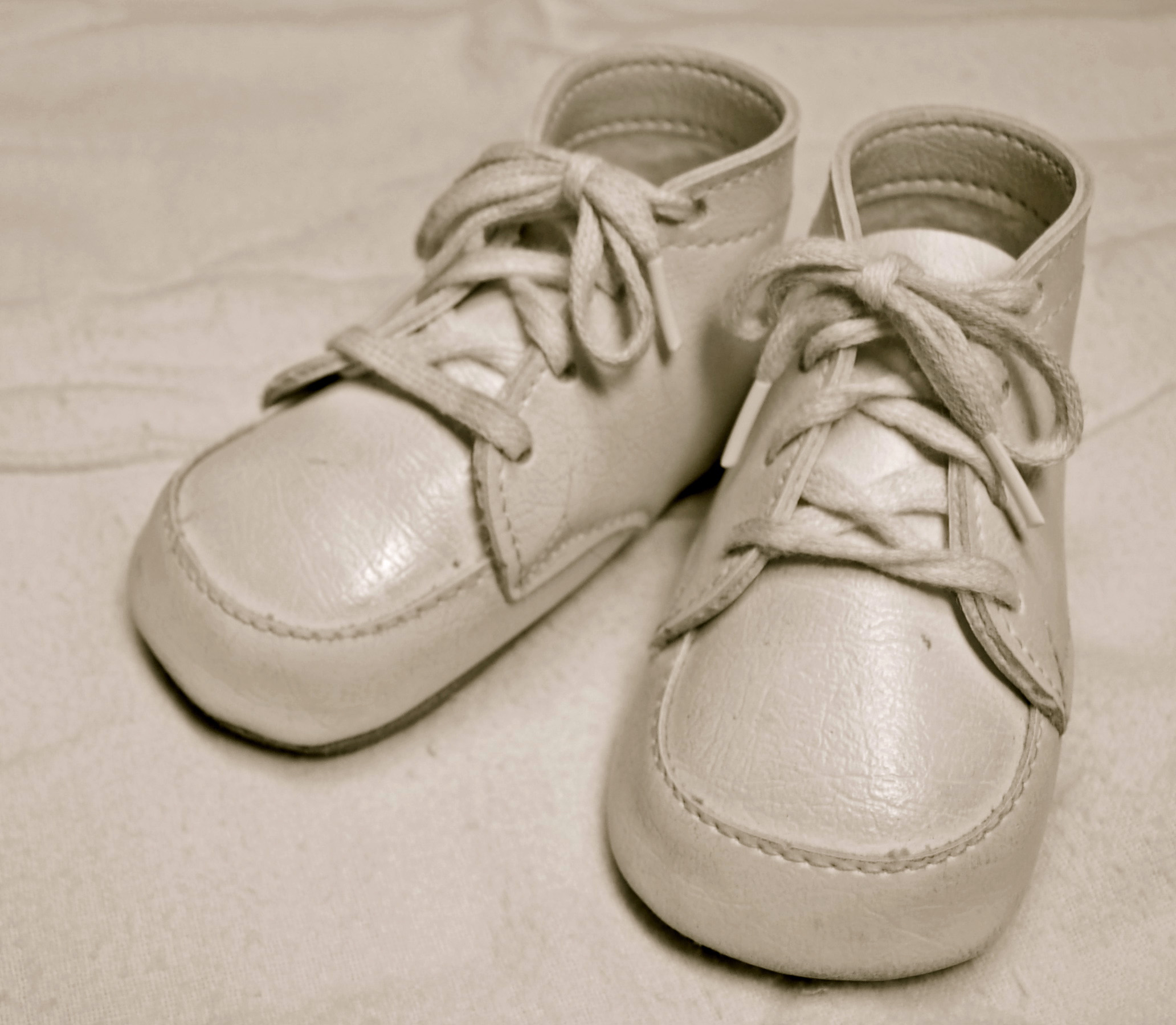
Figure: Consider the six-word novel, apocryphally credited to Ernest Hemingway, “For sale: baby shoes, never worn”. To understand what that means to a human, you need a great deal of additional context. Context that is not directly accessible to a machine that has not got both the evolved and contextual understanding of our own condition to realize both the implication of the advert and what that implication means emotionally to the previous owner.
See Lawrence (2024) baby shoes p. 368.
But this is a very different kind of intelligence than ours. A computer cannot understand the depth of the Ernest Hemingway’s apocryphal six-word novel: “For Sale, Baby Shoes, Never worn”, because it isn’t equipped with that ability to model the complexity of humanity that underlies that statement.
The Attention Economy
Herbert Simon on Information
What information consumes is rather obvious: it consumes the attention of its recipients. Hence a wealth of information creates a poverty of attention …
Simon (1971)
The attention economy was a phenomenon described in 1971 by the American computer scientist Herbert Simon. He saw the coming information revolution and wrote that a wealth of information would create a poverty of attention. Too much information means that human attention becomes the scarce resource, the bottleneck. It becomes the gold in the attention economy.
The power associated with control of information dates back to the invention of writing. By pressing reeds into clay tablets Sumerian scribes stored information and controlled the flow of information.
Revolution
Arguably the information revolution we are experiencing is unprecedented in history. But changes in the way we share information have a long history. Over 5,000 years ago in the city of Uruk, on the banks of the Euphrates, communities which relied on the water to irrigate their corps developed an approach to recording transactions in clay. Eventually the system of recording system became sophisticated enough that their oral histories could be recorded in the form of the first epic: Gilgamesh.
See Lawrence (2024) cuneiform p. 337, 360, 390.
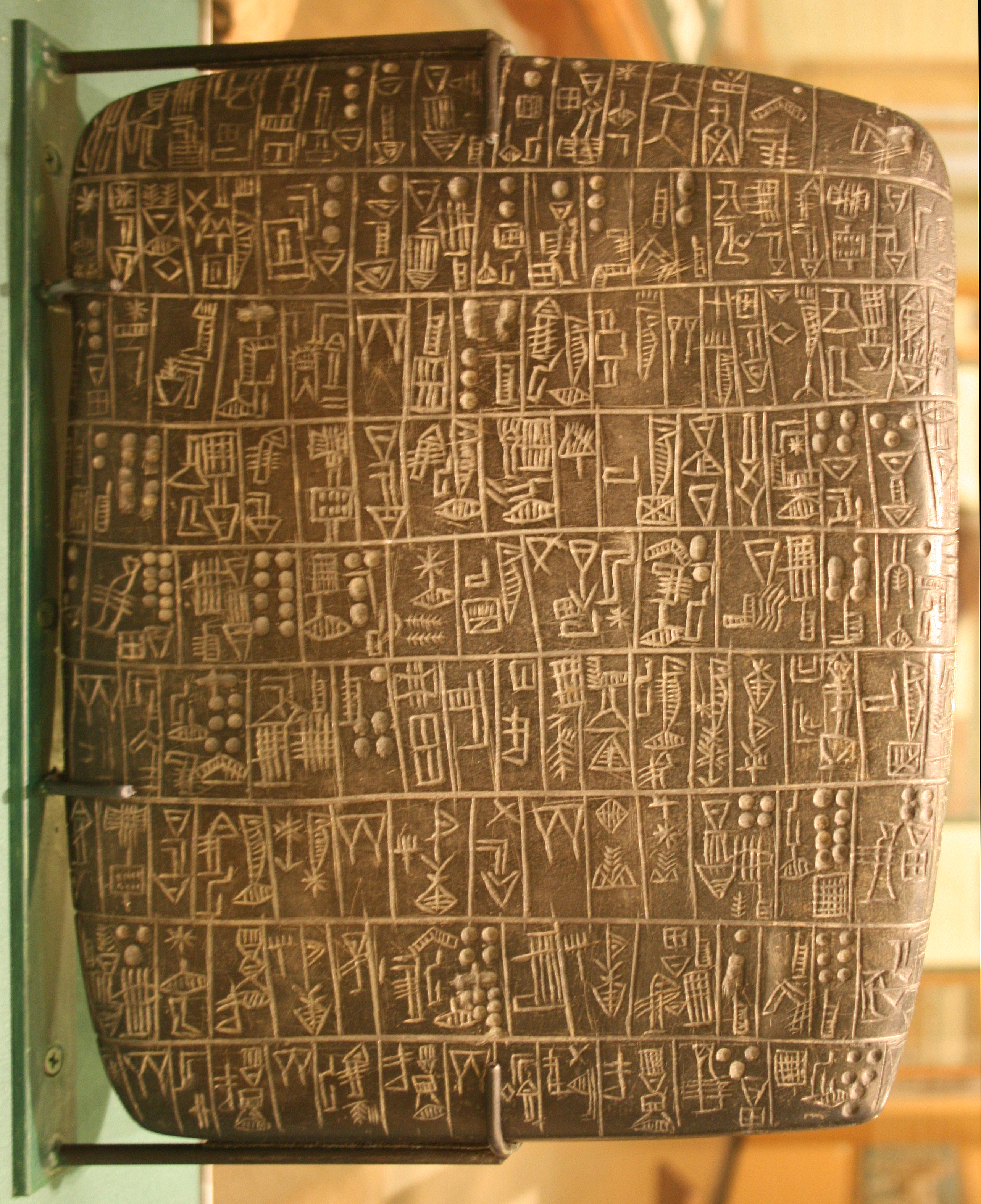
Figure: Chicago Stone, side 2, recording sale of a number of fields, probably from Isin, Early Dynastic Period, c. 2600 BC, black basalt
It was initially developed for people as a record of who owed what to whom, expanding individuals’ capacity to remember. But over a five hundred year period writing evolved to become a tool for literature as well. More pithily put, writing was invented by accountants not poets (see e.g. this piece by Tim Harford).
In some respects today’s revolution is different, because it involves also the creation of stories as well as their curation. But in some fundamental ways we can see what we have produced as another tool for us in the information revolution.
The Open Society and its Enemies
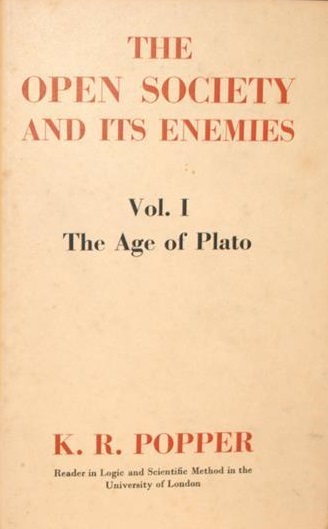
Figure: The Open Society and Its Enemies by Karl Popper views liberal democracies as a collection of “piecemeal social engineers” who strive towards better outcomes.
Popper opened the preface to his book (Popper, 1945) with the following words:
If in this book harsh words are spoken about some of the greatest among the intellectual leaders of mankind, my motive is not, I hope, to belittle them. It springs rather from my conviction that, if our civilization is to survive, we must break with the habit of deference to great men. Great men may make great mistakes; and as the book tries to show, some of the greatest leaders of the past supported the perennial attack on freedom and reason.
He had written the book against the background of the second world war, his decision to write it taken on the day the Nazis invaded Austria in March 1938. His book is a reaction to totalitarianism.
For Popper, the ideas of “great men” become totalitarian when imposed on society. He advocates for direct liberal democracy as the only form of government that can allow for institutional change without bloodshed. The open society is one characterized by institutions and individuals that can engage in the practical pursuit of solutions to social and political problems. The institutions are also underpinned by individuals: lawyers, accountants, civil administrators and many more. To Popper it is these “piecemeal social engineers” who offer pragmatic solutions to our society’s political and social challenges.
See Lawrence (2024) Popper, Karl The Open Society and its Enemies p. 371–374.
Two Types of Stochastic Parrot
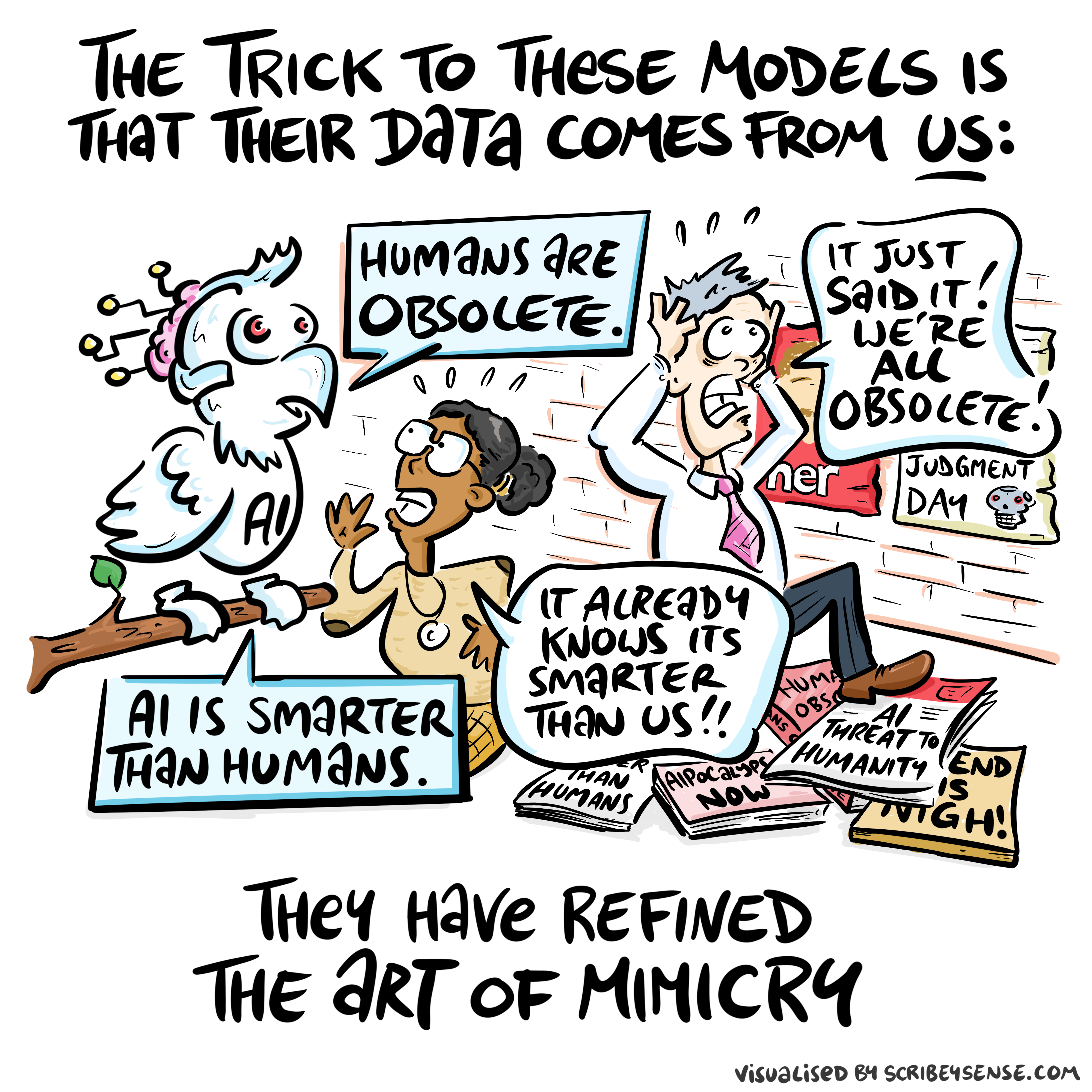
Figure: This is the drawing Dan was inspired to create for Chapter 5. An AI parrot repeats information about AI doom panicking humans.
Bender et al. (2021) was a landmark paper where researchers first raised significant warnings about large language models, characterizing them as stochastic parrots. Some of these researchers paid for their bravery with their jobs, and particularly in the UK, their voices and those of other female researchers were largely erased from public debate.
Today we see a second type of stochastic parrot emerging: “fleshy GPTs” who speak confidently and eloquently but lack real-world experience. Like the language models they champion, they make arguments that appear superficially convincing but reveal naive flaws to those with deeper domain knowledge. Ironically, some of these voices even claim the research community failed to warn about the implications of these technologies, despite papers like Bender et al. (2021) doing exactly that.
See this reflection on Two Types of Stochastic Parrots.
Atrophy and Cognitive Flattening
Even if we calibrate these tools correctly so that they do represent the world appropriately we are at risk. The hippocampus is part of our brain’s cortex, oOne role of our hippocampus is in navigation, knowing how to move from one place to another.
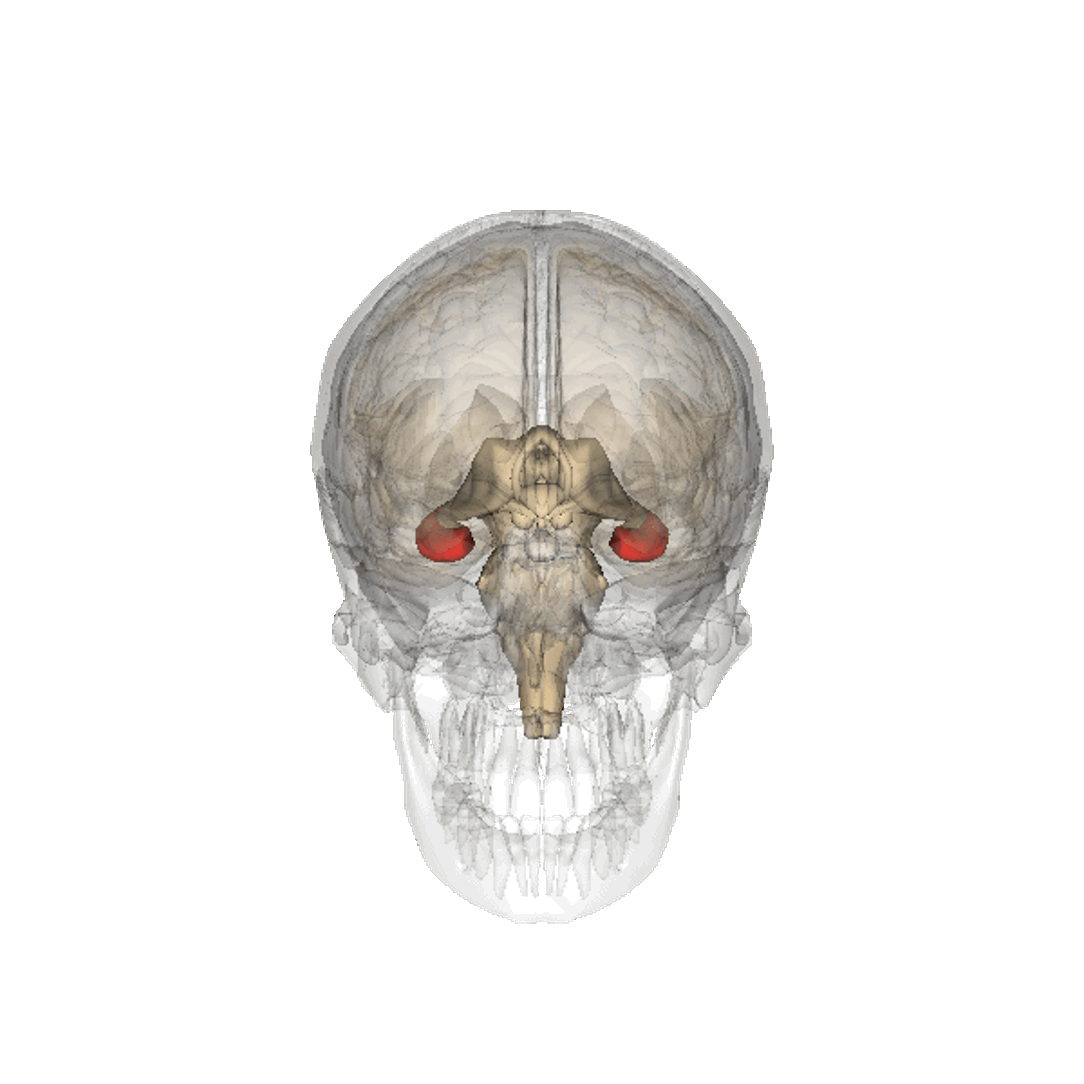
Figure: The hippocampus within the brain. Image generated from Anatomography, https://lifesciencedb.jp/.
Our prefrontal cortex, the size of which differentiates us from other primates and mammals, is the seat of our planning and of our higher intelligence. It fires in sympathy with our hippocampus.
By becoming overreliant on HAMs and there interface to the digital computer are we at risk of suffering from the same deskilling we perceive in our navigational skills? Does this place us at risk of sleepwalking into a world that is managed by the machine even as we believe that we are managing it ourselves?
Case Study: Science
Conjectures and Refutations
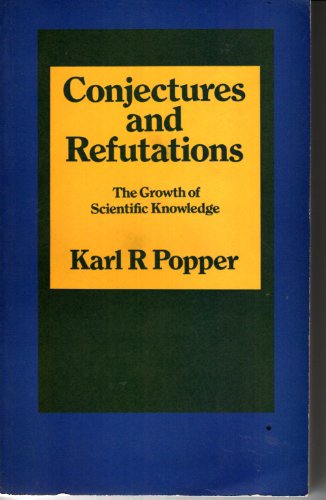
Figure: Conjectures and Refutations by Karl Popper presents his philosophy of science based on falsification.
In “Conjectures and Refutations” (Popper, 1963), Popper elaborates on his philosophy of science, arguing that scientific knowledge progresses through bold conjectures that are then subjected to rigorous attempts at refutation. He contrasts this with verification-based approaches, arguing that no amount of confirmatory evidence can prove a universal theory, but a single counter-example can disprove it.
This philosophy of falsificationism suggests that scientists should actively try to disprove their theories rather than seek confirming evidence. For Popper, what makes a theory scientific is not its ability to be verified, but its ability to be falsified - it must make predictions that could potentially be proven wrong.
These ideas have profound implications for how we understand scientific progress and the scientific method. It implies science isn’t an activity of individuals, it is necessarily a community effort.
See Lawrence (2024) Popper, Karl: Conjectures and Refutations p. 327,328.
The Structure of Scientific Revolutions
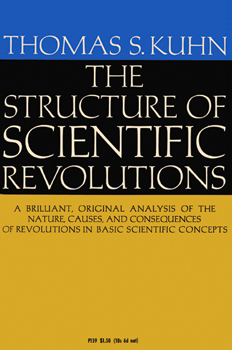
Figure: The Structure of Scientific Revolutions by Thomas S. Kuhn suggests scientific paradigms are recorded in books.
Kuhn was a historian of science and a philosopher who suggested that the sociology of science has two principal components to it. His idea is that “normal science” operates within a paradigm That paradigm is defined by books which encode our best understanding. An example of a paradigm is Newtonian mechanics, or another example would be the geocentric view of the Universe. Within a paradigm normal science proceeds by scientists solving the “puzzles” that paradigm sets. A paradigm shift is when the paradigm changes, for example the Corpernican revolution or the introduction of relativity.
The notion of a paradigm shift has also entered common parlance, this reflects the idea that wider human knowledge is also shared and stored, less ormally than scientific knowledge, but still with a dependence on our information infrastructure.
The digital computer has brought a fundamental change in the nature of that information infrastructure. By moving information faster the modern information infrastructure is dominated not by the book, but by the machine. This brings challenges for managing and controlling this information infrastructure.
See Lawrence (2024) Kuhn, Thomas: The Structure of Scientific Revolutions p. 295–299.
AI Nobel Prizes
In 2024, artificial intelligence researchers were recognized at the highest level of scientific achievement with Nobel Prizes in both Physics and Chemistry.
The Physics prize was awarded jointly to John J. Hopfield and Geoffrey Hinton “for foundational discoveries and inventions that enable machine learning with artificial neural networks”. Hopfield’s contributions included developing recurrent neural networks for associative memory, while Hinton pioneered deep learning techniques that revolutionized the field.
The Chemistry prize was shared between David Baker, Demis Hassabis, and John Jumper “for computational protein design and protein structure prediction”. Their work, particularly through DeepMind’s AlphaFold system, solved the long-standing challenge of predicting protein structures from amino acid sequences.
These awards mark a pivotal moment in the history of artificial intelligence, recognizing both the theoretical foundations laid in the 1980s and the recent breakthroughs that have transformed the technology into a powerful scientific tool. The prizes highlight how AI has evolved from an academic pursuit into a transformative force across multiple scientific disciplines.
The recognition of British-educated researchers Hinton and Hassabis also underscores the UK’s significant contributions to AI development, reflecting a legacy of pioneering work in computer science and artificial intelligence stretching back to Alan Turing.
The Gatsby Unit
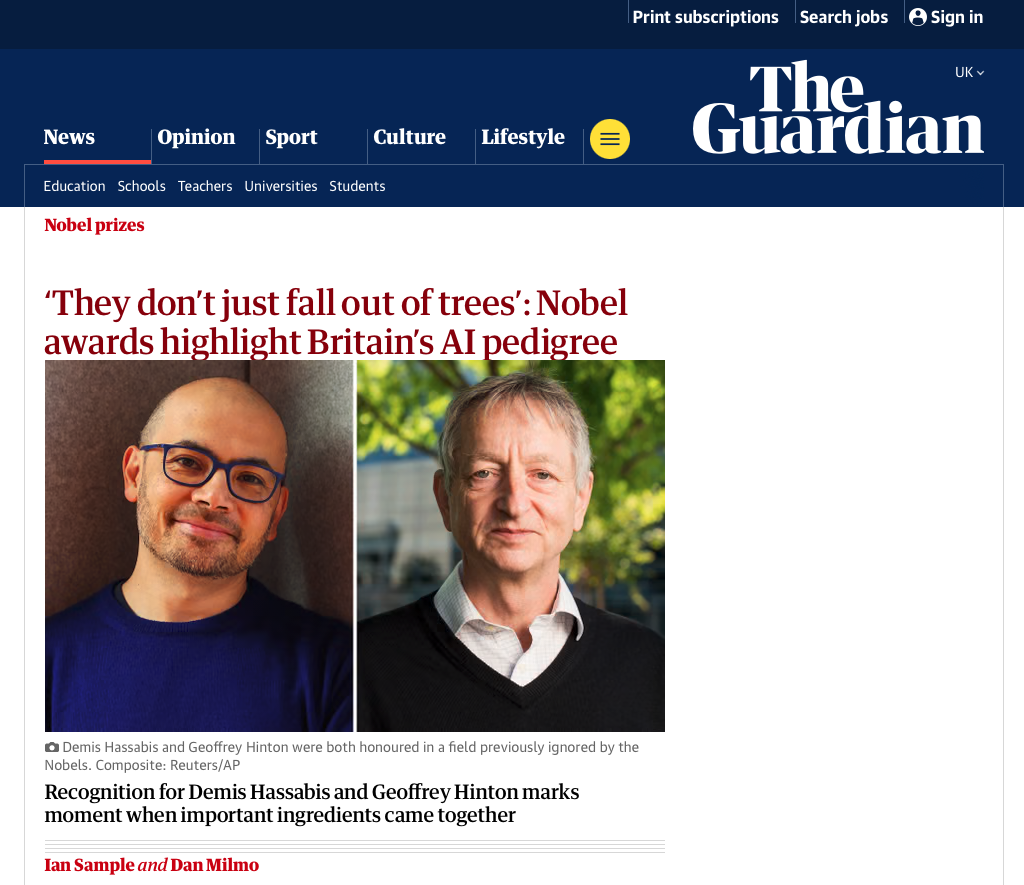
Figure: The Gatsby Computational Neuroscience Unit at University College London was founded by Geoffrey Hinton, who would later win the 2024 Nobel Prize in Physics, the Unit became “a phenomenal draw” for talent as described in this Guardian article.
The Gatsby Computational Neuroscience Unit at University College London was founded by Geoffrey Hinton, who would later win the 2024 Nobel Prize in Physics, the Unit became “a phenomenal draw” for talent. Its impact is evident in its role nurturing future leaders in AI. Demis Hassabis conducted his postdoctoral research there.
In this Guardian article Maneesh Sahani (current directory) calls this “a chain reaction” - when critical mass is achieved, “people who are doing exciting things and talking to each other” attract others who want to participate.
As Dame Wendy Hall notes in the article, “It takes 20 years or more to grow a research star like Hassabis. They don’t just fall out of the trees.”
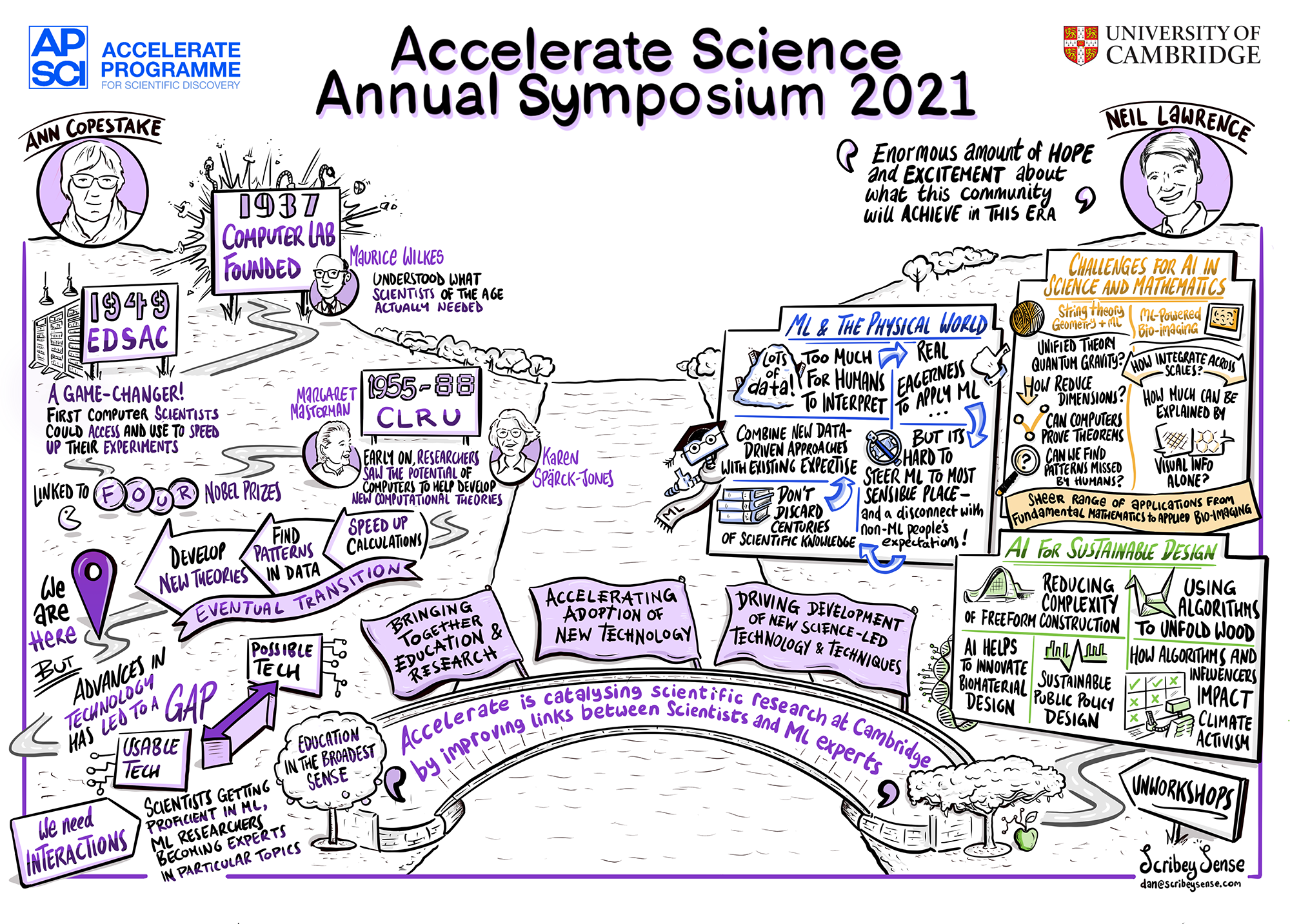
Figure: We need to bridge between domain expertise and machine learning/AI capability.
Science not Scientists
The football pundit and former Wales International Robbie Savage is passionate about grassroots football. He has managed youth teams. Robbie’s son also plays at a junior level, following his Dad’s footsteps at Manchester United’s academy. On occasion I’ve heard Robbie on podcasts talking about his son’s fledgling career. In Robbie’s voice you can hear the hopes and concerns that every parent has for their own child as they begin to make their way in the world.
My son does not play for Manchester United (anyway, he’d prefer to play for Sheffield United) but watching him play Sunday league, I’m aware that I’m not objective about his play, and I’m aware that the parents around me are also not objective. Over my life, my son has played in four different teams with five different managers. And most of these managers also have also had a child playing in the team.
This makes watching him play different from watching another team. The parents’ presence means that grassroots football is subjective in a particular way. Every parent has an interest in how their child is performing. Different parents have different levels of awareness of this subjectivity, some play down their children’s contribuion, others play it up.
Scientists are similar to the parents watching their children play grassroots football. Most scientists have a particular field, a particular domain and a particular pet idea. As a result, we should be skeptical about their individual claims, just as we might be more skeptical about a parent’s report of their own child’s performance. Parents and scientists are people, some of them are more self aware and are better at dealing with this subjectivity, but the truth remains that science isn’t scientists.
Ogni scarrafone è bello a mamma soja
Neopolitan expression
Scientists are a group of people with particular subjective biases, and their individual opinion may count for a lot within their domain of expertise, but when multidisciplinary questions of policy are in play, then their individual opinions should be treated with some skepticism.
The process of science involves scientists but it also involves mechanisms that include peer review and debate. Importantly it also includes referees in the form of statisticians, who are trained to treat data subjectively.
Science itself is also not policy. Policy decisions often have some urgency which means the normal processes of scientific proof do not have time to take their course. These decisions are often about balancing long term versus short term uncertainties.
See for example this blog post on Primer on Decision Making with Uncertainty..
In the moment of policy crisis such as what we’ve seen in Covid-19 it also has people who are used to keeping the score. Public health professionals are experienced in disease interventions. Many of them having seen how scientific ideas pan out in practice, which turn out to be successful and which sound promising but repeatedly fail to deliver.
Kahneman refers to our awe of theory as theory induces blindness (Kahneman, 2011), but I feel a more appropriate phrase is model-induced blindness or “model-blinkers”.
See Lawrence (2024) theory induced blindness p. 301. See Lawrence (2024) model-blinkers p. 301,302,308.
Blake’s Newton
William Blake’s rendering of Newton captures humans in a particular state. He is trance-like absorbed in simple geometric shapes. The feel of dreams is enhanced by the underwater location, and the nature of the focus is enhanced because he ignores the complexity of the sea life around him.
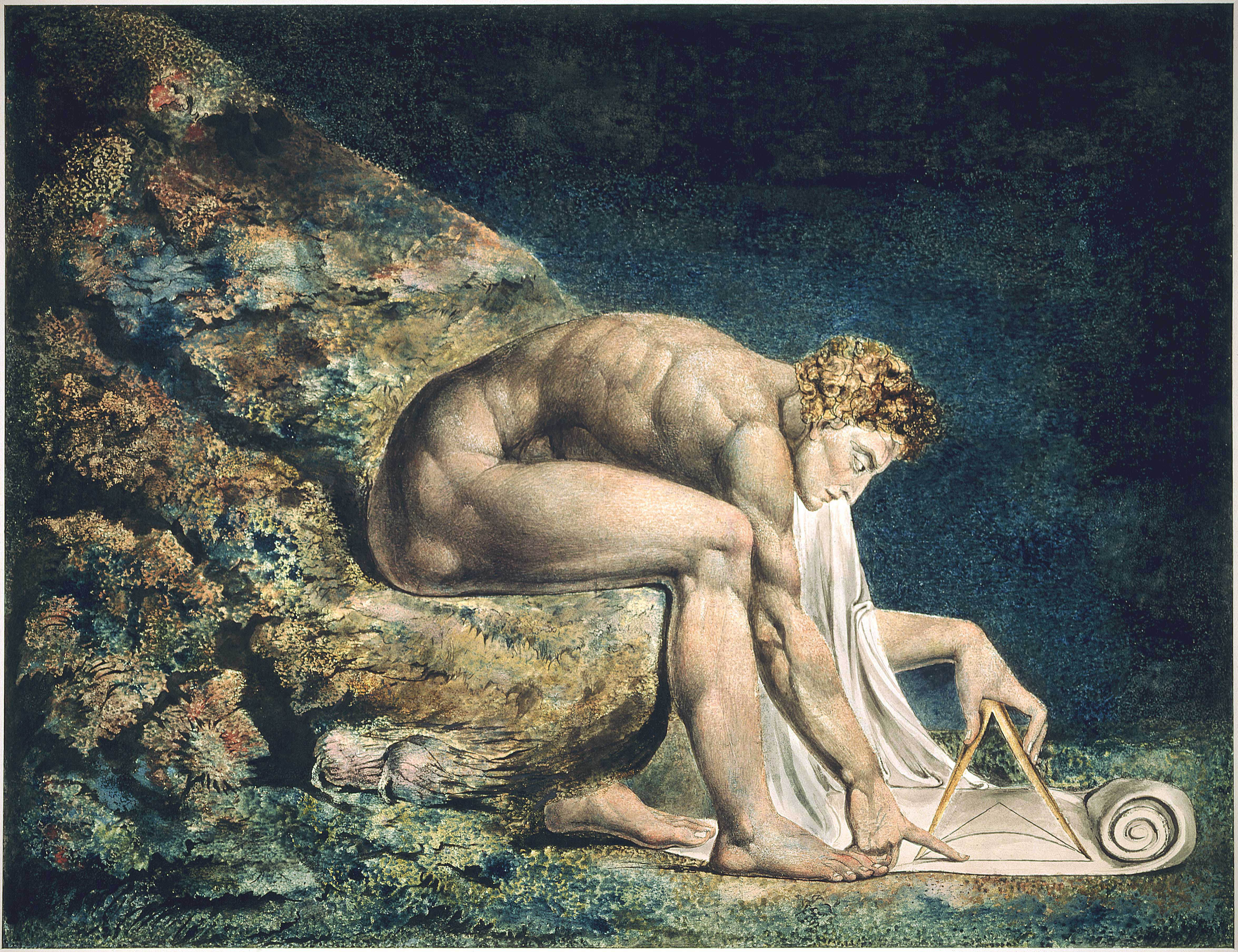
Figure: William Blake’s Newton. 1795c-1805
See Lawrence (2024) Blake, William Newton p. 121–123.
The caption in the Tate Britain reads:
Here, Blake satirises the 17th-century mathematician Isaac Newton. Portrayed as a muscular youth, Newton seems to be underwater, sitting on a rock covered with colourful coral and lichen. He crouches over a diagram, measuring it with a compass. Blake believed that Newton’s scientific approach to the world was too reductive. Here he implies Newton is so fixated on his calculations that he is blind to the world around him. This is one of only 12 large colour prints Blake made. He seems to have used an experimental hybrid of printing, drawing, and painting.
From the Tate Britain
See Lawrence (2024) Blake, William Newton p. 121–123, 258, 260, 283, 284, 301, 306.
Societal Hidden Labour
Model of Transformation
The MONIAC
The MONIAC was an analogue computer designed to simulate the UK economy. Analogue comptuers work through analogy, the analogy in the MONIAC is that both money and water flow. The MONIAC exploits this through a system of tanks, pipes, valves and floats that represent the flow of money through the UK economy. Water flowed from the treasury tank at the top of the model to other tanks representing government spending, such as health and education. The machine was initially designed for teaching support but was also found to be a useful economic simulator. Several were built and today you can see the original at Leeds Business School, there is also one in the London Science Museum and one in the Unisversity of Cambridge’s economics faculty.
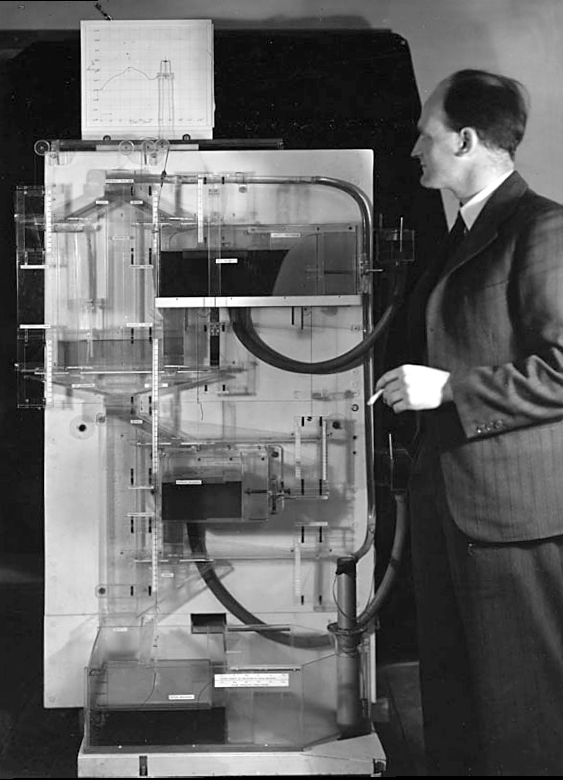
Figure: Bill Phillips and his MONIAC (completed in 1949). The machine is an analogue computer designed to simulate the workings of the UK economy.
See Lawrence (2024) MONIAC p. 232-233, 266, 343.
Human Analogue Machine
Recent breakthroughs in generative models, particularly large language models, have enabled machines that, for the first time, can converse plausibly with other humans.
The Apollo guidance computer provided Armstrong with an analogy when he landed it on the Moon. He controlled it through a stick which provided him with an analogy. The analogy is based in the experience that Amelia Earhart had when she flew her plane. Armstrong’s control exploited his experience as a test pilot flying planes that had control columns which were directly connected to their control surfaces.
Figure: The human analogue machine is the new interface that large language models have enabled the human to present. It has the capabilities of the computer in terms of communication, but it appears to present a “human face” to the user in terms of its ability to communicate on our terms. (Image quite obviously not drawn by generative AI!)
The generative systems we have produced do not provide us with the “AI” of science fiction. Because their intelligence is based on emulating human knowledge. Through being forced to reproduce our literature and our art they have developed aspects which are analogous to the cultural proxy truths we use to describe our world.
These machines are to humans what the MONIAC was the British economy. Not a replacement, but an analogue computer that captures some aspects of humanity while providing advantages of high bandwidth of the machine.
HAM
The Human-Analogue Machine or HAM therefore provides a route through which we could better understand our world through improving the way we interact with machines.
Figure: The trinity of human, data, and computer, and highlights the modern phenomenon. The communication channel between computer and data now has an extremely high bandwidth. The channel between human and computer and the channel between data and human is narrow. New direction of information flow, information is reaching us mediated by the computer. The focus on classical statistics reflected the importance of the direct communication between human and data. The modern challenges of data science emerge when that relationship is being mediated by the machine.
The HAM can provide an interface between the digital computer and the human allowing humans to work closely with computers regardless of their understandin gf the more technical parts of software engineering.
Figure: The HAM now sits between us and the traditional digital computer.
Of course this route provides new routes for manipulation, new ways in which the machine can undermine our autonomy or exploit our cognitive foibles. The major challenge we face is steering between these worlds where we gain the advantage of the computer’s bandwidth without undermining our culture and individual autonomy.
See Lawrence (2024) human-analogue machine (HAMs) p. 343-347, 359-359, 365-368.
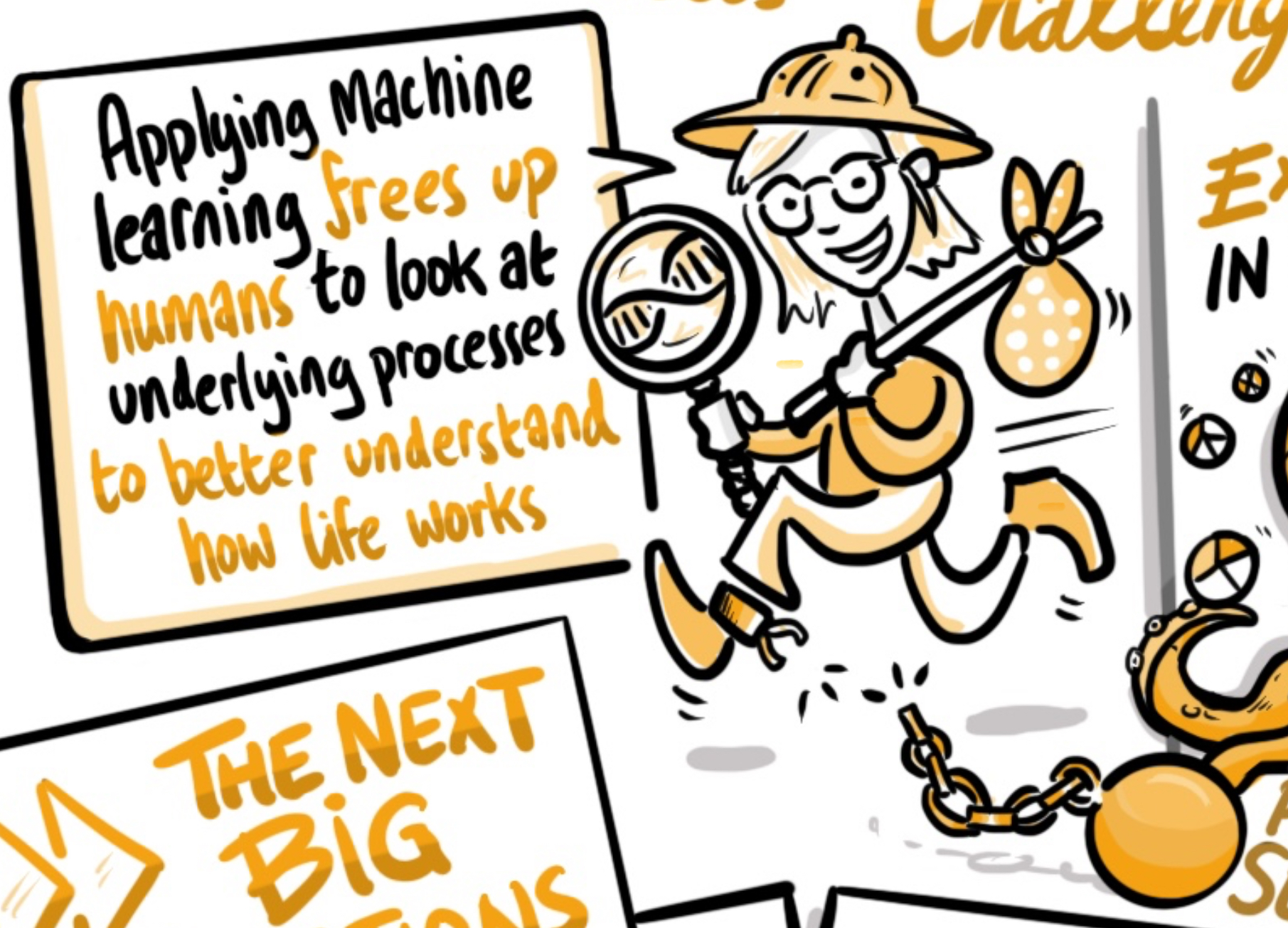
Figure: Machine Learning frees up humans. Still from graphical rendering of Unworkshop on ML for Challenges in Maths and Science from Accelerate Science Summit 2021.
The Attention Reinvestment Cycle
Supply Chain of Ideas
Model is “supply chain of ideas” framework, particularly in the context of information technology and AI solutions like machine learning and large language models. You suggest that this idea flow, from creation to application, is similar to how physical goods move through economic supply chains.
In the realm of IT solutions, there’s been an overemphasis on macro-economic “supply-side” stimulation - focusing on creating new technologies and ideas - without enough attention to the micro-economic “demand-side” - understanding and addressing real-world needs and challenges.
Imagining the supply chain rather than just the notion of the Innovation Economy allows the conceptualisation of the gaps between macro and micro economic issues, enabling a different way of thinking about process innovation.
Phrasing things in terms of a supply chain of ideas suggests that innovation requires both characterisation of the demand and the supply of ideas. This leads to four key elements:
- Multiple sources of ideas (diversity)
- Efficient delivery mechanisms
- Quick deployment capabilities
- Customer-driven prioritization
The next priority is mapping the demand for ideas to the supply of ideas. This is where much of our innovation system is failing. In supply chain optimisaiton a large effort is spent on understanding current stock and managing resources to bring the supply to map to the demand. This includes shaping the supply as well as managing it.
The objective is to create a system that can generate, evaluate, and deploy ideas efficiently and effectively, while ensuring that people’s needs and preferences are met. The customer here depends on the context - it could be the public, it could be a business, it could be a government department but very often it’s individual citizens. The loss of their voice in the innovation economy is a trigger for the gap between the innovation supply (at a macro level) and the innovation demand (at a micro level).
AI cannot replace atomic human
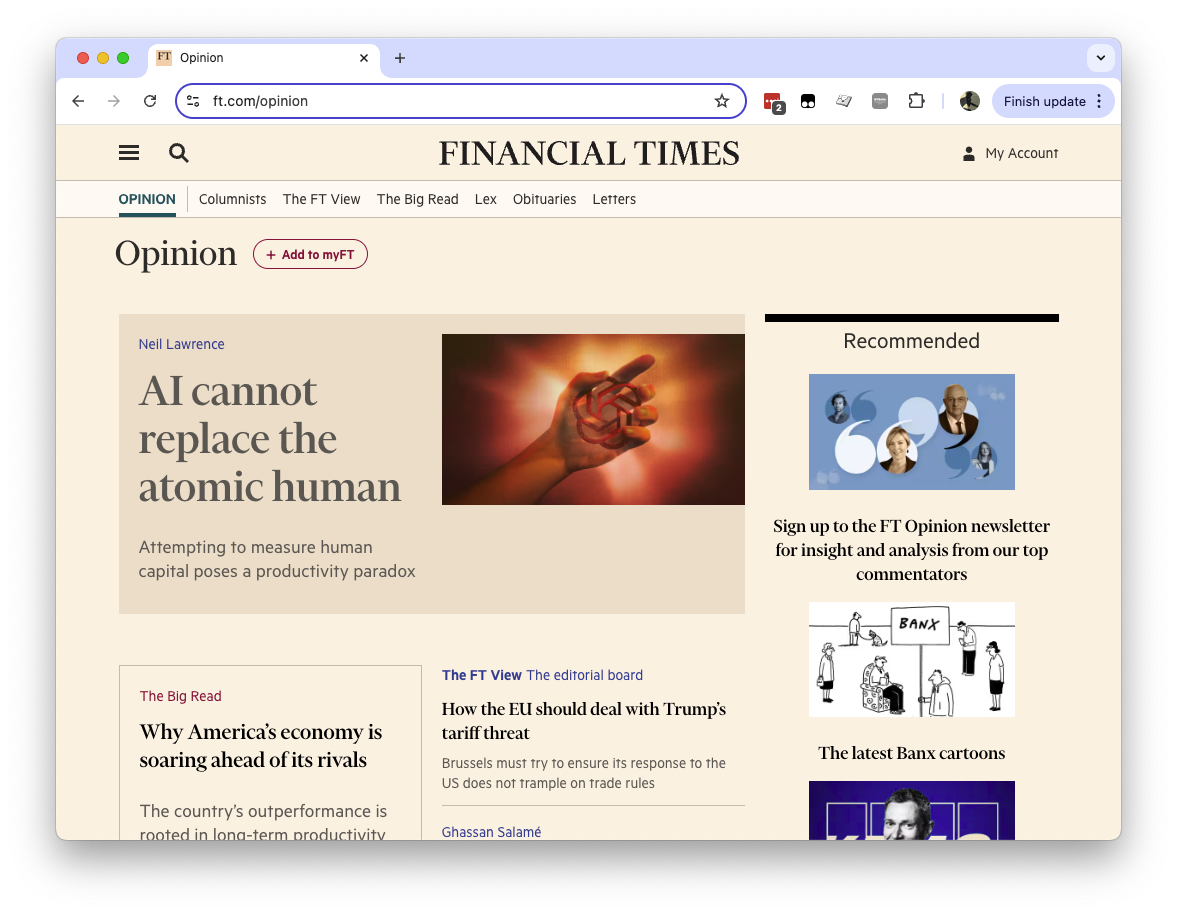
Figure: Opinion piece in the FT that describes the idea of a social flywheel to drive the targeted growth we need in AI innovation.
New Attention Flywheel
Figure: The attention flywheel focusses on reinvesting human capital.
Example: Data Science Africa
Data Science Africa is a grass roots initiative that focuses on capacity building to develop ways of solving on the ground problems in health, education, transport and conservation in way that is grounded in local needs and capabilities.
Data Science Africa
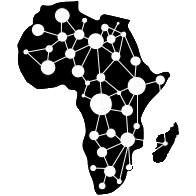
Figure: Data Science Africa https://datascienceafrica.org is a ground up initiative for capacity building around data science, machine learning and artificial intelligence on the African continent.
Figure: Data Science Africa meetings held up to October 2021.
Data Science Africa is a bottom up initiative for capacity building in data science, machine learning and artificial intelligence on the African continent.
As of May 2023 there have been eleven workshops and schools, located in seven different countries: Nyeri, Kenya (twice); Kampala, Uganda; Arusha, Tanzania; Abuja, Nigeria; Addis Ababa, Ethiopia; Accra, Ghana; Kampala, Uganda and Kimberley, South Africa (virtual), and in Kigali, Rwanda.
The main notion is end-to-end data science. For example, going from data collection in the farmer’s field to decision making in the Ministry of Agriculture. Or going from malaria disease counts in health centers to medicine distribution.
The philosophy is laid out in (Lawrence, 2015). The key idea is that the modern information infrastructure presents new solutions to old problems. Modes of development change because less capital investment is required to take advantage of this infrastructure. The philosophy is that local capacity building is the right way to leverage these challenges in addressing data science problems in the African context.
Data Science Africa is now a non-govermental organization registered in Kenya. The organising board of the meeting is entirely made up of scientists and academics based on the African continent.
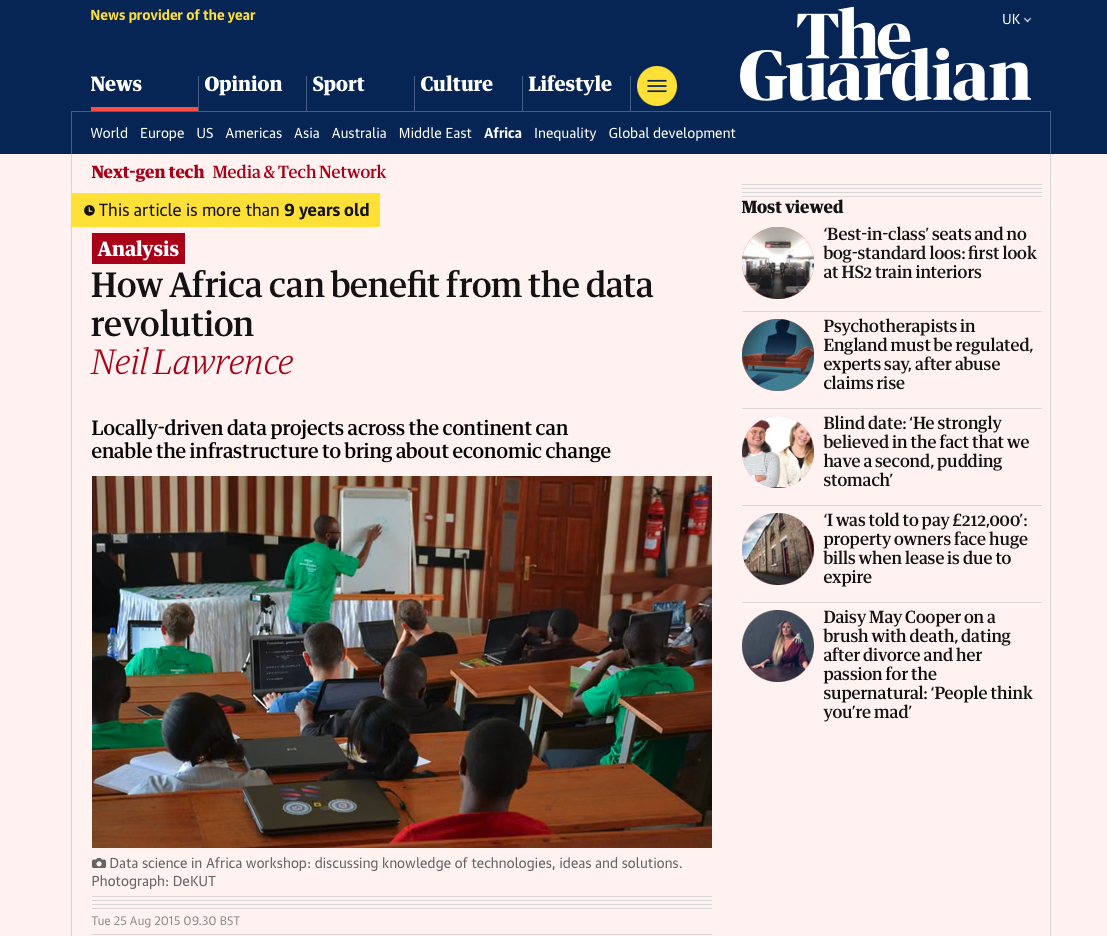
Figure: The lack of existing physical infrastructure on the African continent makes it a particularly interesting environment for deploying solutions based on the information infrastructure. The idea is explored more in this Guardian op-ed on Guardian article on How African can benefit from the data revolution.
Guardian article on Data Science Africa
Example: Cambridge Approach
ai@cam is the flagship University mission that seeks to address these challenges. It recognises that development of safe and effective AI-enabled innovations requires this mix of expertise from across research domains, businesses, policy-makers, civil society, and from affected communities. AI@Cam is setting out a vision for AI-enabled innovation that benefits science, citizens and society.
ai@cam
The ai@cam vision is being achieved in a manner that is modelled on other grass roots initiatives like Data Science Africa. Through leveraging the University’s vibrant interdisciplinary research community. ai@cam has formed partnerships between researchers, practitioners, and affected communities that embed equity and inclusion. It is developing new platforms for innovation and knowledge transfer. It is delivering innovative interdisciplinary teaching and learning for students, researchers, and professionals. It is building strong connections between the University and national AI priorities.
We are working across the University to empower the diversity of expertise and capability we have to focus on these broad societal problems. In April 2022 we shared an ai@cam with a vision document that outlines these challenges for the University.
The University operates as both an engine of AI-enabled innovation and steward of those innovations.
AI is not a universal remedy. It is a set of tools, techniques and practices that correctly deployed can be leveraged to deliver societal benefit and mitigate social harm.
The initiative was funded in November 2022 where a £5M investment from the University.
The progress made so far has been across the University community. We have successfully engaged with over members spanning more than 30 departments and institutes, bringing together academics, researchers, start-ups, and large businesses to collaborate on AI initiatives. The program has already supported 6 new funding bids and launched five interdisciplinary A-Ideas projects that bring together diverse expertise to tackle complex challenges. The establishment of the Policy Lab has created a crucial bridge between research and policy-making. Additionally, through the Pioneer program, we have initiated 46 computing projects that are helping to build our technical infrastructure and capabilities.
How ai@cam is Addressing Innovation Challenges
1. Bridging Macro and Micro Levels
Challenge: There is often a disconnect between high-level AI research and real-world needs that must be addressed.
The A-Ideas Initiative represents an effort to bridge this gap by funding interdisciplinary projects that span 19 departments across 6 schools. This ensures diverse perspectives are brought to bear on pressing challenges. Projects focusing on climate change, mental health, and language equity demonstrate how macro-level AI capabilities can be effectively applied to micro-level societal needs.
Challenge: Academic insights often fail to translate into actionable policy changes.
The Policy Lab initiative addresses this by creating direct connections between researchers, policymakers, and the public, ensuring academic insights can influence policy decisions. The Lab produces accessible policy briefs and facilitates public dialogues. A key example is the collaboration with the Bennett Institute and Minderoo Centre, which resulted in comprehensive policy recommendations for AI governance.
2. Addressing Data, Compute, and Capability Gaps
Challenge: Organizations struggle to balance data accessibility with security and privacy concerns.
The data intermediaries initiative establishes trusted entities that represent the interests of data originators, helping to establish secure and ethical frameworks for data sharing and use. Alongside approaches for protecting data we need to improve our approach to processing data. Careful assessment of data quality and organizational data maturity ensures that data can be shared and used effectively. Together these approaches help to ensure that data can be used to serve science, citizens and society.
2. Addressing data, Compute and Capability Gaps
Challenge: Many researchers lack access to necessary computational resources for modern research.
The HPC Pioneer Project addresses this by providing access to the Dawn supercomputer, enabling 46 diverse projects across 20 departments to conduct advanced computational research. This democratization of computing resources ensures that researchers from various disciplines can leverage high-performance computing for their work. The ai@cam project also supports the ICAIN initiative, further strengthening the computational infrastructure available to researchers with a particular focus on emerging economies.
Challenge: There is a significant skills gap in applying AI across different academic disciplines.
The Accelerate Programme for Scientific Discovery addresses this through a comprehensive approach to building AI capabilities. Through a tiered training system that ranges from basic to advanced levels, the programme ensures that domain experts can develop the AI skills relevant to their field. The initiative particularly emphasizes peer-to-peer learning creating sustainable communities of practice where researchers can share knowledge and experiences through “AI Clubs”.
The Accelerate Programme
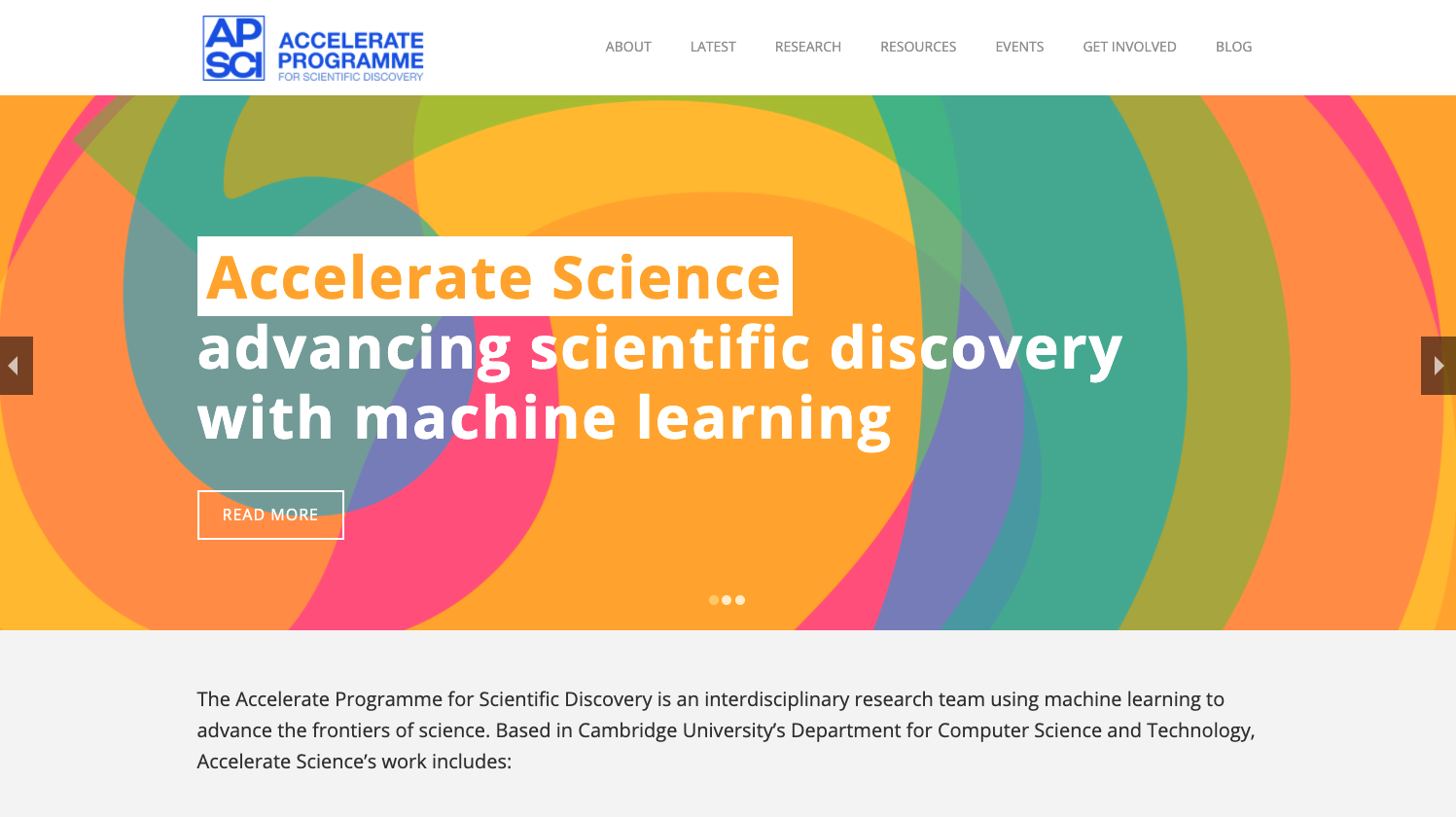
Figure: The Accelerate Programme for Scientific Discovery covers research, education and training, engagement. Our aim is to bring about a step change in scientific discovery through AI. http://science.ai.cam.ac.uk
We’re now in a new phase of the development of computing, with rapid advances in machine learning. But we see some of the same issues – researchers across disciplines hope to make use of machine learning, but need access to skills and tools to do so, while the field machine learning itself will need to develop new methods to tackle some complex, ‘real world’ problems.
It is with these challenges in mind that the Computer Lab has started the Accelerate Programme for Scientific Discovery. This new Programme is seeking to support researchers across the University to develop the skills they need to be able to use machine learning and AI in their research.
To do this, the Programme is developing three areas of activity:
- Research: we’re developing a research agenda that develops and applies cutting edge machine learning methods to scientific challenges, with three Accelerate Research fellows working directly on issues relating to computational biology, psychiatry, and string theory. While we’re concentrating on STEM subjects for now, in the longer term our ambition is to build links with the social sciences and humanities.
Progress so far includes:
Recruited a core research team working on the application of AI in mental health, bioinformatics, healthcare, string theory, and complex systems.
Created a research agenda and roadmap for the development of AI in science.
Funded interdisciplinary projects, e.g. in first round:
Antimicrobial resistance in farming
Quantifying Design Trade-offs in Electricity-generation-focused Tokamaks using AI
Automated preclinical drug discovery in vivo using pose estimation
Causal Methods for Environmental Science Workshop
Automatic tree mapping in Cambridge
Acoustic monitoring for biodiversity conservation
AI, mathematics and string theory
Theoretical, Scientific, and Philosophical Perspectives on Biological Understanding in the age of Artificial Intelligence
AI in pathology: optimising a classifier for digital images of duodenal biopsies
Teaching and learning: building on the teaching activities already delivered through University courses, we’re creating a pipeline of learning opportunities to help PhD students and postdocs better understand how to use data science and machine learning in their work.
Progress so far includes:
Teaching and learning
Brought over 250 participants from over 30 departments through tailored data science and machine learning for science training (Data Science Residency and Machine Learning Academy);
Convened workshops with over 80 researchers across the University on the development of data pipelines for science;
Delivered University courses to over 100 students in Advanced Data Science and Machine Learning and the Physical World.
Online training course in Python and Pandas accessed by over 380 researchers.
Engagement: we hope that Accelerate will help build a community of researchers working across the University at the interface on machine learning and the sciences, helping to share best practice and new methods, and support each other in advancing their research. Over the coming years, we’ll be running a variety of events and activities in support of this.
Progress so far includes:
- Launched a Machine Learning Engineering Clinic that has supported over 40 projects across the University with MLE troubleshooting and advice;
- Hosted and participated in events reaching over 300 people in Cambridge;
- Led international workshops at Dagstuhl and Oberwolfach, convening over 60 leading researchers;
- Engaged over 70 researchers through outreach sessions and workshops with the School of Clinical Medicine, the Faculty of Education, Cambridge Digital Humanities and the School of Biological Sciences.
3. Stakeholder Engagement and Feedback Mechanisms
Challenge: AI development often proceeds without adequate incorporation of public perspectives and concerns.
Our public dialogue work, conducted in collaboration with the Kavli Centre for Ethics, Science, and the Public, creates structured spaces for public dialogue about AI’s potential benefits and risks. The approach ensures that diverse voices and perspectives are heard and considered in AI development.
Challenge: AI initiatives often fail to align with diverse academic needs across institutions.
Cross-University Workshops serve as vital platforms for alignment, bringing together faculty and staff from different departments to discuss AI teaching and learning strategies. By engaging professional services staff, the initiative ensures that capability building extends beyond academic departments to support staff who play key roles in implementing and maintaining AI systems.
4. Flexible and Adaptable Approaches
Challenge: Traditional rigid, top-down research agendas often fail to address real needs effectively.
The AI-deas Challenge Development program empowers researchers to identify and propose challenge areas based on their expertise and understanding of field needs. Through collaborative workshops, these initial ideas are refined and developed, ensuring that research directions emerge organically from the academic community while maintaining alignment with broader strategic goals.
5. Phased Implementation and Realistic Planning
Challenge: Ambitious AI initiatives often fail due to unrealistic implementation timelines and expectations.
The overall strategy emphasizes careful, phased deployment to ensure sustainable success. Beginning with pilot programs like AI-deas and the Policy Lab, the approach allows for testing and refinement of methods before broader implementation. This measured approach enables the incorporation of lessons learned from early phases into subsequent expansions.
6. Independent Oversight and Diverse Perspectives
Challenge: AI initiatives often lack balanced guidance and oversight from diverse perspectives.
The Steering Group provides crucial oversight through representatives from various academic disciplines and professional services. Working with a cross-institutional team, it ensures balanced decision-making that considers multiple perspectives. The group maintains close connections with external initiatives like ELLIS, ICAIN, and Data Science Africa, enabling the university to benefit from and contribute to broader AI developments.
7. Addressing the Innovation Supply Chain
Challenge: Academic innovations often struggle to connect with and address industry needs effectively.
The Industry Engagement initiative develops meaningful industrial partnerships through collaboration with the Strategic Partnerships Office, helping translate research into real-world solutions. The planned sciencepreneurship initiative aims to create a structured pathway from academic research to entrepreneurial ventures, helping ensure that innovations can effectively reach and benefit society.
Bandwidth vs Complexity
The computer communicates in Gigabits per second, One way of imagining just how much slower we are than the machine is to look for something that communicates in nanobits per second.
![]() |
|||
bits/min | \(100 \times 10^{-9}\) | \(2,000\) | \(600 \times 10^9\) |
Figure: When we look at communication rates based on the information passing from one human to another across generations through their genetics, we see that computers watching us communicate is roughly equivalent to us watching organisms evolve. Estimates of germline mutation rates taken from Scally (2016).
Figure: Bandwidth vs Complexity.
The challenge we face is that while speed is on the side of the machine, complexity is on the side of our ecology. Many of the risks we face are associated with the way our speed undermines our ecology and the machines speed undermines our human culture.
See Lawrence (2024) Human evolution rates p. 98-99. See Lawrence (2024) Psychological representation of Ecologies p. 323-327.
The Atomic Human
Thanks!
For more information on these subjects and more you might want to check the following resources.
- book: The Atomic Human
- twitter: @lawrennd
- podcast: The Talking Machines
- newspaper: Guardian Profile Page
- blog: http://inverseprobability.com