Bridging BeSci & Data Science in the UN
Abstract
How can behavioural science and data science come together to drive meaningful change in the UN? This advanced session will explore innovative and existing approaches to using BeSci and data for efficiency gains, informed decision-making, and strategic insights. Professor Neil Lawrence (University of Cambridge) will provide opening remarks, setting the stage for a discussion on human-centred data science in UN contexts. Colleagues from UNHCR and UNICEF will then share real-world examples, discuss the challenges of integrating data and BeSci, and highlight the value of partnerships and research. From overcoming data constraints to investing in the right skill sets, this session will examine how to maximise BeSci and analytics for scalable impact particularly in resource-sensitive environments.
The Attention Economy and Human Capital
The Attention Economy
Herbert Simon on Information
What information consumes is rather obvious: it consumes the attention of its recipients. Hence a wealth of information creates a poverty of attention …
Simon (1971)
The attention economy was a phenomenon described in 1971 by the American computer scientist Herbert Simon. He saw the coming information revolution and wrote that a wealth of information would create a poverty of attention. Too much information means that human attention becomes the scarce resource, the bottleneck. It becomes the gold in the attention economy.
The power associated with control of information dates back to the invention of writing. By pressing reeds into clay tablets Sumerian scribes stored information and controlled the flow of information.
In our modern economy, automation has the same effect. During the industrial revolution, steel and steam replaced human manual labour. Today, silicon and electrons are being combined to replace human mental labour.
Human Capital Index
The World Bank’s human capital index is one area where many European countries are leading international economy, or at least an area where they currently outperform both the USA and China. The index is a measure of education and health of a population.
Technological progress disrupts existing systems. A new social contract is needed to smooth the transition and guard against rising inequality. Significant investments in human capital throughout a person’s lifecycle are vital to this effort.
World Bank (2019)]
The Productivity and Attention Flywheels
Productivity Flywheel
Figure: The productivity flywheel suggests technical innovation is reinvested.
The productivity flywheel should return the gains released by productivity through funding. This relies on the economic value mapping the underlying value.
Attention Reinvestment Cycle
Figure: The attention flywheel focusses on reinvesting human capital.
While the traditional productivity flywheel focuses on reinvesting financial capital, the attention flywheel focuses on reinvesting human capital - our most precious resource in an AI-augmented world. This requires deliberately creating systems that capture the value of freed attention and channel it toward human-centered activities that machines cannot replicate.
Public Dialogue and Human-Centered Design
Public Dialogue on AI in Public Services
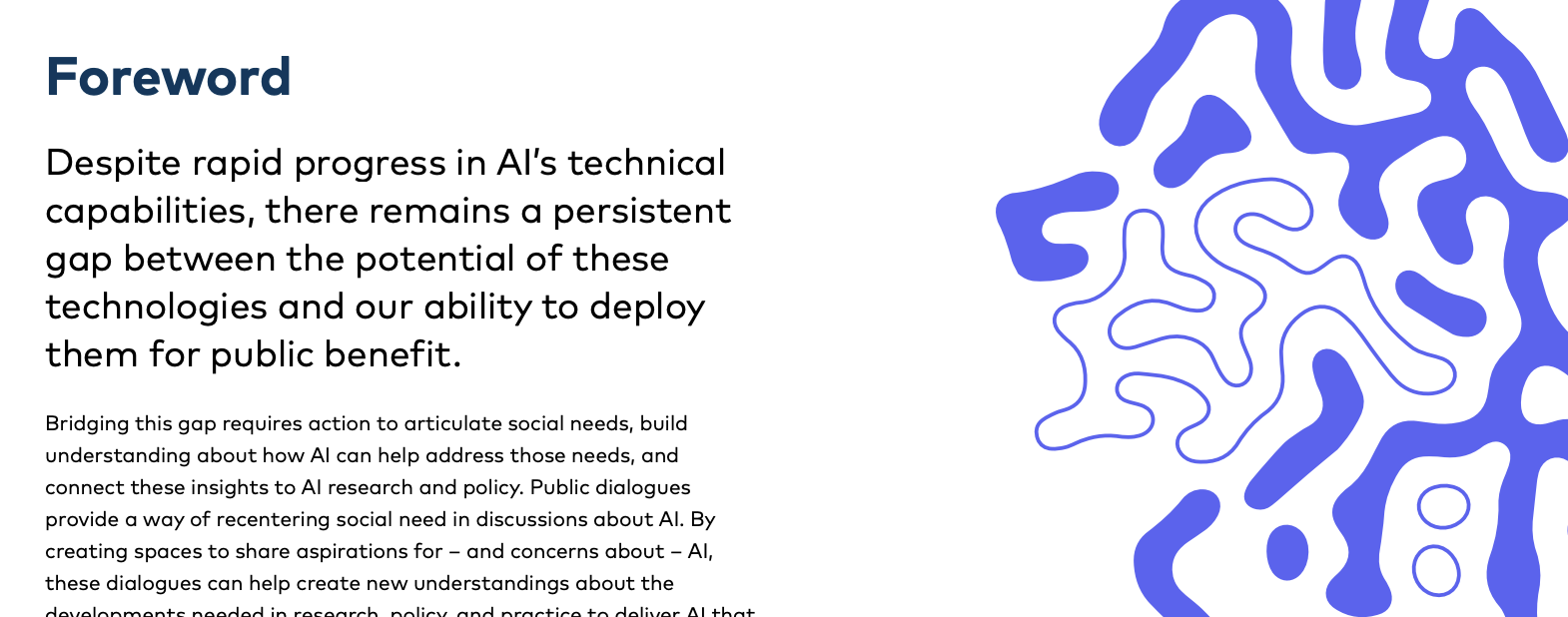
Figure: In September 2024, ai@cam convened a series of public dialogues to understand perspectives on the role of AI in delivering policy agendas.
In September 2024, ai@cam convened a series of public dialogues to understand perspectives on the role of AI in delivering priority policy agendas. Through workshops in Cambridge and Liverpool, 40 members of the public shared their views on how AI could support delivery of four key government missions around health, crime and policing, education, and energy and net zero.
The dialogue revealed a pragmatic public view that sees clear benefits from AI in reducing administrative burdens and improving service efficiency, while emphasizing the importance of maintaining human-centered services and establishing robust governance frameworks.
Key participant quotes illustrate this balanced perspective:
“It must be so difficult for GPs to keep track of how medication reacts with other medication on an individual basis. If there’s some database that shows all that, then it can only help, can’t it?”
Public Participant, Liverpool pg 10 ai@cam and Hopkins Van Mil (2024)
“I think a lot of the ideas need to be about AI being like a co-pilot to someone. I think it has to be that. So not taking the human away.”
Public Participant, Liverpool pg 15 ai@cam and Hopkins Van Mil (2024)
Public dialogue work reveals that AI should enhance rather than replace human capabilities, with strong governance frameworks in place before deployment. Public engagement and transparency are essential, and benefits must be distributed fairly across society.
AI in Healthcare: Public Perspectives
In healthcare discussions, participants saw clear opportunities for AI to support NHS administration and improve service delivery, while expressing caution about AI involvement in direct patient care and diagnosis.
Participants identified several key aspirations for AI in healthcare. A major focus was on reducing the administrative workload that currently burdens healthcare professionals, allowing them to spend more time on direct patient care. There was strong support for AI’s potential in early diagnosis and preventive care, where it could help identify health issues before they become severe. The public also saw significant value in AI accelerating medical research and drug development processes, potentially leading to new treatments more quickly. Finally, participants recognized AI’s capability to help manage complex medical conditions by analyzing large amounts of patient data and identifying optimal treatment strategies. These aspirations reflect a pragmatic view of AI as a tool to enhance healthcare delivery while maintaining the central role of human medical professionals.
Illustrative quotes show the nuanced views.
“My wife [an NHS nurse] says that the paperwork side takes longer than the actual care.”
Public Participant, Liverpool pg 9 ai@cam and Hopkins Van Mil (2024)
“I wouldn’t just want to rely on the technology for something big like that, because obviously it’s a lifechanging situation.”
Public Participant, Cambridge pg 10 ai@cam and Hopkins Van Mil (2024)
Concerns focused particularly on maintaining human involvement in healthcare decisions and protecting patient privacy.
AI in Education: Public Perspectives
In education discussions, participants strongly supported AI’s potential to reduce teacher workload but expressed significant concerns about screen time and the importance of human interaction in learning.
A clear distinction emerged between support for AI in administrative tasks versus direct teaching roles. Participants emphasized that core aspects of education require human qualities that AI cannot replicate.
Key quotes illustrate these views:
“Education isn’t just about learning, it’s about preparing children for life, and you don’t do all of that in front of a screen.”
Public Participant, Cambridge ai@cam and Hopkins Van Mil (2024) pg 18
“Kids with ADHD or autism might prefer to interact with an iPad than they would a person, it could lighten the load for them.”
Public Participant, Liverpool ai@cam and Hopkins Van Mil (2024) pg 17
The dialogue revealed particular concern about the risk of AI increasing screen time and reducing social interaction, while acknowledging potential benefits for personalized learning support.
Dialogue Summary
The public dialogue revealed several important cross-cutting themes about how AI should be deployed in public services. First and foremost was the principle that AI should enhance rather than replace human capabilities - participants consistently emphasized that AI should be a tool to support and augment human work rather than substitute for it. There was also strong consensus that robust governance frameworks need to be established before AI systems are deployed in public services, to ensure proper oversight and accountability.
Transparency and public engagement emerged as essential requirements, with participants emphasizing the need for clear communication about how AI is being used and meaningful opportunities for public input. The fair distribution of benefits was another key concern - participants wanted assurance that AI-enabled improvements would benefit all segments of society rather than exacerbating existing inequalities. Finally, there was strong emphasis on maintaining human-centered service delivery, ensuring that the introduction of AI doesn’t diminish the crucial human elements of public services.
A powerful theme throughout the dialogue was the desire to maintain human connection and expertise while leveraging AI’s capabilities to improve service efficiency and effectiveness. As one participant noted:
“We need to look at the causes, we need to do some more thinking and not just start using AI to plaster over them [societal issues].”
Public Participant, Cambridge pg 13 ai@cam and Hopkins Van Mil (2024)
Bridging Behavioral Science and Data Science
New Flow of Information
Classically the field of statistics focused on mediating the relationship between the machine and the human. Our limited bandwidth of communication means we tend to over-interpret the limited information that we are given, in the extreme we assign motives and desires to inanimate objects (a process known as anthropomorphizing). Much of mathematical statistics was developed to help temper this tendency and understand when we are valid in drawing conclusions from data.
Figure: The trinity of human, data, and computer, and highlights the modern phenomenon. The communication channel between computer and data now has an extremely high bandwidth. The channel between human and computer and the channel between data and human is narrow. New direction of information flow, information is reaching us mediated by the computer. The focus on classical statistics reflected the importance of the direct communication between human and data. The modern challenges of data science emerge when that relationship is being mediated by the machine.
Data science brings new challenges. In particular, there is a very large bandwidth connection between the machine and data. This means that our relationship with data is now commonly being mediated by the machine. Whether this is in the acquisition of new data, which now happens by happenstance rather than with purpose, or the interpretation of that data where we are increasingly relying on machines to summarize what the data contains. This is leading to the emerging field of data science, which must not only deal with the same challenges that mathematical statistics faced in tempering our tendency to over interpret data but must also deal with the possibility that the machine has either inadvertently or maliciously misrepresented the underlying data.
New Productivity Paradox
Thus we face a new productivity paradox. The classical tools of economic intervention cannot map hard-to-measure supply and demand of quality human attention. So how do we build a new economy that utilises our lead in human capital and delivers the digital future we aspire to?
One answer is to look at the human capital index. This measures the quality and quantity of the attention economy via the health and education of our population.
We need to value this and find a way to reinvest human capital, returning the value of the human back into the system when considering productivity gains from technology like AI.
This means a tighter mapping between what the public want and what the innovation economy delivers. It means more agile policy that responds to public dialogue with tangible solutions co-created with the people who are doing the actual work. It means, for example, freeing up a nurse’s time with technology tools and allowing them to spend that time with patients.
To deliver this, our academic institutions need to step up. Too often in the past we have been distant from the difficulties that society faces. We have been too remote from the real challenges of everyday lives — challenges that don’t make the covers of prestige science magazines. People are rightly angry that innovations like AI have yet to address the problems they face, including in health, social care and education.
Of course, universities cannot fix this on their own, but academics can operate as honest brokers that bridge gaps between public and private considerations, convene different groups and understand, celebrate and empower the contributions of individuals.
This requires people who are prepared to dedicate their time to improving each other’s lives, developing new best practices and sharing them with colleagues and coworkers.
To preserve our human capital and harness our potential, we need the AI alchemists to provide us with solutions that can serve both science and society.
The new productivity paradox highlights the challenges of measuring human attention in economic terms and the need for agile policy responses to public needs.
HAM
The Human-Analogue Machine or HAM therefore provides a route through which we could better understand our world through improving the way we interact with machines.
Figure: The trinity of human, data, and computer, and highlights the modern phenomenon. The communication channel between computer and data now has an extremely high bandwidth. The channel between human and computer and the channel between data and human is narrow. New direction of information flow, information is reaching us mediated by the computer. The focus on classical statistics reflected the importance of the direct communication between human and data. The modern challenges of data science emerge when that relationship is being mediated by the machine.
The HAM can provide an interface between the digital computer and the human allowing humans to work closely with computers regardless of their understandin gf the more technical parts of software engineering.
Figure: The HAM now sits between us and the traditional digital computer.
Of course this route provides new routes for manipulation, new ways in which the machine can undermine our autonomy or exploit our cognitive foibles. The major challenge we face is steering between these worlds where we gain the advantage of the computer’s bandwidth without undermining our culture and individual autonomy.
See Lawrence (2024) human-analogue machine (HAMs) p. 343-347, 359-359, 365-368.
Examples of Human-Centered Data Science
Example: Data Science Africa
Data Science Africa is a grass roots initiative that focuses on capacity building to develop ways of solving on the ground problems in health, education, transport and conservation in way that is grounded in local needs and capabilities.
Data Science Africa
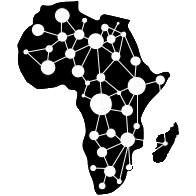
Figure: Data Science Africa https://datascienceafrica.org is a ground up initiative for capacity building around data science, machine learning and artificial intelligence on the African continent.
Figure: Data Science Africa meetings held up to October 2021.
Data Science Africa is a bottom up initiative for capacity building in data science, machine learning and artificial intelligence on the African continent.
As of May 2023 there have been eleven workshops and schools, located in seven different countries: Nyeri, Kenya (twice); Kampala, Uganda; Arusha, Tanzania; Abuja, Nigeria; Addis Ababa, Ethiopia; Accra, Ghana; Kampala, Uganda and Kimberley, South Africa (virtual), and in Kigali, Rwanda.
The main notion is end-to-end data science. For example, going from data collection in the farmer’s field to decision making in the Ministry of Agriculture. Or going from malaria disease counts in health centers to medicine distribution.
The philosophy is laid out in (Lawrence, 2015). The key idea is that the modern information infrastructure presents new solutions to old problems. Modes of development change because less capital investment is required to take advantage of this infrastructure. The philosophy is that local capacity building is the right way to leverage these challenges in addressing data science problems in the African context.
Data Science Africa is now a non-govermental organization registered in Kenya. The organising board of the meeting is entirely made up of scientists and academics based on the African continent.
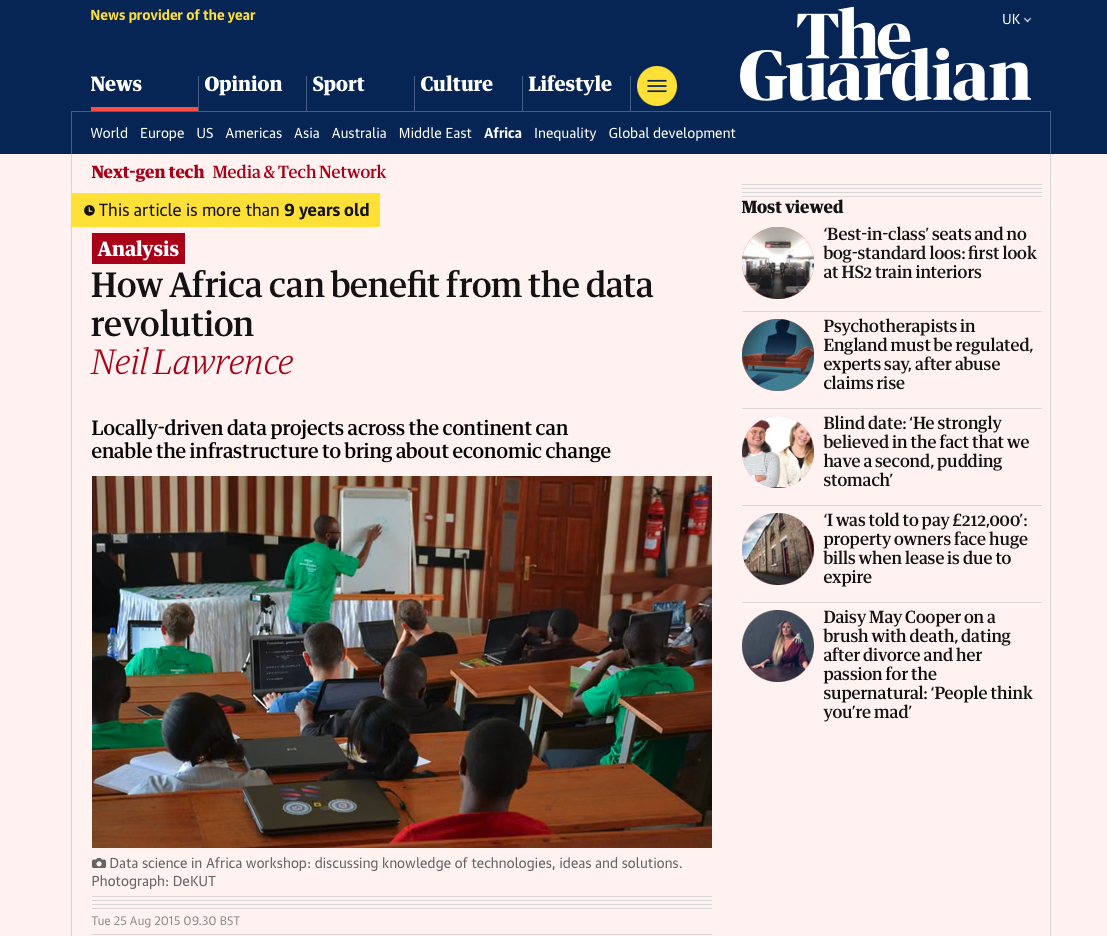
Figure: The lack of existing physical infrastructure on the African continent makes it a particularly interesting environment for deploying solutions based on the information infrastructure. The idea is explored more in this Guardian op-ed on Guardian article on How African can benefit from the data revolution.
Guardian article on Data Science Africa
Data Science Africa is a grassroots initiative that focuses on capacity building to develop ways of solving on-the-ground problems in health, education, transport and conservation in a way that is grounded in local needs and capabilities.
Example: Cambridge Approach
ai@cam is the flagship University mission that seeks to address these challenges. It recognises that development of safe and effective AI-enabled innovations requires this mix of expertise from across research domains, businesses, policy-makers, civil society, and from affected communities. AI@Cam is setting out a vision for AI-enabled innovation that benefits science, citizens and society.
ai@cam
The ai@cam vision is being achieved in a manner that is modelled on other grass roots initiatives like Data Science Africa. Through leveraging the University’s vibrant interdisciplinary research community. ai@cam has formed partnerships between researchers, practitioners, and affected communities that embed equity and inclusion. It is developing new platforms for innovation and knowledge transfer. It is delivering innovative interdisciplinary teaching and learning for students, researchers, and professionals. It is building strong connections between the University and national AI priorities.
We are working across the University to empower the diversity of expertise and capability we have to focus on these broad societal problems. In April 2022 we shared an ai@cam with a vision document that outlines these challenges for the University.
The University operates as both an engine of AI-enabled innovation and steward of those innovations.
AI is not a universal remedy. It is a set of tools, techniques and practices that correctly deployed can be leveraged to deliver societal benefit and mitigate social harm.
The initiative was funded in November 2022 where a £5M investment from the University.
The progress made so far has been across the University community. We have successfully engaged with over members spanning more than 30 departments and institutes, bringing together academics, researchers, start-ups, and large businesses to collaborate on AI initiatives. The program has already supported 6 new funding bids and launched five interdisciplinary A-Ideas projects that bring together diverse expertise to tackle complex challenges. The establishment of the Policy Lab has created a crucial bridge between research and policy-making. Additionally, through the Pioneer program, we have initiated 46 computing projects that are helping to build our technical infrastructure and capabilities.
How ai@cam is Addressing Innovation Challenges
1. Bridging Macro and Micro Levels
Challenge: There is often a disconnect between high-level AI research and real-world needs that must be addressed.
The A-Ideas Initiative represents an effort to bridge this gap by funding interdisciplinary projects that span 19 departments across 6 schools. This ensures diverse perspectives are brought to bear on pressing challenges. Projects focusing on climate change, mental health, and language equity demonstrate how macro-level AI capabilities can be effectively applied to micro-level societal needs.
Challenge: Academic insights often fail to translate into actionable policy changes.
The Policy Lab initiative addresses this by creating direct connections between researchers, policymakers, and the public, ensuring academic insights can influence policy decisions. The Lab produces accessible policy briefs and facilitates public dialogues. A key example is the collaboration with the Bennett Institute and Minderoo Centre, which resulted in comprehensive policy recommendations for AI governance.
2. Addressing Data, Compute, and Capability Gaps
Challenge: Organizations struggle to balance data accessibility with security and privacy concerns.
The data intermediaries initiative establishes trusted entities that represent the interests of data originators, helping to establish secure and ethical frameworks for data sharing and use. Alongside approaches for protecting data we need to improve our approach to processing data. Careful assessment of data quality and organizational data maturity ensures that data can be shared and used effectively. Together these approaches help to ensure that data can be used to serve science, citizens and society.
2. Addressing data, Compute and Capability Gaps
Challenge: Many researchers lack access to necessary computational resources for modern research.
The HPC Pioneer Project addresses this by providing access to the Dawn supercomputer, enabling 46 diverse projects across 20 departments to conduct advanced computational research. This democratization of computing resources ensures that researchers from various disciplines can leverage high-performance computing for their work. The ai@cam project also supports the ICAIN initiative, further strengthening the computational infrastructure available to researchers with a particular focus on emerging economies.
Challenge: There is a significant skills gap in applying AI across different academic disciplines.
The Accelerate Programme for Scientific Discovery addresses this through a comprehensive approach to building AI capabilities. Through a tiered training system that ranges from basic to advanced levels, the programme ensures that domain experts can develop the AI skills relevant to their field. The initiative particularly emphasizes peer-to-peer learning creating sustainable communities of practice where researchers can share knowledge and experiences through “AI Clubs”.
The Accelerate Programme
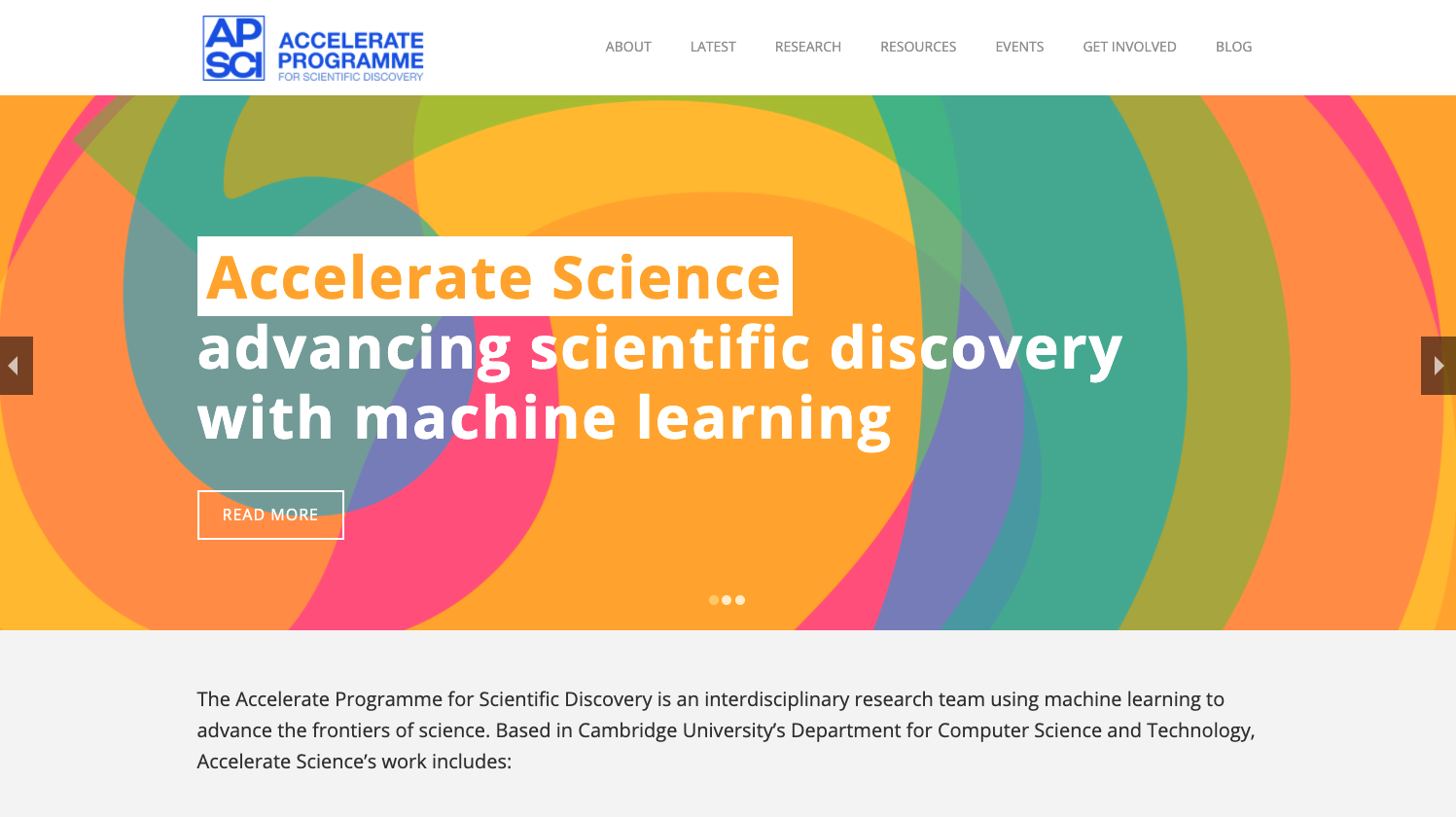
Figure: The Accelerate Programme for Scientific Discovery covers research, education and training, engagement. Our aim is to bring about a step change in scientific discovery through AI. http://science.ai.cam.ac.uk
We’re now in a new phase of the development of computing, with rapid advances in machine learning. But we see some of the same issues – researchers across disciplines hope to make use of machine learning, but need access to skills and tools to do so, while the field machine learning itself will need to develop new methods to tackle some complex, ‘real world’ problems.
It is with these challenges in mind that the Computer Lab has started the Accelerate Programme for Scientific Discovery. This new Programme is seeking to support researchers across the University to develop the skills they need to be able to use machine learning and AI in their research.
To do this, the Programme is developing three areas of activity:
- Research: we’re developing a research agenda that develops and applies cutting edge machine learning methods to scientific challenges, with three Accelerate Research fellows working directly on issues relating to computational biology, psychiatry, and string theory. While we’re concentrating on STEM subjects for now, in the longer term our ambition is to build links with the social sciences and humanities.
Progress so far includes:
Recruited a core research team working on the application of AI in mental health, bioinformatics, healthcare, string theory, and complex systems.
Created a research agenda and roadmap for the development of AI in science.
Funded interdisciplinary projects, e.g. in first round:
Antimicrobial resistance in farming
Quantifying Design Trade-offs in Electricity-generation-focused Tokamaks using AI
Automated preclinical drug discovery in vivo using pose estimation
Causal Methods for Environmental Science Workshop
Automatic tree mapping in Cambridge
Acoustic monitoring for biodiversity conservation
AI, mathematics and string theory
Theoretical, Scientific, and Philosophical Perspectives on Biological Understanding in the age of Artificial Intelligence
AI in pathology: optimising a classifier for digital images of duodenal biopsies
Teaching and learning: building on the teaching activities already delivered through University courses, we’re creating a pipeline of learning opportunities to help PhD students and postdocs better understand how to use data science and machine learning in their work.
Progress so far includes:
Teaching and learning
Brought over 250 participants from over 30 departments through tailored data science and machine learning for science training (Data Science Residency and Machine Learning Academy);
Convened workshops with over 80 researchers across the University on the development of data pipelines for science;
Delivered University courses to over 100 students in Advanced Data Science and Machine Learning and the Physical World.
Online training course in Python and Pandas accessed by over 380 researchers.
Engagement: we hope that Accelerate will help build a community of researchers working across the University at the interface on machine learning and the sciences, helping to share best practice and new methods, and support each other in advancing their research. Over the coming years, we’ll be running a variety of events and activities in support of this.
Progress so far includes:
- Launched a Machine Learning Engineering Clinic that has supported over 40 projects across the University with MLE troubleshooting and advice;
- Hosted and participated in events reaching over 300 people in Cambridge;
- Led international workshops at Dagstuhl and Oberwolfach, convening over 60 leading researchers;
- Engaged over 70 researchers through outreach sessions and workshops with the School of Clinical Medicine, the Faculty of Education, Cambridge Digital Humanities and the School of Biological Sciences.
3. Stakeholder Engagement and Feedback Mechanisms
Challenge: AI development often proceeds without adequate incorporation of public perspectives and concerns.
Our public dialogue work, conducted in collaboration with the Kavli Centre for Ethics, Science, and the Public, creates structured spaces for public dialogue about AI’s potential benefits and risks. The approach ensures that diverse voices and perspectives are heard and considered in AI development.
Challenge: AI initiatives often fail to align with diverse academic needs across institutions.
Cross-University Workshops serve as vital platforms for alignment, bringing together faculty and staff from different departments to discuss AI teaching and learning strategies. By engaging professional services staff, the initiative ensures that capability building extends beyond academic departments to support staff who play key roles in implementing and maintaining AI systems.
4. Flexible and Adaptable Approaches
Challenge: Traditional rigid, top-down research agendas often fail to address real needs effectively.
The AI-deas Challenge Development program empowers researchers to identify and propose challenge areas based on their expertise and understanding of field needs. Through collaborative workshops, these initial ideas are refined and developed, ensuring that research directions emerge organically from the academic community while maintaining alignment with broader strategic goals.
5. Phased Implementation and Realistic Planning
Challenge: Ambitious AI initiatives often fail due to unrealistic implementation timelines and expectations.
The overall strategy emphasizes careful, phased deployment to ensure sustainable success. Beginning with pilot programs like AI-deas and the Policy Lab, the approach allows for testing and refinement of methods before broader implementation. This measured approach enables the incorporation of lessons learned from early phases into subsequent expansions.
6. Independent Oversight and Diverse Perspectives
Challenge: AI initiatives often lack balanced guidance and oversight from diverse perspectives.
The Steering Group provides crucial oversight through representatives from various academic disciplines and professional services. Working with a cross-institutional team, it ensures balanced decision-making that considers multiple perspectives. The group maintains close connections with external initiatives like ELLIS, ICAIN, and Data Science Africa, enabling the university to benefit from and contribute to broader AI developments.
7. Addressing the Innovation Supply Chain
Challenge: Academic innovations often struggle to connect with and address industry needs effectively.
The Industry Engagement initiative develops meaningful industrial partnerships through collaboration with the Strategic Partnerships Office, helping translate research into real-world solutions. The planned sciencepreneurship initiative aims to create a structured pathway from academic research to entrepreneurial ventures, helping ensure that innovations can effectively reach and benefit society.
ai@cam is a flagship University mission that seeks to address innovation challenges. It recognizes that development of safe and effective AI-enabled innovations requires expertise from across research domains, businesses, policy-makers, civil society, and from affected communities.
Conclusion: The Innovation Economy
Innovation Economy Conclusion
ai@cam’s approach aims to address the macro-micro disconnects in AI innovation through several key strategies. We are building bridges between macro and micro levels, fostering interdisciplinary collaboration, engaging diverse stakeholders and voices, and providing crucial resources and training. Through these efforts, ai@cam is working to create a more integrated and effective AI innovation ecosystem.
Our implementation approach emphasizes several critical elements learned from past IT implementation failures. We focus on flexibility to adapt to changing needs, phased rollout of initiatives to manage risk, establishing continuous feedback loops for improvement, and maintaining a learning mindset throughout the process.
Looking to the future, we recognize that AI technologies and their applications will continue to evolve rapidly. This evolution requires strategic agility and a continued focus on effective implementation. We will need to remain adaptable, continuously assessing and adjusting our strategies while working to bridge capability gaps between high-level AI capabilities and on-the-ground implementation challenges.
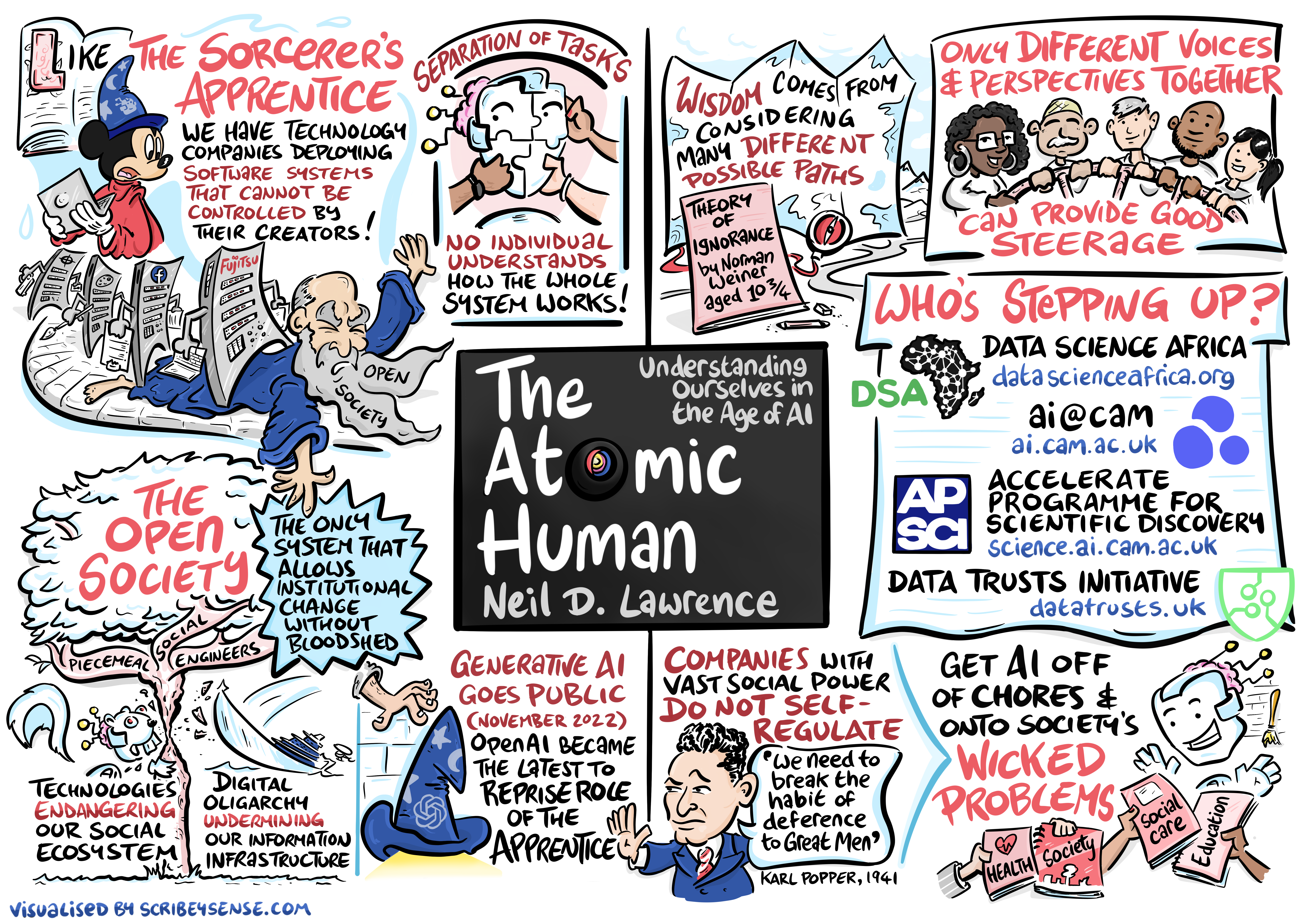
Figure: This is the drawing Dan was inspired to create for the Epilogue, it captures the challenges society faces and summarises some of the initiatives that are looking to address them.
See blog post on Who is Stepping Up?.
Thanks!
For more information on these subjects and more you might want to check the following resources.
- book: The Atomic Human
- twitter: @lawrennd
- podcast: The Talking Machines
- newspaper: Guardian Profile Page
- blog: http://inverseprobability.com